/ Introduction
/ Introduction
/ Introduction
Recommendation enables media applications to support users in discovering additional media content (e.g., news articles, videos) and to keep consumers engaged. The main challenge in this context is that the recommendation approaches has little potential for the discovery of new types of content for the consumer and they might cause the popular media content becoming even more popular. Such problems can ultimately lead to filter bubbles, echo chambers or group-think conditions. WP2 will tackle these undesired phenomena which are likely originated from the current personalization and recommendation approaches.
This will be done by computing responsible (predictive) models for a fair recommendation that will enhance user engagement through novel mechanisms by (i) providing explanations of recommendations to users (transparency), (ii) expanding recommendations to cover a rich spectrum of media content (diversity), (iii) ensuring the niche or minority content is suggested to users (fairness).
The outcome will be novel recommendation algorithms taking into account multiple competing objectives (e.g., relevance vs. information balance). In doing so, WP2 will address the following main research questions: To what extent can we effectively and fairly model online user behavior and predict this behavior? To what extent can we personalize and engage users online to efficiently keep them informed, and at the same time do this responsibly?
Objective: To develop user modeling and personalisation techniques capable of effectively eliciting user preferences in order to enchance the user experience when interacting with media content while taking into account important competing factors (e.g., business values, societal values, individual values).
Recommendation enables media applications to support users in discovering additional media content (e.g., news articles, videos) and to keep consumers engaged. The main challenge in this context is that the recommendation approaches has little potential for the discovery of new types of content for the consumer and they might cause the popular media content becoming even more popular. Such problems can ultimately lead to filter bubbles, echo chambers or group-think conditions. WP2 will tackle these undesired phenomena which are likely originated from the current personalization and recommendation approaches.
This will be done by computing responsible (predictive) models for a fair recommendation that will enhance user engagement through novel mechanisms by (i) providing explanations of recommendations to users (transparency), (ii) expanding recommendations to cover a rich spectrum of media content (diversity), (iii) ensuring the niche or minority content is suggested to users (fairness). The outcome will be novel recommendation algorithms taking into account multiple competing objectives (e.g., relevance vs. information balance). In doing so, WP2 will address the following main research questions: To what extent can we effectively and fairly model online user behavior and predict this behavior? To what extent can we personalize and engage users online to efficiently keep them informed, and at the same time do this responsibly?
Objective: To develop user modeling and personalisation techniques capable of effectively eliciting user preferences in order to enchance the user experience when interacting with media content while taking into account important competing factors (e.g., business values, societal values, individual values).
Recommendation enables media applications to support users in discovering additional media content (e.g., news articles, videos) and to keep consumers engaged. The main challenge in this context is that the recommendation approaches has little potential for the discovery of new types of content for the consumer and they might cause the popular media content becoming even more popular. Such problems can ultimately lead to filter bubbles, echo chambers or group-think conditions. WP2 will tackle these undesired phenomena which are likely originated from the current personalization and recommendation approaches.
This will be done by computing responsible (predictive) models for a fair recommendation that will enhance user engagement through novel mechanisms by (i) providing explanations of recommendations to users (transparency), (ii) expanding recommendations to cover a rich spectrum of media content (diversity), (iii) ensuring the niche or minority content is suggested to users (fairness). The outcome will be novel recommendation algorithms taking into account multiple competing objectives (e.g., relevance vs. information balance). In doing so, WP2 will address the following main research questions: To what extent can we effectively and fairly model online user behavior and predict this behavior? To what extent can we personalize and engage users online to efficiently keep them informed, and at the same time do this responsibly?
Objective: To develop user modeling and personalisation techniques capable of effectively eliciting user preferences in order to enchance the user experience when interacting with media content while taking into account important competing factors (e.g., business values, societal values, individual values).
/ People
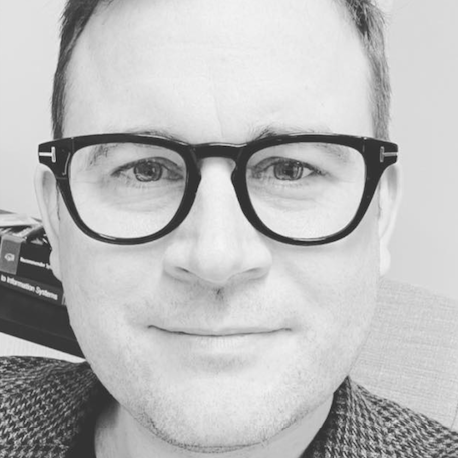
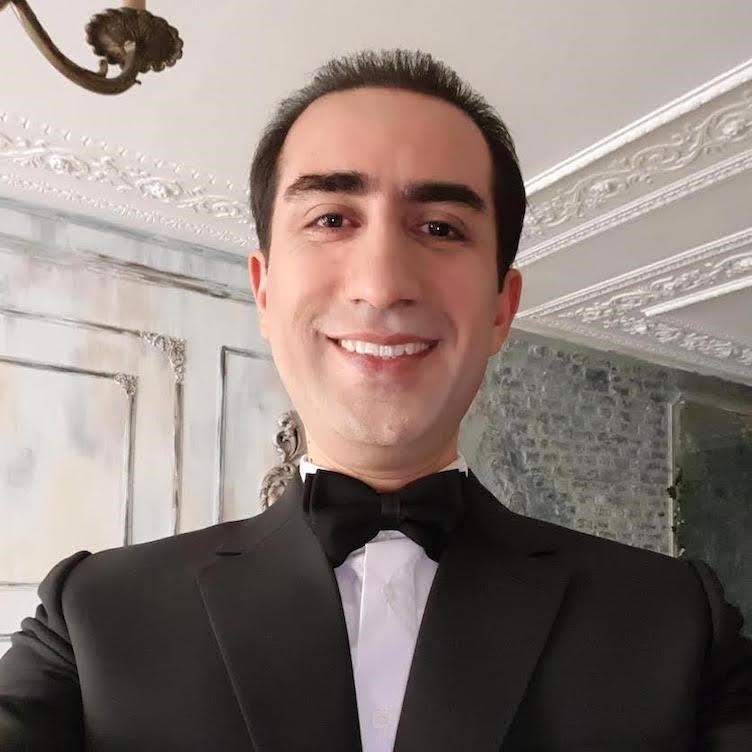
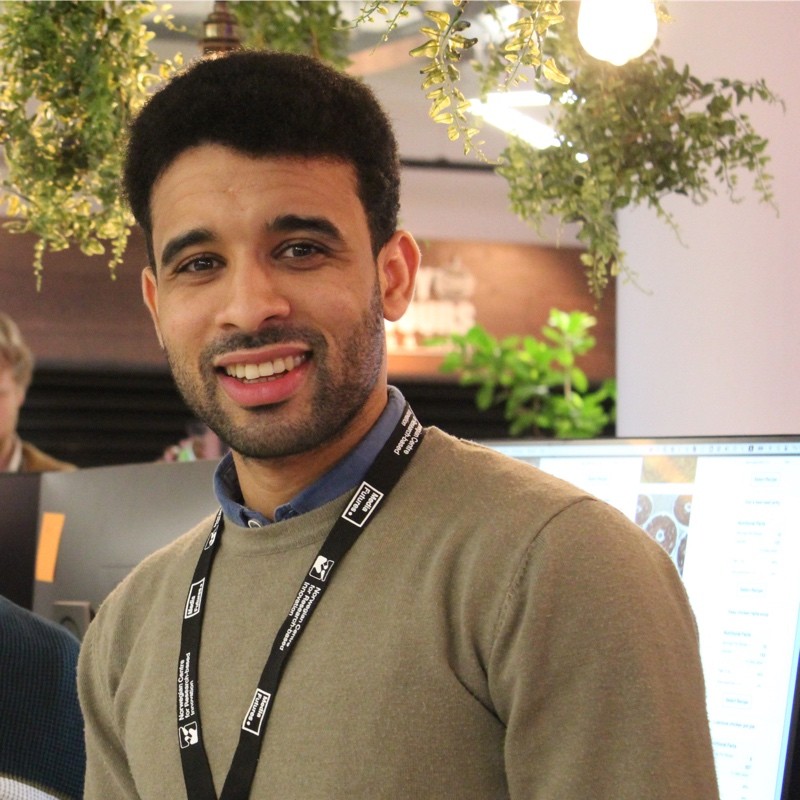
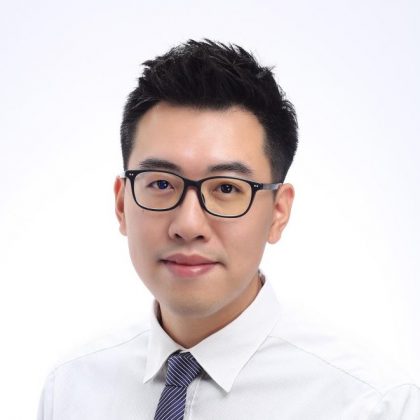
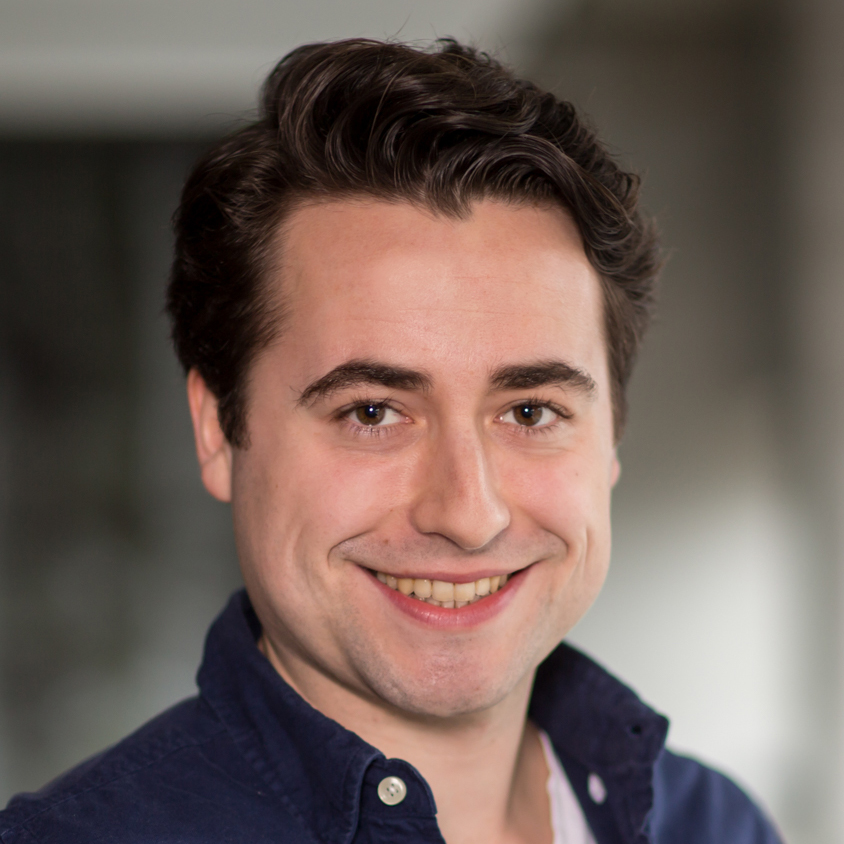
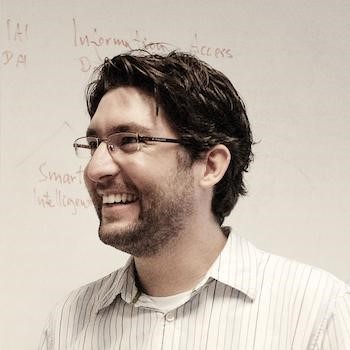
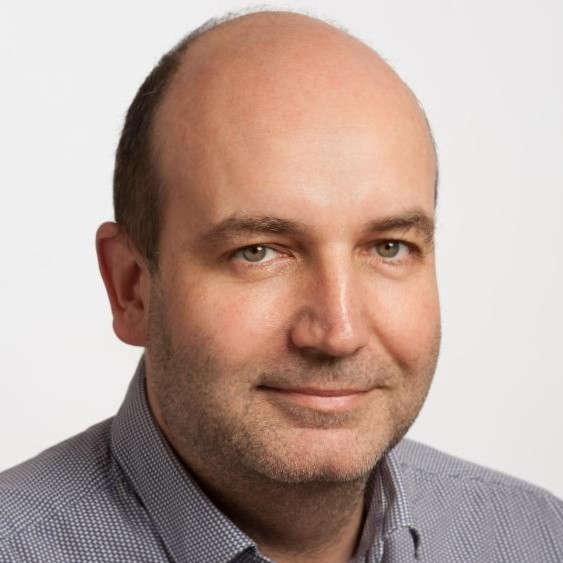
Dietmar Jannach
Work Package Advisor & Key Researcher
Universität Klagenfurt
Read more
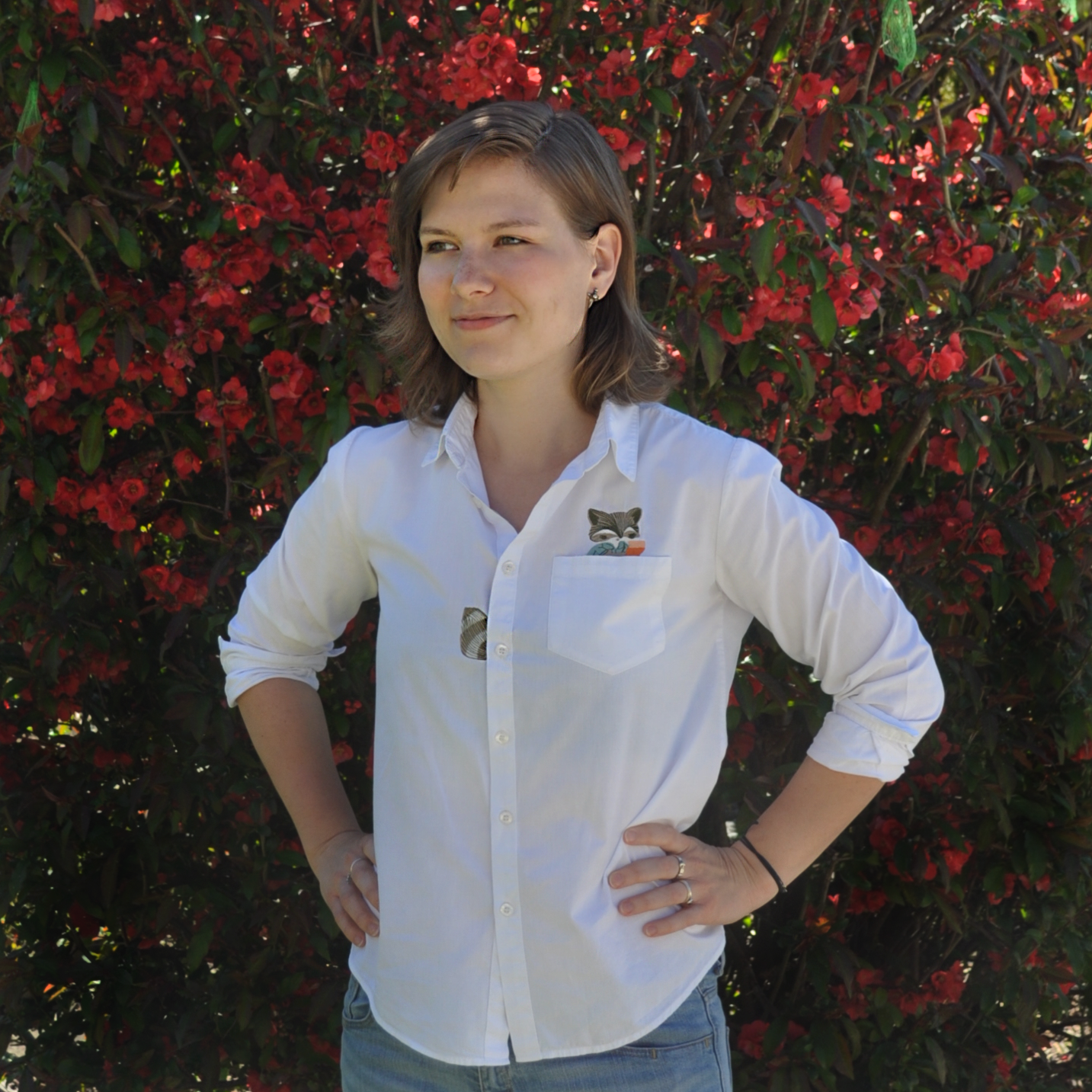
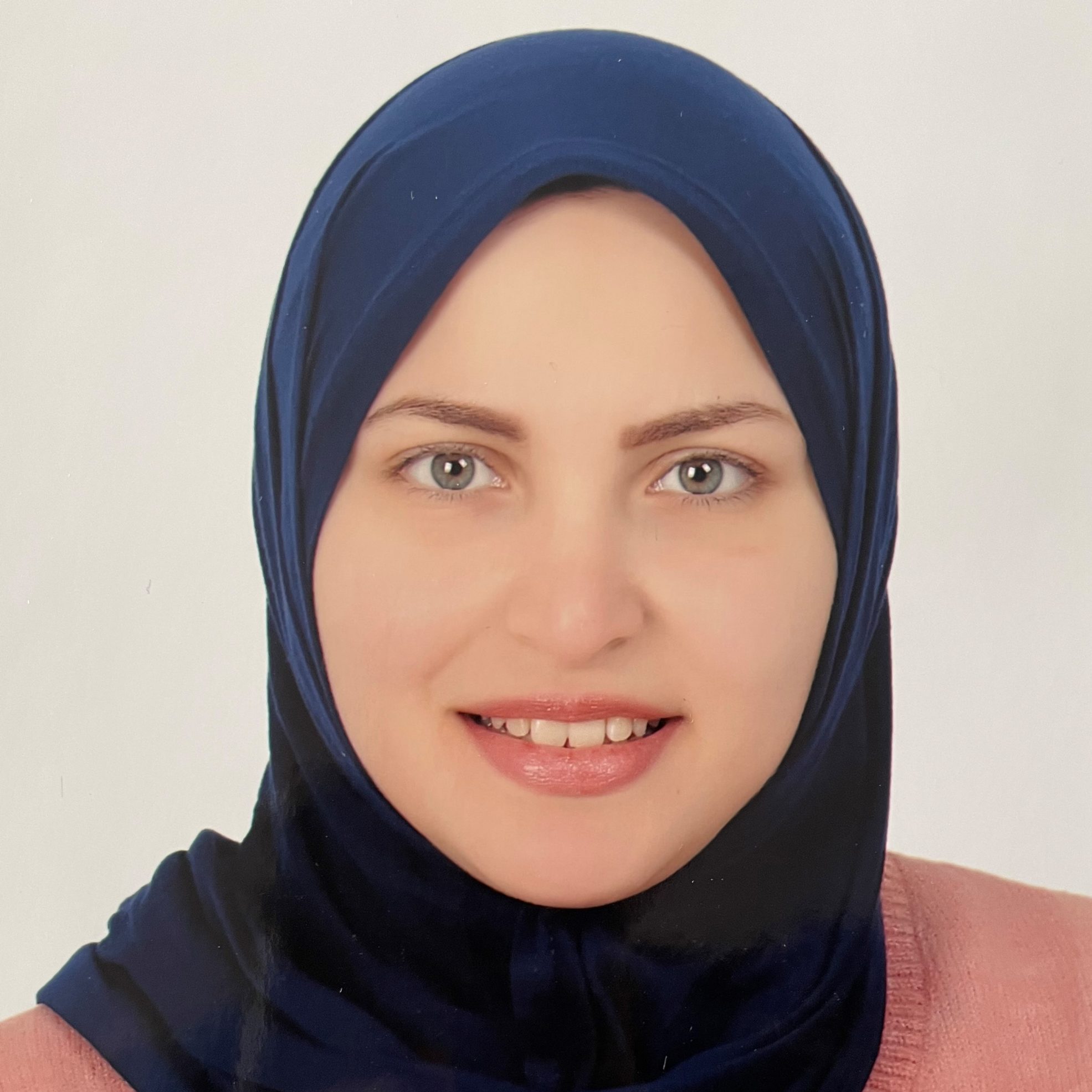
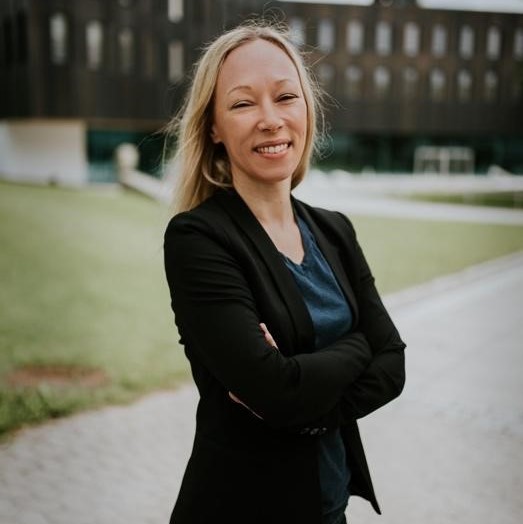
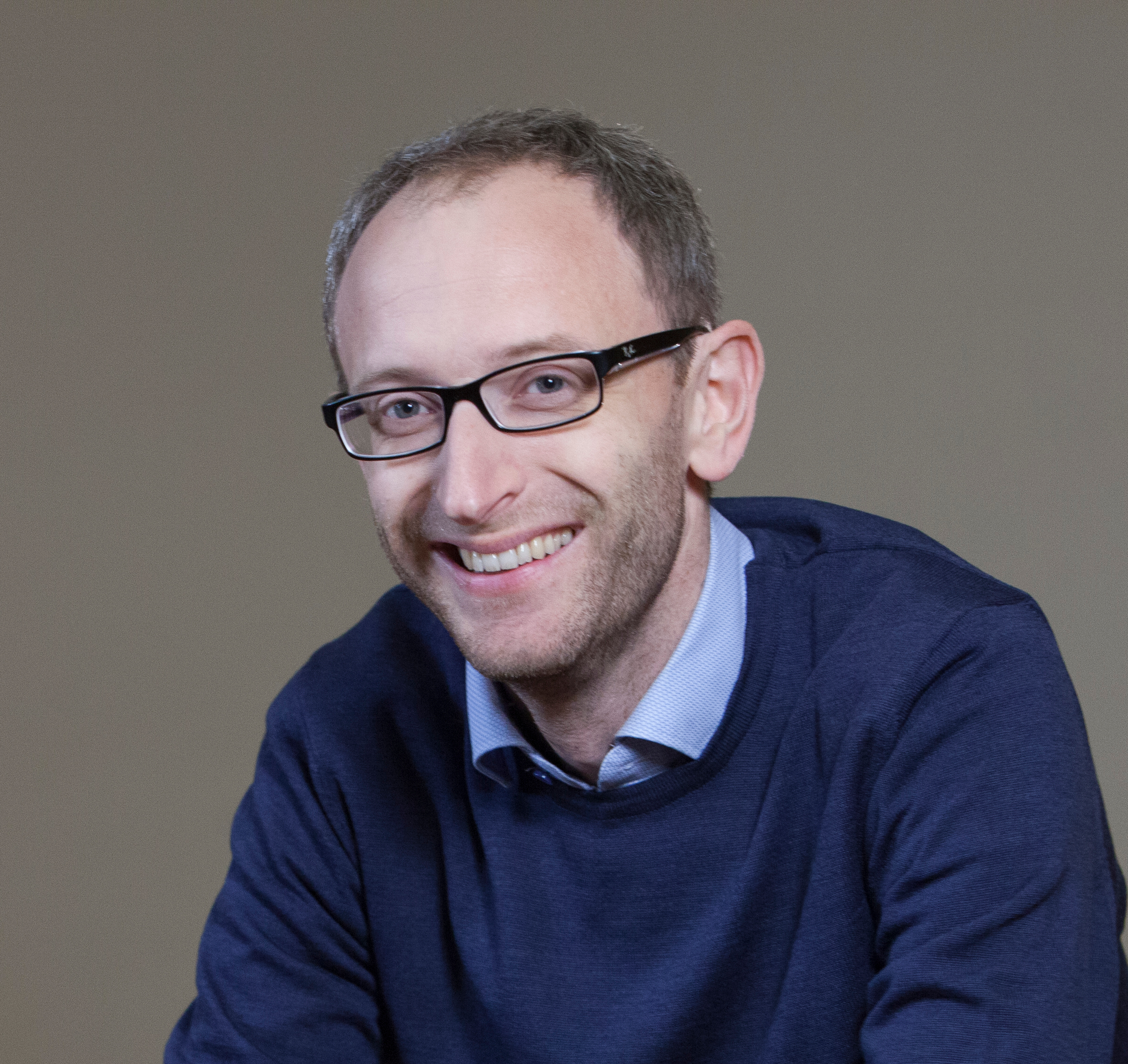
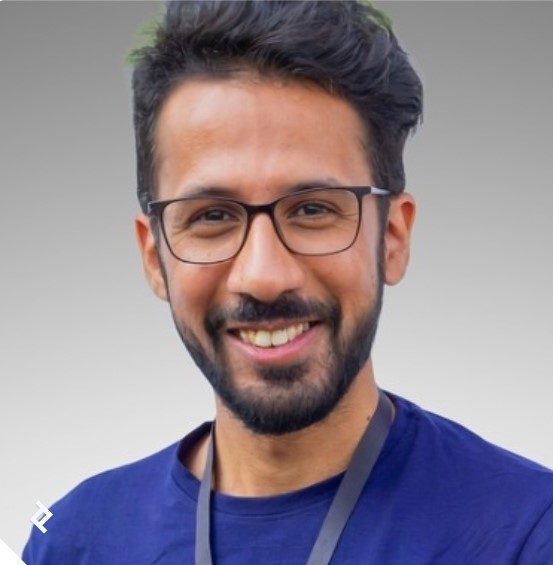
Igor Pipkin
Researcher
Amedia
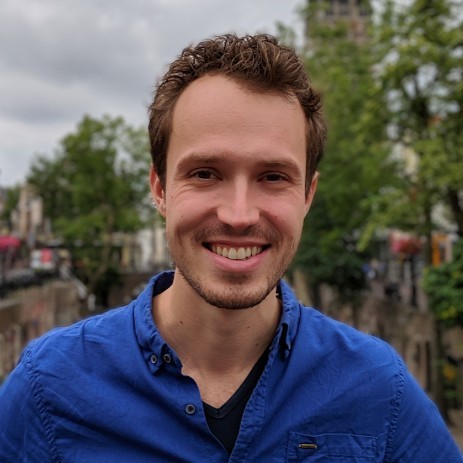
Thomas Husken
Researcher
BT
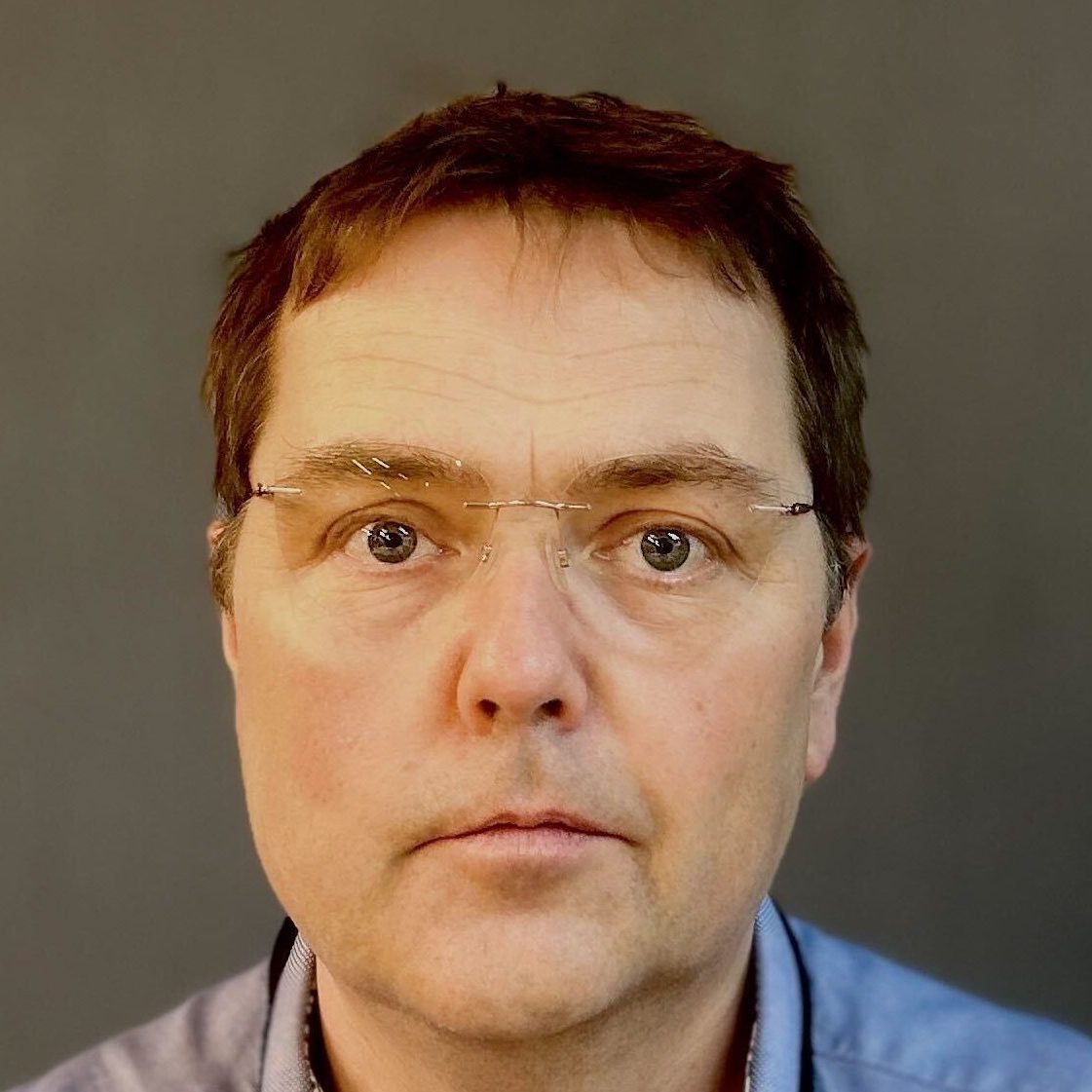
Eivind Throndsen
Schibsted
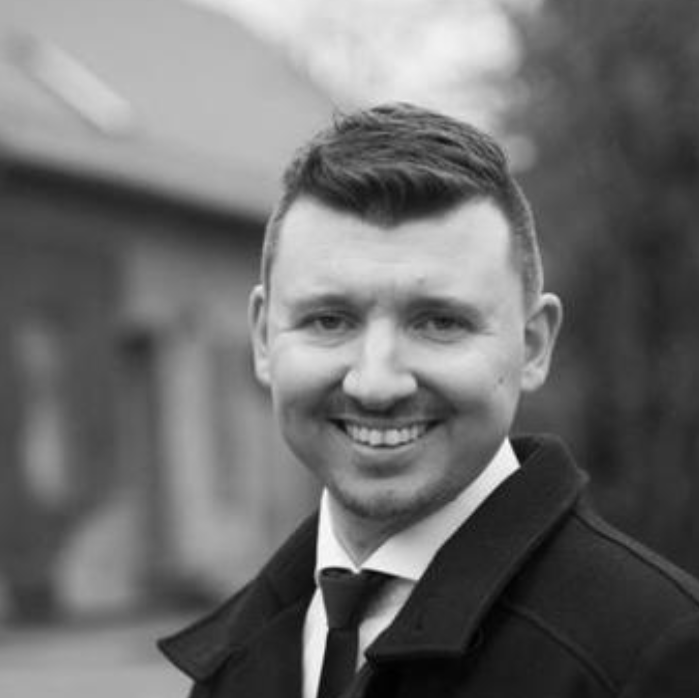
Erik Knudsen
Researcher
University of Bergen
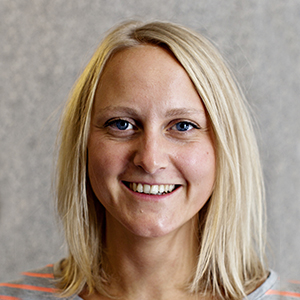
Eva Hagan
NRK
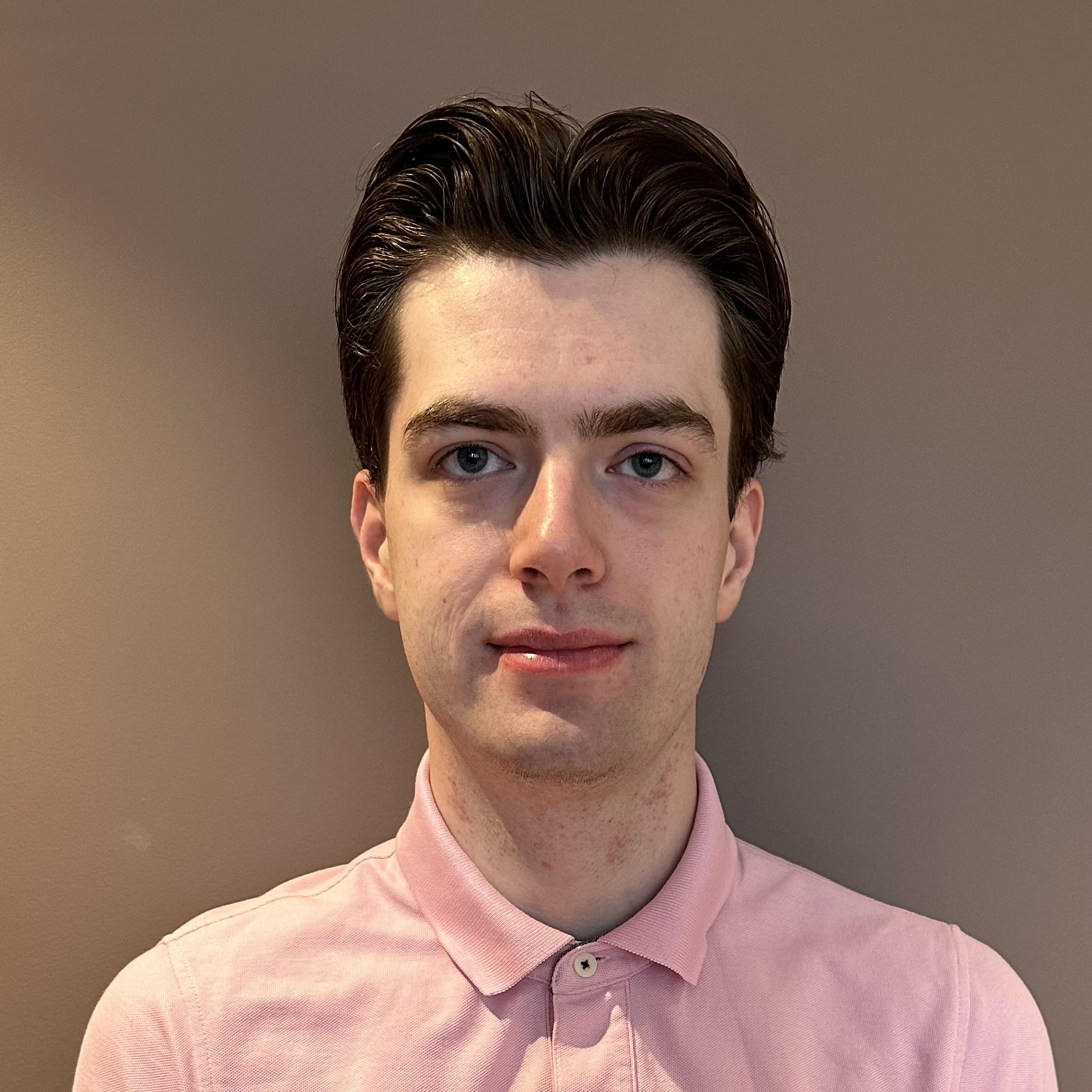
Anders Sandvik Bremnes
Research Assistant
University in Bergen
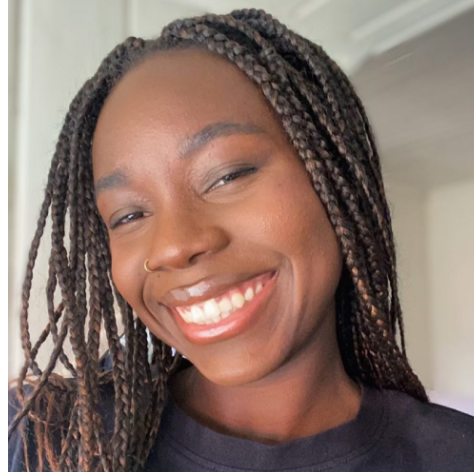
Gloria Kasangu
Research Assistant
University of Bergen
/ Publications
2024
Daniel Rosnes; Alain Starke; Christoph Trattner
Shaping the Future of Content-based News Recommenders: Insights from Evaluating Feature-Specific Similarity Metrics Conference
ACM UMAP '24, 2024.
@conference{umap2024Daniel,
title = {Shaping the Future of Content-based News Recommenders: Insights from Evaluating Feature-Specific Similarity Metrics},
author = {Daniel Rosnes and Alain Starke and Christoph Trattner },
url = {https://mediafutures.no/umap2024/},
year = {2024},
date = {2024-07-01},
booktitle = {ACM UMAP '24},
abstract = {In news media, recommender system technology faces several domain-specific challenges. The continuous stream of new content and users deems content-based recommendation strategies, based on similar-item retrieval, to remain popular. However, a persistent challenge is to select relevant features and corresponding similarity functions, and whether this depends on the specific context. We evaluated feature-specific similarity metrics using human similarity judgments across national and local news domains. We performed an online experiment ($N = 141$) where we asked participants to judge the similarity between pairs of randomly sampled news articles. We had three contributions: (1) comparing novel metrics based on large language models to ones traditionally used in news recommendations, (2) exploring differences in similarity judgments across national and local news domains, and (3) examining which content-based strategies were perceived as appropriate in the news domain. Our results showed that one of the novel large language model based metrics (SBERT) was highly correlated with human judgments, while there were only small, most non-significant differences across national and local news domains. Finally, we found that while it may be possible to automatically recommend similar news using feature-specific metrics, their representativeness and appropriateness varied. We explain how our findings can guide the design of future content-based and hybrid recommender strategies in the news domain.},
keywords = {},
pubstate = {published},
tppubtype = {conference}
}
Jia Hua Jeng; Gloria Anne Babile Kasangu; Alain D. Starke; Christoph Trattner
Emotional Reframing of Economic News using a Large Language Model Conference
ACM UMAP 2024, 2024.
@conference{emorefram24,
title = {Emotional Reframing of Economic News using a Large Language Model},
author = {Jia Hua Jeng and Gloria Anne Babile Kasangu and Alain D. Starke and Christoph Trattner},
url = {https://mediafutures.no/umap2024___jeng_alain_gloria_christoph__workshop_-3/},
year = {2024},
date = {2024-07-01},
urldate = {2024-07-01},
booktitle = {ACM UMAP 2024},
abstract = {News media framing can shape public perception and potentially polarize views. Emotional language can exacerbate these framing effects, as a user’s emotional state can be an important contextual factor to use in news recommendation. Our research explores the relation between emotional framing techniques and the emotional states of readers, as well as readers’ perceived trust in specific news articles. Users (N = 200) had to read three economic news articles from the Washington Post. We used ChatGPT-4 to reframe news articles with specific emotional languages (Anger, Fear, Hope), compared to a neutral baseline reframed by a human journalist. Our results revealed that negative framing (Anger, Fear) elicited stronger negative emotional states among users than the neutral baseline, while Hope led to little changes overall. In contrast, perceived trust levels varied little across the different conditions. We discuss the implications of our findings and how emotional framing could affect societal polarization issues},
keywords = {},
pubstate = {published},
tppubtype = {conference}
}
Alain D. Starke; Anders Sandvik Bremnes; Erik Knudsen; Damian Trilling; Christoph Trattner
Perception versus Reality: Evaluating User Awareness of Political Selective Exposure in News Recommender Systems Conference
ACM UMAP 2024, 2024.
@conference{percepvsreal24,
title = {Perception versus Reality: Evaluating User Awareness of Political Selective Exposure in News Recommender Systems},
author = {Alain D. Starke and Anders Sandvik Bremnes and Erik Knudsen and Damian Trilling and Christoph Trattner},
url = {https://mediafutures.no/umap2024___erik_alain_damian_anders_christoph/},
year = {2024},
date = {2024-07-01},
urldate = {2024-07-01},
booktitle = {ACM UMAP 2024},
abstract = {News Recommender Systems (NRSs) have become increasingly pivotal in shaping the news landscape, particularly in how news is disseminated. This has also led to concerns about information diversity, especially regarding selective exposure in the realm of political news. Users may not recognize that news content presented to them is subject to selective exposure, through users that incorporate political beliefs. Within the U.S. two-party system, our research explores the interactions between NRSs and users’ ability to discern news articles that align with their political biases. We performed an online experiment (N = 160) to address the issue of user awareness and self-recognition of selective exposure within NRSs. Users were asked to select any number of news articles that matched their political orientation (i.e., Democrat or Republican) from a list of 50 news articles (5 Democrat, 5 Republican, 40 filler articles), which were either ranked saliently towards their political orientation or randomly. Contrary to expectations, our findings reveal no significant difference in article selection between participants exposed to a baseline random order and those who where presented with the more salient and easy to select version. We did observe that Republicans performed worse than Democrats in identifying aligning articles, based on precision and recall metrics.},
keywords = {},
pubstate = {published},
tppubtype = {conference}
}
Bilal Mahmood; Mehdi Elahi; Samia Touileb; Lubos Steskal; Christoph Trattner
Incorporating Editorial Feedback in the Evaluation of News Recommender Systems Conference
ACM UMAP 2024, 2024.
@conference{incoed24,
title = {Incorporating Editorial Feedback in the Evaluation of News Recommender Systems},
author = {Bilal Mahmood and Mehdi Elahi and Samia Touileb and Lubos Steskal and Christoph Trattner},
url = {https://mediafutures.no/lbr_umap_editorial_component_in_nrs/},
year = {2024},
date = {2024-07-01},
urldate = {2024-07-01},
booktitle = {ACM UMAP 2024},
abstract = {Research in the recommender systems field typically applies a rather traditional evaluation methodology when assessing the quality of recommendations. This methodology heavily relies on incorporating different forms of user feedback (e.g., clicks) representing the specific needs and interests of the users. While this methodology may offer various benefits, it may fail to comprehensively project the complexities of certain application domains, such as the news domain. This domain is distinct from other domains primarily due to the strong influence of editorial control in the news delivery process. Incorporation of this role can profoundly impact how the relevance of news articles is measured when recommended to the users. Despite its critical importance, there appears to be a research gap in investigating the dynamics between the roles of editorial control and personalization in the community of recommender systems. In this paper, we address this gap by conducting experiments where the relevance of recommendations is assessed from an editorial perspective. We received a real-world dataset from TV 2, one of the largest editor-managed commercial media houses in Norway, which includes editors’ feedback on how news articles are being related. In our experiment, we considered a scenario where algorithm-generated recommendations, using the K-Nearest Neighbor (KNN) model, employing various text embedding models to encode different sections of the news articles (e.g., title, lead title, body text, and full text), are compared against the editorial feedback. The results are promising, demonstrating the effectiveness of the recommendation in fulfilling the editorial prospects.},
keywords = {},
pubstate = {published},
tppubtype = {conference}
}
Anastasiia Klimashevskaia; Dietmar Jannach; Mehdi Elahi; Christoph Trattner
A Survey on Popularity Bias in Recommender Systems Journal Article Forthcoming
In: User Modeling and User-Adapted Interaction (UMUAI), Forthcoming.
@article{biasanas24,
title = {A Survey on Popularity Bias in Recommender Systems},
author = {Anastasiia Klimashevskaia and Dietmar Jannach and Mehdi Elahi and Christoph Trattner},
url = {https://mediafutures.no/popularitybias_literature_review-5/},
year = {2024},
date = {2024-06-13},
journal = {User Modeling and User-Adapted Interaction (UMUAI)},
abstract = {Recommender systems help people find relevant content in a personalized way. One main promise of such systems is that they are able to increase the visibility of items in the long tail, i.e., the lesser-
known items in a catalogue. Existing research, however, suggests that in many situations today’s recommendation algorithms instead exhibit a popularity bias, meaning that they often focus on rather popular items in their recommendations. Such a bias may not only lead to the limited value of the recommendations for consumers and providers in the short run, but it may also cause undesired reinforcement effects over time. In this paper, we discuss the potential reasons for popularity bias and review existing approaches to detect, quantify and mitigate popularity bias in
recommender systems. Our survey, therefore, includes both an overview
of the computational metrics used in the literature as well as a review of the main technical approaches to reduce the bias. Furthermore, we critically discuss today’s literature, where we observe that the research is almost entirely based on computational experiments and on certain
assumptions regarding the practical effects of including long-tail items in the recommendations.},
keywords = {},
pubstate = {forthcoming},
tppubtype = {article}
}
known items in a catalogue. Existing research, however, suggests that in many situations today’s recommendation algorithms instead exhibit a popularity bias, meaning that they often focus on rather popular items in their recommendations. Such a bias may not only lead to the limited value of the recommendations for consumers and providers in the short run, but it may also cause undesired reinforcement effects over time. In this paper, we discuss the potential reasons for popularity bias and review existing approaches to detect, quantify and mitigate popularity bias in
recommender systems. Our survey, therefore, includes both an overview
of the computational metrics used in the literature as well as a review of the main technical approaches to reduce the bias. Furthermore, we critically discuss today’s literature, where we observe that the research is almost entirely based on computational experiments and on certain
assumptions regarding the practical effects of including long-tail items in the recommendations.
Alain D. Starke; Martijn C. Willemsen
Psychologically Informed Design of Energy Recommender Systems: Are Nudges Still Effective in Tailored Choice Environments? Book Chapter
In: pp. 221–259, A Human-Centered Perspective of Intelligent Personalized Environments and Systems, 2024.
BibTeX | Links:
@inbook{Alain_pysch24,
title = {Psychologically Informed Design of Energy Recommender Systems: Are Nudges Still Effective in Tailored Choice Environments?},
author = {Alain D. Starke and Martijn C. Willemsen},
url = {https://mediafutures.no/starke2024-book-chapter-psych-informed-energy-recsys-4/},
year = {2024},
date = {2024-05-01},
urldate = {2024-05-01},
pages = {221–259},
edition = {A Human-Centered Perspective of Intelligent Personalized Environments and Systems},
series = {Human–Computer Interaction Series},
keywords = {},
pubstate = {published},
tppubtype = {inbook}
}
Maria Soledad Pera; Federica Cena; Monica Landoni; Cataldo Musto; Alain D. Starke
Human Factors in User Modeling for Intelligent Systems Book Chapter
In: pp. 3–42, A Human-Centered Perspective of Intelligent Personalized Environments and Systems, 2024.
@inbook{Alain_humanF24,
title = {Human Factors in User Modeling for Intelligent Systems},
author = {Maria Soledad Pera and Federica Cena and Monica Landoni and Cataldo Musto and Alain D. Starke},
url = {https://mediafutures.no/pera2024-book-chapter-holistic-user-modeling/},
year = {2024},
date = {2024-05-01},
urldate = {2024-05-01},
pages = {3–42},
edition = {A Human-Centered Perspective of Intelligent Personalized Environments and Systems},
series = {Human–Computer Interaction Series.},
abstract = {In the current digital landscape, humans take center stage. This has caused a paradigm shift in the realm of intelligent technologies, prompting researchers and (industry) practitioners to reflect on the challenges and complexities involved in understanding the (potential) users of the technologies they develop. In this chapter, we provide an overview of human factors in user modeling, a core component of human-centered intelligent solutions. We discuss critical concepts, dimensions, and theories that inform the design of user models that are more attuned to human characteristics. Additionally, we emphasize the need for a comprehensive user model that simultaneously considers multiple factors to represent the intricacies of individuals’ interests and behaviors. Such a holistic model can, in turn, shape the design of intelligent solutions that are better able to adapt and cater to a wide range of user groups.},
keywords = {},
pubstate = {published},
tppubtype = {inbook}
}
2023
Erik Knudsen; Alain D. Starke; Christoph Trattner
Topical Preference Trumps Other Features in News Recommendation: A Conjoint Analysis on a Representative Sample from Norway Conference
Association for Computing Machinery (ACM) RecSys ’23, 2023.
BibTeX | Links:
@conference{inra2023-1,
title = {Topical Preference Trumps Other Features in News Recommendation: A Conjoint Analysis on a Representative Sample from Norway},
author = {Erik Knudsen and Alain D. Starke and Christoph Trattner },
url = {https://mediafutures.no/inra2023-1/},
year = {2023},
date = {2023-09-18},
urldate = {2023-09-18},
booktitle = {Association for Computing Machinery (ACM) RecSys ’23},
keywords = {},
pubstate = {published},
tppubtype = {conference}
}
Khadiga Seddik; Erik Knudsen; Damian Trilling; Christoph Trattner
Understanding How News Recommender Systems Influence Selective Exposure Conference
Association for Computing Machinery (ACM) RecSys ’23, 2023.
BibTeX | Links:
@conference{behavrec2023,
title = {Understanding How News Recommender Systems Influence Selective Exposure},
author = {Khadiga Seddik and Erik Knudsen and Damian Trilling and Christoph Trattner },
url = {https://mediafutures.no/behavrec2023/},
year = {2023},
date = {2023-09-18},
urldate = {2023-09-18},
booktitle = {Association for Computing Machinery (ACM) RecSys ’23},
keywords = {},
pubstate = {published},
tppubtype = {conference}
}
Anastasiia Klimashevskaia; Mehdi Elahi; Dietmar Jannach; Lars Skjærven; Astrid Tessem; Christoph Trattner
Evaluating The Effects of Calibrated Popularity Bias Mitigation: A Field Study Conference
Association for Computing Machinery (ACM) RecSys ’23, 2023.
BibTeX | Links:
@conference{RecSys_2023_LBR,
title = {Evaluating The Effects of Calibrated Popularity Bias Mitigation: A Field Study},
author = {Anastasiia Klimashevskaia and Mehdi Elahi and Dietmar Jannach and Lars Skjærven and Astrid Tessem and Christoph Trattner},
url = {https://mediafutures.no/recsys_2023_lbr/},
year = {2023},
date = {2023-09-18},
booktitle = {Association for Computing Machinery (ACM) RecSys ’23},
keywords = {},
pubstate = {published},
tppubtype = {conference}
}
Ayoub El Majjodi; Alain D. Starke; Christoph Trattner
The Interplay between Food Knowledge, Nudges, and Preference Elicitation Methods Determines the Evaluation of a Recipe Recommender System Conference
Association for Computing Machinery (ACM) RecSys ’23, 2023.
BibTeX | Links:
@conference{inra2023,
title = {The Interplay between Food Knowledge, Nudges, and Preference Elicitation Methods Determines the Evaluation of a Recipe Recommender System},
author = {Ayoub El Majjodi and Alain D. Starke and Christoph Trattner },
url = {https://mediafutures.no/intrs2023-2/},
year = {2023},
date = {2023-09-18},
urldate = {2023-09-18},
booktitle = {Association for Computing Machinery (ACM) RecSys ’23},
keywords = {},
pubstate = {published},
tppubtype = {conference}
}
Alain Starke; Kimia Emami; Andrea Makarová; Bruce Ferwerda
Using Visual and Linguistic Framing to Support Sustainable Decisions in an Online Store Conference
Association for Computing Machinery (ACM) RecSys ’23,, 2023.
BibTeX | Links:
@conference{intrs23_session3,
title = {Using Visual and Linguistic Framing to Support Sustainable Decisions in an Online Store},
author = {Alain Starke and Kimia Emami and Andrea Makarová and Bruce Ferwerda },
url = {https://mediafutures.no/intrs23_session3_paper_2/},
year = {2023},
date = {2023-09-18},
urldate = {2023-09-18},
booktitle = {Association for Computing Machinery (ACM) RecSys ’23,},
keywords = {},
pubstate = {published},
tppubtype = {conference}
}
Jia Hua Jeng; Alain D. Starke; Christoph Trattner
Towards Attitudinal Change in News Recommender Systems: A Pilot Study on Climate Change Workshop
2023.
@workshop{Jeng2023,
title = {Towards Attitudinal Change in News Recommender Systems: A Pilot Study on Climate Change},
author = {Jia Hua Jeng and Alain D. Starke and Christoph Trattner},
url = {https://mediafutures.no/jeng2023-towards-attitudinal-change-in-news2908-2/},
year = {2023},
date = {2023-04-18},
urldate = {2023-04-18},
abstract = {Personalized recommender systems facilitate decision-making in various domains by presenting content closely aligned with users’ preferences.
However, personalization can lead to unintended consequences. In news, selective information exposure and consumption might amplify
polarization, as users are empowered to seek out information that is in line with their own attitudes and viewpoints. However, personalization in
terms of algorithmic content and persuasive technology could also help to narrow the gap between polarized user attitudes and news consumption
patterns. This paper presents a pilot study on climate change news. We examined the relation between users’ level of environmental concern, their preferences
for news articles, and news article content. We aimed to capture a news article’s viewpoint through sentiment analysis. Users (N = 180)
were asked to read and evaluate 10 news articles from the Washington Post. We found a positive correlation between users’ level of environmental
concern and whether they liked the article. In contrast, no significant correlation was found between sentiment and environmental concern.
We argue why a different type of news article analysis than sentiment is needed. Finally, we present our research agenda on how persuasive technology
might help to support more exploration of news article viewpoints in the future.},
keywords = {},
pubstate = {published},
tppubtype = {workshop}
}
However, personalization can lead to unintended consequences. In news, selective information exposure and consumption might amplify
polarization, as users are empowered to seek out information that is in line with their own attitudes and viewpoints. However, personalization in
terms of algorithmic content and persuasive technology could also help to narrow the gap between polarized user attitudes and news consumption
patterns. This paper presents a pilot study on climate change news. We examined the relation between users’ level of environmental concern, their preferences
for news articles, and news article content. We aimed to capture a news article’s viewpoint through sentiment analysis. Users (N = 180)
were asked to read and evaluate 10 news articles from the Washington Post. We found a positive correlation between users’ level of environmental
concern and whether they liked the article. In contrast, no significant correlation was found between sentiment and environmental concern.
We argue why a different type of news article analysis than sentiment is needed. Finally, we present our research agenda on how persuasive technology
might help to support more exploration of news article viewpoints in the future.
2022
Ayoub El Majjodi; Alain D. Starke; Christoph Trattner
Nudging Towards Health? Examining the Merits of Nutrition Labels and Personalization in a Recipe Recommender System Conference
Nudging Towards Health? Examining the Merits of Nutrition Labels and Personalization in a Recipe Recommender System, 2022.
@conference{Majjodi2022,
title = {Nudging Towards Health? Examining the Merits of Nutrition Labels and Personalization in a Recipe Recommender System},
author = { Ayoub El Majjodi and Alain D. Starke and Christoph Trattner
},
url = {https://dl.acm.org/doi/10.1145/3503252.3531312?fbclid=IwAR0eb6MPuISpVs9Vfkd-ww_KN7EjbMbiGdDQnPxjayogfKbHFgkSgeLdaxs},
year = {2022},
date = {2022-07-03},
urldate = {2022-07-03},
booktitle = {Nudging Towards Health? Examining the Merits of Nutrition Labels and Personalization in a Recipe Recommender System},
abstract = {Food recommender systems show personalized recipes to users based on content liked previously. Despite their potential, often recommended (popular) recipes in previous studies have turned out to be unhealthy, negatively contributing to prevalent obesity problems worldwide. Changing how foods are presented through digital nudges might help, but these are usually examined in non-personalized contexts, such as a brick-and-mortar supermarket. This study seeks to support healthy food choices in a personalized interface by adding front-of-package nutrition labels to recipes in a food recommender system. After performing an offline evaluation, we conducted an online study (N = 600) with six different recommender interfaces, based on a 2 (non-personalized vs. personalized recipe advice) x 3 (No Label, Multiple Traffic Light, Nutri-Score) between-subjects design. We found that recipe choices made in the non-personalized scenario were healthier, while the use of nutrition labels (our digital nudge) reduced choice difficulty when the content was personalized.},
keywords = {},
pubstate = {published},
tppubtype = {conference}
}
Mehdi Elahi; Alain D. Starke; Nabil El Ioini; Anna Alexander Lambrix; Christoph Trattner
Developing and Evaluating a University Recommender System Journal Article
In: Frontiers in Artificial Intelligence , 2022.
@article{Elahi2022,
title = {Developing and Evaluating a University Recommender System},
author = {Mehdi Elahi and Alain D. Starke and Nabil El Ioini and Anna Alexander Lambrix and Christoph Trattner},
url = {https://www.frontiersin.org/articles/10.3389/frai.2021.796268/full},
doi = {https://doi.org/10.3389/frai.2021.796268},
year = {2022},
date = {2022-02-02},
journal = {Frontiers in Artificial Intelligence },
abstract = {A challenge for many young adults is to find the right institution to follow higher education. Global university rankings are a commonly used, but inefficient tool, for they do not consider a person's preferences and needs. For example, some persons pursue prestige in their higher education, while others prefer proximity. This paper develops and evaluates a university recommender system, eliciting user preferences as ratings to build predictive models and to generate personalized university ranking lists. In Study 1, we performed offline evaluation on a rating dataset to determine which recommender approaches had the highest predictive value. In Study 2, we selected three algorithms to produce different university recommendation lists in our online tool, asking our users to compare and evaluate them in terms of different metrics (Accuracy, Diversity, Perceived Personalization, Satisfaction, and Novelty). We show that a SVD algorithm scores high on accuracy and perceived personalization, while a KNN algorithm scores better on novelty. We also report findings on preferred university features.},
keywords = {},
pubstate = {published},
tppubtype = {article}
}
2021
Alain D. Starke; Martijn C. Willemsen; Christoph Trattner
Nudging Healthy Choices in Food Search Through Visual Attractiveness Journal Article
In: no. April 2021, pp. 1-18, 2021.
@article{Starke2021,
title = {Nudging Healthy Choices in Food Search Through Visual Attractiveness},
author = {Alain D. Starke and Martijn C. Willemsen and Christoph Trattner},
url = {https://www.frontiersin.org/articles/10.3389/frai.2021.621743/full},
doi = {10.3389/frai.2021.621743},
year = {2021},
date = {2021-04-22},
number = {April 2021},
pages = {1-18},
abstract = {Recipe websites are becoming increasingly popular to support people in their home cooking. However, most of these websites prioritize popular recipes, which tend to be unhealthy. Drawing upon research on visual biases and nudges, this paper investigates whether healthy food choices can be supported in food search by depicting attractive images alongside recipes, as well as by re-ranking search results on health. After modelling the visual attractiveness of recipe images, we asked 239 users to search for specific online recipes and to select those they liked the most. Our analyses revealed that users tended to choose a healthier recipe if a visually attractive image was depicted alongside it, as well as if it was listed at the top of a list of search results. Even though less popular recipes were promoted this way, it did not come at the cost of a user’s level of satisfaction},
keywords = {},
pubstate = {published},
tppubtype = {article}
}
2020
Ulle Endriss; Ronald de Haan; Jerôme Lang; Marija Slavkovik
The complexity landscape of outcome determination in judgment aggregation Journal Article
In: Journal of Artificial Intelligence Research, vol. 69, pp. 687–731, 2020, (Pre SFI).
@article{Endriss2020,
title = {The complexity landscape of outcome determination in judgment aggregation},
author = {Ulle Endriss and Ronald de Haan and Jerôme Lang and Marija Slavkovik },
url = {https://www.jair.org/index.php/jair/article/view/11970/26619},
doi = {10.1613/jair.1.11970},
year = {2020},
date = {2020-11-04},
journal = {Journal of Artificial Intelligence Research},
volume = {69},
pages = {687–731},
abstract = {We provide a comprehensive analysis of the computational complexity of the outcome determinationproblem for the most important aggregation rules proposed in the literature on logic-based judgmentaggregation. Judgment aggregation is a powerful and flexible framework for studying problems ofcollective decision making that has attracted interest in a range of disciplines, including Legal Theory,Philosophy, Economics, Political Science, and Artificial Intelligence. The problem of computing theoutcome for a given list of individual judgments to be aggregated into a single collective judgment isthe most fundamental algorithmic challenge arising in this context. Our analysis applies to severaldifferent variants of the basic framework of judgment aggregation that have been discussed in theliterature, as well as to a new framework that encompasses all existing such frameworks in terms ofexpressive power and representational succinctness.},
note = {Pre SFI},
keywords = {},
pubstate = {published},
tppubtype = {article}
}
Naieme Hazrati; Mehdi Elahi
Addressing the New Item problem in video recommender systems by incorporation of visual features with restricted Boltzmann machines. Journal Article
In: Expert Systems, vol. e12645, pp. 1-20, 2020, (Pre SFI).
@article{Hazrati2020,
title = { Addressing the New Item problem in video recommender systems by incorporation of visual features with restricted Boltzmann machines.},
author = {Naieme Hazrati and Mehdi Elahi},
url = {https://onlinelibrary.wiley.com/doi/epdf/10.1111/exsy.12645},
doi = {https://doi.org/10.1111/exsy.12645},
year = {2020},
date = {2020-10-19},
journal = {Expert Systems},
volume = {e12645},
pages = {1-20},
abstract = {Over the past years, the research of video recommender systems (RSs) has been mainly focussed on the development of novel algorithms. Although beneficial, still any algorithm may fail to recommend video items that the system has no form of data associated to them (New Item Cold Start). This problem occurs when a new item is added to the catalogue of the system and no data are available for that item. In content‐based RSs, the video items are typically represented by semantic attributes, when generating recommendations. These attributes require a group of experts or users for annotation, and still, the generated recommendations might not capture a complete picture of the users' preferences, for example, the visual tastes of users on video style. This article addresses this problem by proposing recommendation based on novel visual features that do not require human annotation and can represent visual aspects of video items. We have designed a novel evaluation methodology considering three realistic scenarios, that is, (a) extreme cold start, (b) moderate cold start and (c) warm‐start scenario. We have conducted a set of comprehensive experiments, and our results have shown the superior performance of recommendations based on visual features, in all of the evaluation scenarios.},
note = {Pre SFI},
keywords = {},
pubstate = {published},
tppubtype = {article}
}
Krisztian Balog; Filip Radlinski
Measuring Recommendation Explanation Quality: The Conflicting Goals of Explanations Conference
Proceedings of the 43rd International ACM SIGIR Conference on Research and Development in Information Retrieval (SIGIR '20), New York, 2020, (Pre SFI).
@conference{Balog2020,
title = {Measuring Recommendation Explanation Quality: The Conflicting Goals of Explanations},
author = {Krisztian Balog and Filip Radlinski},
url = {https://dl.acm.org/doi/pdf/10.1145/3397271.3401032},
doi = {https://doi.org/10.1145/3397271.3401032},
year = {2020},
date = {2020-07-01},
booktitle = {Proceedings of the 43rd International ACM SIGIR Conference on Research and Development in Information Retrieval (SIGIR '20)},
pages = {329–338},
address = {New York},
abstract = {Explanations have a large effect on how people respond to recommendations. However, there are many possible intentions a system may have in generating explanations for a given recommendation -from increasing transparency, to enabling a faster decision, to persuading the recipient. As a good explanation for one goal may not be good for others, we address the questions of (1) how to robustly measure if an explanation meets a given goal and (2) how the different goals interact with each other. Specifically, this paper presents a first proposal of how to measure the quality of explanations along seven common goal dimensions catalogued in the literature. We find that the seven goals are not independent, but rather exhibit strong structure. Proposing two novel explanation evaluation designs, we identify challenges in evaluation, and provide more efficient measurement approaches of explanation quality.},
note = {Pre SFI},
keywords = {},
pubstate = {published},
tppubtype = {conference}
}
Than Htut Soe; Oda Elise Nordberg; Frode Guribye; Marija Slavkovik
Circumvention by design - dark patterns in cookie consents for online news outlets Conference
Proceedings of the 11th Nordic Conference on Human-Computer Interaction, 2020, (Pre SFI).
@conference{Soe2020,
title = {Circumvention by design - dark patterns in cookie consents for online news outlets},
author = {Than Htut Soe and Oda Elise Nordberg and Frode Guribye and Marija Slavkovik},
url = {https://dl.acm.org/doi/pdf/10.1145/3419249.3420132},
doi = {10.1145/3419249.3420132},
year = {2020},
date = {2020-06-24},
booktitle = {Proceedings of the 11th Nordic Conference on Human-Computer Interaction},
abstract = {To ensure that users of online services understand what data are collected and how they are used in algorithmic decision-making, the European Union's General Data Protection Regulation (GDPR) specifies informed consent as a minimal requirement. For online news outlets consent is commonly elicited through interface design elements in the form of a pop-up. We have manually analyzed 300 data collection consent notices from news outlets that are built to ensure compliance with GDPR. The analysis uncovered a variety of strategies or dark patterns that circumvent the intent of GDPR by design. We further study the presence and variety of these dark patterns in these "cookie consents" and use our observations to specify the concept of dark pattern in the context of consent elicitation.},
note = {Pre SFI},
keywords = {},
pubstate = {published},
tppubtype = {conference}
}
Ingrid Nunes; Dietmar Jannach
A Systematic Review and Taxonomy of Explanations in Decision Support and Recommender Systems Journal Article
In: User-Modeling and User-Adapted Interaction, vol. 27, no. 3-5, pp. 393-444, 2020, (Pre SFI).
@article{Nunes2020,
title = {A Systematic Review and Taxonomy of Explanations in Decision Support and Recommender Systems},
author = {Ingrid Nunes and Dietmar Jannach},
url = {https://arxiv.org/pdf/2006.08672.pdf},
doi = {10.1007/s11257-017-9195-0},
year = {2020},
date = {2020-06-15},
journal = {User-Modeling and User-Adapted Interaction},
volume = {27},
number = {3-5},
pages = {393-444},
abstract = {With the recent advances in the field of artificial intelligence, an increasing number of decision-making tasks are delegated to software systems. A key requirement for the success and adoption of such systems is that users must trust system choices or even fully automated decisions. To achieve this, explanation facilities have been widely investigated as a means of establishing trust in these systems since the early years of expert systems. With today's increasingly sophisticated machine learning algorithms, new challenges in the context of explanations, accountability, and trust towards such systems constantly arise. In this work, we systematically review the literature on explanations in advice-giving systems. This is a family of systems that includes recommender systems, which is one of the most successful classes of advice-giving software in practice. We investigate the purposes of explanations as well as how they are generated, presented to users, and evaluated. As a result, we derive a novel comprehensive taxonomy of aspects to be considered when designing explanation facilities for current and future decision support systems. The taxonomy includes a variety of different facets, such as explanation objective, responsiveness, content and presentation. Moreover, we identified several challenges that remain unaddressed so far, for example related to fine-grained issues associated with the presentation of explanations and how explanation facilities are evaluated.},
note = {Pre SFI},
keywords = {},
pubstate = {published},
tppubtype = {article}
}
Helle Sjøvaag; Thomas Owren; Turid Borgen
Strategic and Organisational fit in Corporate News Markets: A Principal-agent Approach to Studying Newspaper Mergers Journal Article
In: Journalism Practice, pp. 1-18, 2020, (Pre SFI).
@article{Sjøvaag2020,
title = {Strategic and Organisational fit in Corporate News Markets: A Principal-agent Approach to Studying Newspaper Mergers},
author = {Helle Sjøvaag and Thomas Owren and Turid Borgen},
url = {https://www.tandfonline.com/doi/epub/10.1080/17512786.2020.1772097?needAccess=true},
doi = {https://doi.org/10.1080/17512786.2020.1772097},
year = {2020},
date = {2020-05-20},
journal = {Journalism Practice},
pages = {1-18},
abstract = {This article analyses strategic and organisational fit in corporate newspaper mergers in the context of the digitalisation of local newspaper markets. Using the 2019 acquisition of Nordsjø Media by Amedia in Norway as case, we analyse how eight editors-in-chief perceive the process of incorporating small, low-frequency, print-oriented monopolistic newspapers into one of Scandinavia’s largest newspaper chains. The semi-structured interviews were analysed in light of perceived strategic and organisational fit in a principal-agent theoretical framework, the aim of which is to shed light on corporate ownership effects in consolidated newspaper markets. The analysis reveals the precarity of independent ownership in digitising news markets, to which corporatisation emerges as a necessary and welcomed solution. We find the strategic fit as perceived by editors to be tied to technological resources and scale economics, while organisational fit is hampered by the speed and pressure of corporatisation processes. While these results largely support findings from previous acquisition studies in the news industries, the contribution of this analysis lies primarily with the necessity of scale required by the technological transformation that forces independent newspapers to submit to larger chain operations and how it influences considerations of fit in disruptive digital news markets.},
note = {Pre SFI},
keywords = {},
pubstate = {published},
tppubtype = {article}
}
Shuo Zhang; Krisztian Balog
Web Table Extraction, Retrieval, and Augmentation: A Survey Journal Article
In: ACM Transactions on Intelligent Systems and Technology (TIST), vol. 11, no. 2, pp. 1-35, 2020, (Pre SFI).
@article{Zhang2020,
title = {Web Table Extraction, Retrieval, and Augmentation: A Survey},
author = {Shuo Zhang and Krisztian Balog},
url = {https://arxiv.org/pdf/2002.00207.pdf},
year = {2020},
date = {2020-02-01},
journal = {ACM Transactions on Intelligent Systems and Technology (TIST)},
volume = {11},
number = {2},
pages = {1-35},
abstract = {Tables are a powerful and popular tool for organizing and manipulating data. A vast number of tables can be found on the Web, which represents a valuable knowledge resource. The objective of this survey is to synthesize and present two decades of research on web tables. In particular, we organize existing literature into six main categories of information access tasks: table extraction, table interpretation, table search, question answering, knowledge base augmentation, and table augmentation. For each of these tasks, we identify and describe seminal approaches, present relevant resources, and point out interdependencies among the different tasks.},
note = {Pre SFI},
keywords = {},
pubstate = {published},
tppubtype = {article}
}
2019
Maurizio Ferrari Dacrema; Paolo Cremonesi; Dietmar Jannach
Are We Really Making Much Progress? A Worrying Analysis of Recent Neural Recommendation Approaches Conference
Proceedings of the 2019 ACM Conference on Recommender Systems (RecSys 2019), Copenhagen, 2019, (Pre SFI).
@conference{Dacrema2019,
title = {Are We Really Making Much Progress? A Worrying Analysis of Recent Neural Recommendation Approaches},
author = {Maurizio Ferrari Dacrema and Paolo Cremonesi and Dietmar Jannach},
url = {https://arxiv.org/pdf/1907.06902.pdf},
year = {2019},
date = {2019-08-16},
booktitle = {Proceedings of the 2019 ACM Conference on Recommender Systems (RecSys 2019)},
address = {Copenhagen},
abstract = {Deep learning techniques have become the method of choice for
researchers working on algorithmic aspects of recommender systems. With the strongly increased interest in machine learning in
general, it has, as a result, become difficult to keep track of what
represents the state-of-the-art at the moment, e.g., for top-n recommendation tasks. At the same time, several recent publications
point out problems in today’s research practice in applied machine
learning, e.g., in terms of the reproducibility of the results or the
choice of the baselines when proposing new models.
In this work, we report the results of a systematic analysis of algorithmic proposals for top-n recommendation tasks. Specifically,
we considered 18 algorithms that were presented at top-level research conferences in the last years. Only 7 of them could be reproduced with reasonable effort. For these methods, it however
turned out that 6 of them can often be outperformed with comparably simple heuristic methods, e.g., based on nearest-neighbor or
graph-based techniques. The remaining one clearly outperformed
the baselines but did not consistently outperform a well-tuned nonneural linear ranking method. Overall, our work sheds light on a
number of potential problems in today’s machine learning scholarship and calls for improved scientific practices in this area.},
note = {Pre SFI},
keywords = {},
pubstate = {published},
tppubtype = {conference}
}
researchers working on algorithmic aspects of recommender systems. With the strongly increased interest in machine learning in
general, it has, as a result, become difficult to keep track of what
represents the state-of-the-art at the moment, e.g., for top-n recommendation tasks. At the same time, several recent publications
point out problems in today’s research practice in applied machine
learning, e.g., in terms of the reproducibility of the results or the
choice of the baselines when proposing new models.
In this work, we report the results of a systematic analysis of algorithmic proposals for top-n recommendation tasks. Specifically,
we considered 18 algorithms that were presented at top-level research conferences in the last years. Only 7 of them could be reproduced with reasonable effort. For these methods, it however
turned out that 6 of them can often be outperformed with comparably simple heuristic methods, e.g., based on nearest-neighbor or
graph-based techniques. The remaining one clearly outperformed
the baselines but did not consistently outperform a well-tuned nonneural linear ranking method. Overall, our work sheds light on a
number of potential problems in today’s machine learning scholarship and calls for improved scientific practices in this area.
Ronald de Haan; Marija Slavkovik
Answer set programming for judgment aggregation Conference
Proceedings of the Twenty-Eighth International Joint Conference on Artificial Intelligence, International Joint Conferences on Artificial Intelligence, 2019, (Pre SFI).
@conference{deHaan2019,
title = {Answer set programming for judgment aggregation},
author = {Ronald de Haan and Marija Slavkovik},
url = {https://www.ijcai.org/Proceedings/2019/0231.pdf},
year = {2019},
date = {2019-08-10},
booktitle = {Proceedings of the Twenty-Eighth International Joint Conference on Artificial Intelligence},
pages = {1668-1674},
publisher = {International Joint Conferences on Artificial Intelligence},
abstract = {Judgment aggregation (JA) studies how to aggregate truth valuations on logically related issues. Computing the outcome of aggregation procedures is notoriously computationally hard, which is the likely reason that no implementation of them exists as of yet. However, even hard problems sometimes need to be solved. The worst-case computational complexity of answer set programming (ASP) matches that of most problems in judgment aggregation. We take advantage of this and propose a natural and modular encoding of various judgment aggregation procedures and related problems in JA into ASP. With these encodings, we achieve two results: (1) paving the way towards constructing a wide range of new benchmark instances (from JA) for answer set solving algorithms; and (2) providing an automated tool for researchers in the area of judgment aggregation.},
note = {Pre SFI},
keywords = {},
pubstate = {published},
tppubtype = {conference}
}
Krisztian Balog; Filip Radlinski; Shushan Arakelyan
Transparent, Scrutable and Explainable User Models for Personalized Recommendation Conference
Proceedings of the 42nd International ACM SIGIR Conference on Research and Development in Information Retrieval (SIGIR’19), 2019, (Pre SFI).
@conference{Balog2019,
title = {Transparent, Scrutable and Explainable User Models for Personalized Recommendation},
author = {Krisztian Balog and Filip Radlinski and Shushan Arakelyan},
url = {https://dl.acm.org/doi/pdf/10.1145/3331184.3331211},
doi = {https://doi.org/10.1145/3331184.3331211},
year = {2019},
date = {2019-07-25},
booktitle = {Proceedings of the 42nd International ACM SIGIR Conference on Research and Development in Information Retrieval (SIGIR’19)},
pages = {265–274},
abstract = {Most recommender systems base their recommendations on implicit or explicit item-level feedback provided by users. These item ratings are combined into a complex user model, which then predicts the suitability of other items. While effective, such methods have limited scrutability and transparency. For instance, if a user's interests change, then many item ratings would usually need to be modified to significantly shift the user's recommendations. Similarly, explaining how the system characterizes the user is impossible, short of presenting the entire list of known item ratings. In this paper, we present a new set-based recommendation technique that permits the user model to be explicitly presented to users in natural language, empowering users to understand recommendations made and improve the recommendations dynamically. While performing comparably to traditional collaborative filtering techniques in a standard static setting, our approach allows users to efficiently improve recommendations. Further, it makes it easier for the model to be validated and adjusted, building user trust and understanding.},
note = {Pre SFI},
keywords = {},
pubstate = {published},
tppubtype = {conference}
}
Helle Sjøvaag
Journalism between the state and the market Book
Routledge, New York, 2019, ISBN: 9781138543348, (Pre SFI).
@book{Sjøvaag2019,
title = {Journalism between the state and the market},
author = {Helle Sjøvaag},
url = {https://www.researchgate.net/publication/332449932_Journalism_Between_the_State_and_the_Market},
isbn = {9781138543348},
year = {2019},
date = {2019-04-24},
publisher = {Routledge},
address = {New York},
abstract = {Using the Nordic media model as an empirical backdrop, Journalism Between the State and the Market defines and analyzes journalism’s fundamental problem: its shifting location between the state and the market.
This book examines how this distance is decreasing as journalism steps closer to both the market (algorithmically monetizing audiences) and the state (lobbying governments for subsidies and attacking public service broadcasting). The book analyzes journalism’s negotiated position between the market and the state in the age of disruptions, offering a theoretical foundation that seeks to account for the structural conditions of journalism in the digital age.
For scholars, graduates and students in journalism, news sociology and media and communication studies, Journalism Between the State and the Market provides a theoretical perspective that can be used as a valuable tool when studying and observing the current developments in journalism.},
note = {Pre SFI},
keywords = {},
pubstate = {published},
tppubtype = {book}
}
This book examines how this distance is decreasing as journalism steps closer to both the market (algorithmically monetizing audiences) and the state (lobbying governments for subsidies and attacking public service broadcasting). The book analyzes journalism’s negotiated position between the market and the state in the age of disruptions, offering a theoretical foundation that seeks to account for the structural conditions of journalism in the digital age.
For scholars, graduates and students in journalism, news sociology and media and communication studies, Journalism Between the State and the Market provides a theoretical perspective that can be used as a valuable tool when studying and observing the current developments in journalism.
Beishui Liao; Marija Slavkovik; Leendert van der Torre
Building Jiminy Cricket: An architecture for moral agreements among stakeholders Conference
Proceedings of the 2019 AAAI/ACM Conference on AI, Ethics, and Society, 2019, (Pre SFI).
@conference{Liao2019,
title = {Building Jiminy Cricket: An architecture for moral agreements among stakeholders},
author = {Beishui Liao and Marija Slavkovik and Leendert van der Torre},
url = {https://arxiv.org/pdf/1812.04741.pdf},
year = {2019},
date = {2019-03-07},
booktitle = {Proceedings of the 2019 AAAI/ACM Conference on AI, Ethics, and Society},
pages = {147–153},
abstract = {An autonomous system is constructed by a manufacturer, operates in a society subject to norms and laws, and is interacting with end-users. We address the
challenge of how the moral values and views of all stakeholders can be integrated
and reflected in the moral behaviour of the autonomous system. We propose an artificial moral agent architecture that uses techniques from normative systems and
formal argumentation to reach moral agreements among stakeholders. We show
how our architecture can be used not only for ethical practical reasoning and collaborative decision-making, but also for the explanation of such moral behavior.},
note = {Pre SFI},
keywords = {},
pubstate = {published},
tppubtype = {conference}
}
challenge of how the moral values and views of all stakeholders can be integrated
and reflected in the moral behaviour of the autonomous system. We propose an artificial moral agent architecture that uses techniques from normative systems and
formal argumentation to reach moral agreements among stakeholders. We show
how our architecture can be used not only for ethical practical reasoning and collaborative decision-making, but also for the explanation of such moral behavior.
2018
Helle Sjøvaag; Truls André Pedersen; Thomas Owren
Is public service broadcasting a threat to commercial media? Journal Article
In: Media, Culture & Society, vol. 46, no. 1, pp. 808-827, 2018, (Pre SFI).
@article{Sjøvaag2018c,
title = {Is public service broadcasting a threat to commercial media?},
author = {Helle Sjøvaag and Truls André Pedersen and Thomas Owren},
url = {https://journals.sagepub.com/doi/pdf/10.1177/0163443718818354},
doi = {https://doi.org/10.1177/0163443718818354},
year = {2018},
date = {2018-12-20},
journal = {Media, Culture & Society},
volume = {46},
number = {1},
pages = {808-827},
abstract = {This article asks to what extent public service broadcasting’s online news service resembles that of commercial media. The context of this inquiry is claims of ‘out-crowding’ facing public service broadcasters across Europe. In Norway, commercial players in this debate accuse the public service broadcaster, NRK, of being too similar to competitors in the private sector for commercial operators to attain sustainable revenues in the online realm. To ascertain the extent to what these claims are warranted, this article compares NRK’s online content with that of nine commercial competitors in national and local markets, using a hybrid methodological approach combining quantitative content analysis with Latent Dirichlet allocation, analysing in excess of 115,000 documents. Findings show that commercial operators resemble each other more than they do NRK, indicating closer competition in the commercial segment than between the public service broadcaster and market players.},
note = {Pre SFI},
keywords = {},
pubstate = {published},
tppubtype = {article}
}
Sjur Dyrkolbotn; Truls Pedersen; Marija Slavkovik
On the distinction between implicit and explicit ethical agency Conference
Proceedings of the 2018 AAAI/ACM Conference on AI, Ethics, and Society (AIES '18), 2018, (Pre SFI).
@conference{Dyrkolbotn2018,
title = {On the distinction between implicit and explicit ethical agency},
author = {Sjur Dyrkolbotn and Truls Pedersen and Marija Slavkovik},
url = {https://dl.acm.org/doi/pdf/10.1145/3278721.3278769},
doi = {https://doi.org/10.1145/3278721.3278769},
year = {2018},
date = {2018-12-01},
booktitle = {Proceedings of the 2018 AAAI/ACM Conference on AI, Ethics, and Society (AIES '18)},
pages = {74–80},
abstract = {With recent advances in artificial intelligence and the rapidly increasing importance of autonomous intelligent systems in society, it is becoming clear that artificial agents will have to be designed to comply with complex ethical standards. As we work to develop moral machines, we also push the boundaries of existing legal categories. The most pressing question is what kind of ethical decision-making our machines are actually able to engage in. Both in law and in ethics, the concept of agency forms a basis for further legal and ethical categorisations, pertaining to decision-making ability. Hence, without a cross-disciplinary understanding of what we mean by ethical agency in machines, the question of responsibility and liability cannot be clearly addressed. Here we make first steps towards a comprehensive definition, by suggesting ways to distinguish between implicit and explicit forms of ethical agency.},
note = {Pre SFI},
keywords = {},
pubstate = {published},
tppubtype = {conference}
}
Helle Sjøvaag; Truls André Pedersen
Female voices in the news: Structural conditions of gender representations in Norwegian newspapers Journal Article
In: Journalism & Mass Communication Quarterly, vol. 96, no. 1, pp. 215-238, 2018, (Pre SFI).
@article{Sjøvaag2018b,
title = {Female voices in the news: Structural conditions of gender representations in Norwegian newspapers},
author = {Helle Sjøvaag and Truls André Pedersen},
url = {https://journals.sagepub.com/doi/pdf/10.1177/1077699018789885},
doi = {https://doi.org/10.1177/1077699018789885},
year = {2018},
date = {2018-08-21},
journal = {Journalism & Mass Communication Quarterly},
volume = {96},
number = {1},
pages = {215-238},
abstract = {The article presents a hybrid analysis combining manual content analysis of 9,131 sources in 5,544 news stories across 75 publications, with computational gender recognition producing 551,102 names from 320,228 articles across 125 newspapers. The article investigates the significance of structural features for the presence of women in the news. Results show female sources are only equal to men as ordinary citizens and children, and only in lifestyle content. Among the structural features examined, only local distribution and a circulation less than 5,000 exhibit improvements in female representation. Ownership, distribution frequency, market position, and direct press support had little or no effect.},
note = {Pre SFI},
keywords = {},
pubstate = {published},
tppubtype = {article}
}
Helle Sjøvaag; Eirik Stavelin; Michael Karlsson; Aske Kammer
The hyperlinked Scandinavian news ecology: The unequal terms forged by the structural properties of digitalisation Journal Article
In: Digital Journalism, vol. 7, no. 4, pp. 507-531, 2018, (Pre SFI).
@article{Sjøvaag2018,
title = {The hyperlinked Scandinavian news ecology: The unequal terms forged by the structural properties of digitalisation},
author = {Helle Sjøvaag and Eirik Stavelin and Michael Karlsson and Aske Kammer},
url = {https://www.tandfonline.com/doi/epub/10.1080/21670811.2018.1454335?needAccess=true},
doi = {https://doi.org/10.1080/21670811.2018.1454335},
year = {2018},
date = {2018-04-06},
journal = {Digital Journalism},
volume = {7},
number = {4},
pages = {507-531},
abstract = {The article presents a network analysis of 22,861,013 geocoded external hyperlinks, collected from 230 Danish, 220 Norwegian and 208 Swedish news websites in 2016. The analysis asks what the structural properties of the Scandinavian media systems—including its geography and ownership structures—mean for news outlets’ centrality within the hyperlinked news ecology. The analysis finds that whereas incumbent legacy media occupy central positions, about one third of the network is absent from the hyperlinked interaction, primarily local, independently owned newspapers. A multiple linear regression analysis shows that national distribution and corporate ownership correlates to network centrality more than other predictors. As brokers in the network consist of the large, legacy, capital-based news organisations, hyperlink connectivity is primarily characterised by proximity to the centres of power, corporate ownership, agenda setting incumbency and national distribution.},
note = {Pre SFI},
keywords = {},
pubstate = {published},
tppubtype = {article}
}
Malte Ludewig; Dietmar Jannach
Evaluation of Session-based Recommendation Algorithms Journal Article
In: User-Modeling and User-Adapted Interaction, vol. 28, no. 4-5, pp. 331-390, 2018, (Pre SFI).
@article{Ludewig2018,
title = {Evaluation of Session-based Recommendation Algorithms},
author = {Malte Ludewig and Dietmar Jannach},
url = {https://arxiv.org/pdf/1803.09587.pdf},
doi = {10.1007/s11257-018-9209-6},
year = {2018},
date = {2018-03-26},
journal = {User-Modeling and User-Adapted Interaction},
volume = {28},
number = {4-5},
pages = {331-390},
abstract = {Recommender systems help users find relevant items of interest, for example on e-commerce or media streaming sites. Most academic research is concerned with approaches that personalize the recommendations according to long-term user profiles. In many real-world applications, however, such long-term profiles often do not exist and recommendations therefore have to be made solely based on the observed behavior of a user during an ongoing session. Given the high practical relevance of the problem, an increased interest in this problem can be observed in recent years, leading to a number of proposals for session-based recommendation algorithms that typically aim to predict the user's immediate next actions. In this work, we present the results of an in-depth performance comparison of a number of such algorithms, using a variety of datasets and evaluation measures. Our comparison includes the most recent approaches based on recurrent neural networks like GRU4REC, factorized Markov model approaches such as FISM or FOSSIL, as well as simpler methods based, e.g., on nearest neighbor schemes. Our experiments reveal that algorithms of this latter class, despite their sometimes almost trivial nature, often perform equally well or significantly better than today's more complex approaches based on deep neural networks. Our results therefore suggest that there is substantial room for improvement regarding the development of more sophisticated session-based recommendation algorithms.},
note = {Pre SFI},
keywords = {},
pubstate = {published},
tppubtype = {article}
}
Massimo Quadrana; Paolo Cremonesi; Dietmar Jannach
Sequence-Aware Recommender Systems Journal Article
In: ACM Computing Surveys, vol. 51, no. 4, pp. 1-35, 2018, (Pre SFI).
@article{Quadrana2018,
title = {Sequence-Aware Recommender Systems},
author = {Massimo Quadrana and Paolo Cremonesi and Dietmar Jannach},
url = {https://arxiv.org/pdf/1802.08452.pdf},
year = {2018},
date = {2018-02-23},
journal = {ACM Computing Surveys},
volume = {51},
number = {4},
pages = {1-35},
abstract = {Recommender systems are one of the most successful applications of data mining and machine learning technology in practice. Academic research in the field is historically often based on the matrix completion problem formulation, where for each user-item-pair only one interaction (e.g., a rating) is considered. In many application domains, however, multiple user-item interactions of different types can be recorded over time. And, a number of recent works have shown that this information can be used to build richer individual user models and to discover additional behavioral patterns that can be leveraged in the recommendation process. In this work we review existing works that consider information from such sequentially-ordered user- item interaction logs in the recommendation process. Based on this review, we propose a categorization of the corresponding recommendation tasks and goals, summarize existing algorithmic solutions, discuss methodological approaches when benchmarking what we call sequence-aware recommender systems, and outline open challenges in the area.},
note = {Pre SFI},
keywords = {},
pubstate = {published},
tppubtype = {article}
}
Shuo Zhang; Krisztian Balog
Ad Hoc Table Retrieval using Semantic Similarity Conference
Proceedings of The Web Conference 2018 (WWW’18), 2018, (Pre SFI).
@conference{Zhang2018,
title = {Ad Hoc Table Retrieval using Semantic Similarity},
author = {Shuo Zhang and Krisztian Balog},
url = {https://arxiv.org/pdf/1802.06159.pdf},
doi = {10.1145/3178876.3186067},
year = {2018},
date = {2018-02-16},
booktitle = {Proceedings of The Web Conference 2018 (WWW’18)},
pages = {1553-1562},
abstract = {We introduce and address the problem of ad hoc table retrieval: answering a keyword query with a ranked list of tables. This task is not only interesting on its own account, but is also being used as a core component in many other table-based information access scenarios, such as table completion or table mining. The main novel contribution of this work is a method for performing semantic matching between queries and tables. Specifically, we (i) represent queries and tables in multiple semantic spaces (both discrete sparse and continuous dense vector representations) and (ii) introduce various similarity measures for matching those semantic representations. We consider all possible combinations of semantic representations and similarity measures and use these as features in a supervised learning model. Using a purpose-built test collection based on Wikipedia tables, we demonstrate significant and substantial improvements over a state-of-the-art baseline.},
note = {Pre SFI},
keywords = {},
pubstate = {published},
tppubtype = {conference}
}
Krisztian Balog
Entity-Oriented Search Book
1, Springer, 2018, ISBN: 978-3-319-93935-3, (Pre SFI).
@book{Balog2018,
title = {Entity-Oriented Search},
author = {Krisztian Balog},
url = {https://www.springer.com/gp/book/9783319939339},
doi = {10.1007/978-3-319-93935-3},
isbn = {978-3-319-93935-3},
year = {2018},
date = {2018-01-01},
volume = {39},
publisher = {Springer},
edition = {1},
abstract = {This open access book covers all facets of entity-oriented search—where “search” can be interpreted in the broadest sense of information access—from a unified point of view, and provides a coherent and comprehensive overview of the state of the art. It represents the first synthesis of research in this broad and rapidly developing area. Selected topics are discussed in-depth, the goal being to establish fundamental techniques and methods as a basis for future research and development. Additional topics are treated at a survey level only, containing numerous pointers to the relevant literature. A roadmap for future research, based on open issues and challenges identified along the way, rounds out the book.},
note = {Pre SFI},
keywords = {},
pubstate = {published},
tppubtype = {book}
}
2017
Mehdi Elahi; Yashar Deldjoo; Farshad Bakhshandegan Moghaddam; Leonardo Cella; Stefano Cerada; Paolo Cremonesi
Exploring the semantic gap for movie recommendations Conference
Proceedings of the Eleventh ACM Conference on Recommender Systems, Association for Computing Machinery New York, 2017, (Pre SFI).
@conference{Elahi2017,
title = {Exploring the semantic gap for movie recommendations},
author = {Mehdi Elahi and Yashar Deldjoo and Farshad Bakhshandegan Moghaddam and Leonardo Cella and Stefano Cerada and Paolo Cremonesi },
url = {https://dl.acm.org/doi/pdf/10.1145/3109859.3109908},
doi = {https://doi.org/10.1145/3109859.3109908},
year = {2017},
date = {2017-08-01},
booktitle = {Proceedings of the Eleventh ACM Conference on Recommender Systems},
pages = {326–330},
address = {New York},
organization = {Association for Computing Machinery},
abstract = {In the last years, there has been much attention given to the semantic gap problem in multimedia retrieval systems. Much effort has been devoted to bridge this gap by building tools for the extraction of high-level, semantics-based features from multimedia content, as low-level features are not considered useful because they deal primarily with representing the perceived content rather than the semantics of it.
In this paper, we explore a different point of view by leveraging the gap between low-level and high-level features. We experiment with a recent approach for movie recommendation that extract low-level Mise-en-Scéne features from multimedia content and combine it with high-level features provided by the wisdom of the crowd.
To this end, we first performed an offline performance assessment by implementing a pure content-based recommender system with three different versions of the same algorithm, respectively based on (i) conventional movie attributes, (ii) mise-en-scene features, and (iii) a hybrid method that interleaves recommendations based on movie attributes and mise-en-scene features. In a second study, we designed an empirical study involving 100 subjects and collected data regarding the quality perceived by the users. Results from both studies show that the introduction of mise-en-scéne features in conjunction with traditional movie attributes improves both offline and online quality of recommendations.},
note = {Pre SFI},
keywords = {},
pubstate = {published},
tppubtype = {conference}
}
In this paper, we explore a different point of view by leveraging the gap between low-level and high-level features. We experiment with a recent approach for movie recommendation that extract low-level Mise-en-Scéne features from multimedia content and combine it with high-level features provided by the wisdom of the crowd.
To this end, we first performed an offline performance assessment by implementing a pure content-based recommender system with three different versions of the same algorithm, respectively based on (i) conventional movie attributes, (ii) mise-en-scene features, and (iii) a hybrid method that interleaves recommendations based on movie attributes and mise-en-scene features. In a second study, we designed an empirical study involving 100 subjects and collected data regarding the quality perceived by the users. Results from both studies show that the introduction of mise-en-scéne features in conjunction with traditional movie attributes improves both offline and online quality of recommendations.
2016
Dietmar Jannach; Alexander Tuzhilin; Markus Zanker
Recommender Systems - Beyond Matrix Completion Journal Article
In: Communications of the ACM, vol. 59, no. 11, pp. 94-102, 2016, (Pre SFI).
@article{Jannach2016,
title = {Recommender Systems - Beyond Matrix Completion},
author = {Dietmar Jannach and Alexander Tuzhilin and Markus Zanker },
url = {https://www.researchgate.net/publication/309600906_Recommender_systems---_beyond_matrix_completion},
doi = {10.1145/2891406},
year = {2016},
date = {2016-10-01},
journal = {Communications of the ACM},
volume = {59},
number = {11},
pages = {94-102},
abstract = {Recommender systems have become a natural part of the user experience in today's online world. These systems are able to deliver value both for users and providers and are one prominent example where the output of academic research has a direct impact on the advancements in industry. In this article, we have briefy reviewed the history of this multidis-ciplinary field and looked at recent efforts in the research community to consider the variety of factors that may influence the long-term success of a recommender system. The list of open issues and success factors is still far from complete and new challenges arise constantly that require further research. For example, the huge amounts of user data and preference signals that become available through the Social Web and the Internet of Things not only leads to technical challenges such as scalability, but also to societal questions concerning user privacy. Based on our reflections on the developments in the field, we finally emphasize the need for a more holistic research approach that combines the insights of different disciplines. We urge that research focuses even more on practical problems that matter and are truly suited to increase the utility of recommendations from the viewpoint of the users.
},
note = {Pre SFI},
keywords = {},
pubstate = {published},
tppubtype = {article}
}
Mehdi Elahi; Francesco Ricci; Neil Rubens
A survey of active learning in collaborative filtering recommender systems Journal Article
In: Computer Science Review, vol. 20, pp. 29-50, 2016, (Pre SFI).
@article{Elahi2016,
title = {A survey of active learning in collaborative filtering recommender systems},
author = {Mehdi Elahi and Francesco Ricci and Neil Rubens},
url = {https://reader.elsevier.com/reader/sd/pii/S1574013715300150?token=EA12A462FC07F42733F4F13375217A57D3FDC7F6047C133156CB1F4E4487DF24C5366547DF4530A25942F690233F2E30},
doi = {10.1016/j.cosrev.2016.05.002},
year = {2016},
date = {2016-06-02},
journal = {Computer Science Review},
volume = {20},
pages = {29-50},
abstract = {In collaborative filtering recommender systems user’s preferences are expressed as ratings for items, and each additional rating extends the knowledge of the system and affects the system’s recommendation accuracy. In general, the more ratings are elicited from the users, the more effective the recommendations are. However, the usefulness of each rating may vary significantly, i.e., different ratings may bring a different amount and type of information about the user’s tastes. Hence, specific techniques, which are defined as “active learning strategies”, can be used to selectively choose the items to be presented to the user for rating. In fact, an active learning strategy identifies and adopts criteria for obtaining data that better reflects users’ preferences and enables to generate better recommendations.
So far, a variety of active learning strategies have been proposed in the literature. In this article, we survey recent strategies by grouping them with respect to two distinct dimensions: personalization, i.e., whether the system selected items are different for different users or not, and, hybridization, i.e., whether active learning is guided by a single criterion (heuristic) or by multiple criteria. In addition, we present a comprehensive overview of the evaluation methods and metrics that have been employed by the research community in order to test active learning strategies for collaborative filtering. Finally, we compare the surveyed strategies and provide guidelines for their usage in recommender systems.},
note = {Pre SFI},
keywords = {},
pubstate = {published},
tppubtype = {article}
}
So far, a variety of active learning strategies have been proposed in the literature. In this article, we survey recent strategies by grouping them with respect to two distinct dimensions: personalization, i.e., whether the system selected items are different for different users or not, and, hybridization, i.e., whether active learning is guided by a single criterion (heuristic) or by multiple criteria. In addition, we present a comprehensive overview of the evaluation methods and metrics that have been employed by the research community in order to test active learning strategies for collaborative filtering. Finally, we compare the surveyed strategies and provide guidelines for their usage in recommender systems.
Ignacio Fernandez Tobias; Matthias Braunhofer; Mehdi Elahi; Francesco Ricci; Ivan Cantador
Alleviating the new user problem in collaborative filtering by exploiting personality information Journal Article
In: User Modeling and User-Adapted Interaction, vol. 26, no. 2-3, pp. 221-255, 2016, (Pre SFI).
@article{Tobias2016,
title = {Alleviating the new user problem in collaborative filtering by exploiting personality information},
author = {Ignacio Fernandez Tobias and Matthias Braunhofer and Mehdi Elahi and Francesco Ricci and Ivan Cantador},
url = {https://www.researchgate.net/publication/285574429_Alleviating_the_New_User_Problem_in_Collaborative_Filtering_by_Exploiting_Personality_Information},
doi = {10.1007/s11257-016-9172-z},
year = {2016},
date = {2016-06-01},
journal = {User Modeling and User-Adapted Interaction},
volume = {26},
number = {2-3},
pages = {221-255},
abstract = {The new user problem in recommender systems is still challenging, and there is not yet a unique solution that can be applied in any domain or situation. In this paper we analyze viable solutions to the new user problem in collaborative filtering (CF) that are based on the exploitation of user personality information: (a) personality-based CF, which directly improves the recommendation prediction model by incorporating user personality information, (b) personality-based active learning, which utilizes personality information for identifying additional useful preference data in the target recommendation domain to be elicited from the user, and (c) personality-based cross-domain recommendation, which exploits personality information to better use user preference data from auxiliary domains which can be used to compensate the lack of user preference data in the target domain. We benchmark the effectiveness of these methods on large datasets that span several domains, namely movies, music and books. Our results show that personality-aware methods achieve performance improvements that range from 6 to 94 % for users completely new to the system, while increasing the novelty of the recommended items by 3–40 % with respect to the non-personalized popularity baseline. We also discuss the limitations of our approach and the situations in which the proposed methods can be better applied, hence providing guidelines for researchers and practitioners in the field.},
note = {Pre SFI},
keywords = {},
pubstate = {published},
tppubtype = {article}
}
2015
Neil Rubens; Mehdi Elahi; Masashi Sugiyama; Dain Kaplan
Active learning in recommender systems Book Chapter
In: Ricci, Francesco; Rokach, Lior; Shapira, Bracha (Ed.): pp. 809-846, Springer, 2015, ISBN: 978-1-4899-7637-6, (Pre SFI).
@inbook{Rubens2015,
title = {Active learning in recommender systems},
author = {Neil Rubens and Mehdi Elahi and Masashi Sugiyama and Dain Kaplan},
editor = {Francesco Ricci and Lior Rokach and Bracha Shapira},
url = {https://link.springer.com/chapter/10.1007/978-1-4899-7637-6_24},
doi = {10.1007/978-1-4899-7637-6_24},
isbn = {978-1-4899-7637-6},
year = {2015},
date = {2015-01-01},
pages = {809-846},
publisher = {Springer},
abstract = {In Recommender Systems (RS), a user’s preferences are expressed in terms of rated items, where incorporating each rating may improve the RS’s predictive accuracy. In addition to a user rating items at-will (a passive process), RSs may also actively elicit the user to rate items, a process known as Active Learning (AL). However, the number of interactions between the RS and the user is still limited. One aim of AL is therefore the selection of items whose ratings are likely to provide the most information about the user’s preferences. In this chapter, we provide an overview of AL within RSs, discuss general objectives and considerations, and then summarize a variety of methods commonly employed. AL methods are categorized based on our interpretation of their primary motivation/goal, and then sub-classified into two commonly classified types, instance-based and model-based, for easier comprehension. We conclude the chapter by outlining ways in which AL methods could be evaluated, and provide a brief summary of methods performance.},
note = {Pre SFI},
keywords = {},
pubstate = {published},
tppubtype = {inbook}
}
0000
Himan Abdollahpouri; Mehdi Elahi; Masoud Mansoury; Shaghayegh Sahebi; Zahra Nazari; Allison Chaney; Babak Loni
MORS 2021: 1st Workshop on Multi-Objective Recommender Systems. Proceedings
0000.
@proceedings{Abdollahpouri2021,
title = {MORS 2021: 1st Workshop on Multi-Objective Recommender Systems.},
author = {Himan Abdollahpouri and Mehdi Elahi and Masoud Mansoury and Shaghayegh Sahebi and Zahra Nazari and Allison Chaney and Babak Loni},
journal = {In Fifteenth ACM Conference on Recommender Systems.},
pages = {787-788},
keywords = {},
pubstate = {published},
tppubtype = {proceedings}
}
/ Publications
2024
Rosnes, Daniel; Starke, Alain; Trattner, Christoph
ACM UMAP '24, 2024.
@conference{umap2024Daniel,
title = {Shaping the Future of Content-based News Recommenders: Insights from Evaluating Feature-Specific Similarity Metrics},
author = {Daniel Rosnes and Alain Starke and Christoph Trattner },
url = {https://mediafutures.no/umap2024/},
year = {2024},
date = {2024-07-01},
booktitle = {ACM UMAP '24},
abstract = {In news media, recommender system technology faces several domain-specific challenges. The continuous stream of new content and users deems content-based recommendation strategies, based on similar-item retrieval, to remain popular. However, a persistent challenge is to select relevant features and corresponding similarity functions, and whether this depends on the specific context. We evaluated feature-specific similarity metrics using human similarity judgments across national and local news domains. We performed an online experiment ($N = 141$) where we asked participants to judge the similarity between pairs of randomly sampled news articles. We had three contributions: (1) comparing novel metrics based on large language models to ones traditionally used in news recommendations, (2) exploring differences in similarity judgments across national and local news domains, and (3) examining which content-based strategies were perceived as appropriate in the news domain. Our results showed that one of the novel large language model based metrics (SBERT) was highly correlated with human judgments, while there were only small, most non-significant differences across national and local news domains. Finally, we found that while it may be possible to automatically recommend similar news using feature-specific metrics, their representativeness and appropriateness varied. We explain how our findings can guide the design of future content-based and hybrid recommender strategies in the news domain.},
keywords = {},
pubstate = {published},
tppubtype = {conference}
}
Jeng, Jia Hua; Kasangu, Gloria Anne Babile; Starke, Alain D.; Trattner, Christoph
Emotional Reframing of Economic News using a Large Language Model Conference
ACM UMAP 2024, 2024.
@conference{emorefram24,
title = {Emotional Reframing of Economic News using a Large Language Model},
author = {Jia Hua Jeng and Gloria Anne Babile Kasangu and Alain D. Starke and Christoph Trattner},
url = {https://mediafutures.no/umap2024___jeng_alain_gloria_christoph__workshop_-3/},
year = {2024},
date = {2024-07-01},
urldate = {2024-07-01},
booktitle = {ACM UMAP 2024},
abstract = {News media framing can shape public perception and potentially polarize views. Emotional language can exacerbate these framing effects, as a user’s emotional state can be an important contextual factor to use in news recommendation. Our research explores the relation between emotional framing techniques and the emotional states of readers, as well as readers’ perceived trust in specific news articles. Users (N = 200) had to read three economic news articles from the Washington Post. We used ChatGPT-4 to reframe news articles with specific emotional languages (Anger, Fear, Hope), compared to a neutral baseline reframed by a human journalist. Our results revealed that negative framing (Anger, Fear) elicited stronger negative emotional states among users than the neutral baseline, while Hope led to little changes overall. In contrast, perceived trust levels varied little across the different conditions. We discuss the implications of our findings and how emotional framing could affect societal polarization issues},
keywords = {},
pubstate = {published},
tppubtype = {conference}
}
Starke, Alain D.; Bremnes, Anders Sandvik; Knudsen, Erik; Trilling, Damian; Trattner, Christoph
ACM UMAP 2024, 2024.
@conference{percepvsreal24,
title = {Perception versus Reality: Evaluating User Awareness of Political Selective Exposure in News Recommender Systems},
author = {Alain D. Starke and Anders Sandvik Bremnes and Erik Knudsen and Damian Trilling and Christoph Trattner},
url = {https://mediafutures.no/umap2024___erik_alain_damian_anders_christoph/},
year = {2024},
date = {2024-07-01},
urldate = {2024-07-01},
booktitle = {ACM UMAP 2024},
abstract = {News Recommender Systems (NRSs) have become increasingly pivotal in shaping the news landscape, particularly in how news is disseminated. This has also led to concerns about information diversity, especially regarding selective exposure in the realm of political news. Users may not recognize that news content presented to them is subject to selective exposure, through users that incorporate political beliefs. Within the U.S. two-party system, our research explores the interactions between NRSs and users’ ability to discern news articles that align with their political biases. We performed an online experiment (N = 160) to address the issue of user awareness and self-recognition of selective exposure within NRSs. Users were asked to select any number of news articles that matched their political orientation (i.e., Democrat or Republican) from a list of 50 news articles (5 Democrat, 5 Republican, 40 filler articles), which were either ranked saliently towards their political orientation or randomly. Contrary to expectations, our findings reveal no significant difference in article selection between participants exposed to a baseline random order and those who where presented with the more salient and easy to select version. We did observe that Republicans performed worse than Democrats in identifying aligning articles, based on precision and recall metrics.},
keywords = {},
pubstate = {published},
tppubtype = {conference}
}
Mahmood, Bilal; Elahi, Mehdi; Touileb, Samia; Steskal, Lubos; Trattner, Christoph
Incorporating Editorial Feedback in the Evaluation of News Recommender Systems Conference
ACM UMAP 2024, 2024.
@conference{incoed24,
title = {Incorporating Editorial Feedback in the Evaluation of News Recommender Systems},
author = {Bilal Mahmood and Mehdi Elahi and Samia Touileb and Lubos Steskal and Christoph Trattner},
url = {https://mediafutures.no/lbr_umap_editorial_component_in_nrs/},
year = {2024},
date = {2024-07-01},
urldate = {2024-07-01},
booktitle = {ACM UMAP 2024},
abstract = {Research in the recommender systems field typically applies a rather traditional evaluation methodology when assessing the quality of recommendations. This methodology heavily relies on incorporating different forms of user feedback (e.g., clicks) representing the specific needs and interests of the users. While this methodology may offer various benefits, it may fail to comprehensively project the complexities of certain application domains, such as the news domain. This domain is distinct from other domains primarily due to the strong influence of editorial control in the news delivery process. Incorporation of this role can profoundly impact how the relevance of news articles is measured when recommended to the users. Despite its critical importance, there appears to be a research gap in investigating the dynamics between the roles of editorial control and personalization in the community of recommender systems. In this paper, we address this gap by conducting experiments where the relevance of recommendations is assessed from an editorial perspective. We received a real-world dataset from TV 2, one of the largest editor-managed commercial media houses in Norway, which includes editors’ feedback on how news articles are being related. In our experiment, we considered a scenario where algorithm-generated recommendations, using the K-Nearest Neighbor (KNN) model, employing various text embedding models to encode different sections of the news articles (e.g., title, lead title, body text, and full text), are compared against the editorial feedback. The results are promising, demonstrating the effectiveness of the recommendation in fulfilling the editorial prospects.},
keywords = {},
pubstate = {published},
tppubtype = {conference}
}
Klimashevskaia, Anastasiia; Jannach, Dietmar; Elahi, Mehdi; Trattner, Christoph
A Survey on Popularity Bias in Recommender Systems Journal Article Forthcoming
In: User Modeling and User-Adapted Interaction (UMUAI), Forthcoming.
@article{biasanas24,
title = {A Survey on Popularity Bias in Recommender Systems},
author = {Anastasiia Klimashevskaia and Dietmar Jannach and Mehdi Elahi and Christoph Trattner},
url = {https://mediafutures.no/popularitybias_literature_review-5/},
year = {2024},
date = {2024-06-13},
journal = {User Modeling and User-Adapted Interaction (UMUAI)},
abstract = {Recommender systems help people find relevant content in a personalized way. One main promise of such systems is that they are able to increase the visibility of items in the long tail, i.e., the lesser-
known items in a catalogue. Existing research, however, suggests that in many situations today’s recommendation algorithms instead exhibit a popularity bias, meaning that they often focus on rather popular items in their recommendations. Such a bias may not only lead to the limited value of the recommendations for consumers and providers in the short run, but it may also cause undesired reinforcement effects over time. In this paper, we discuss the potential reasons for popularity bias and review existing approaches to detect, quantify and mitigate popularity bias in
recommender systems. Our survey, therefore, includes both an overview
of the computational metrics used in the literature as well as a review of the main technical approaches to reduce the bias. Furthermore, we critically discuss today’s literature, where we observe that the research is almost entirely based on computational experiments and on certain
assumptions regarding the practical effects of including long-tail items in the recommendations.},
keywords = {},
pubstate = {forthcoming},
tppubtype = {article}
}
known items in a catalogue. Existing research, however, suggests that in many situations today’s recommendation algorithms instead exhibit a popularity bias, meaning that they often focus on rather popular items in their recommendations. Such a bias may not only lead to the limited value of the recommendations for consumers and providers in the short run, but it may also cause undesired reinforcement effects over time. In this paper, we discuss the potential reasons for popularity bias and review existing approaches to detect, quantify and mitigate popularity bias in
recommender systems. Our survey, therefore, includes both an overview
of the computational metrics used in the literature as well as a review of the main technical approaches to reduce the bias. Furthermore, we critically discuss today’s literature, where we observe that the research is almost entirely based on computational experiments and on certain
assumptions regarding the practical effects of including long-tail items in the recommendations.
Starke, Alain D.; Willemsen, Martijn C.
In: pp. 221–259, A Human-Centered Perspective of Intelligent Personalized Environments and Systems, 2024.
@inbook{Alain_pysch24,
title = {Psychologically Informed Design of Energy Recommender Systems: Are Nudges Still Effective in Tailored Choice Environments?},
author = {Alain D. Starke and Martijn C. Willemsen},
url = {https://mediafutures.no/starke2024-book-chapter-psych-informed-energy-recsys-4/},
year = {2024},
date = {2024-05-01},
urldate = {2024-05-01},
pages = {221–259},
edition = {A Human-Centered Perspective of Intelligent Personalized Environments and Systems},
series = {Human–Computer Interaction Series},
keywords = {},
pubstate = {published},
tppubtype = {inbook}
}
Pera, Maria Soledad; Cena, Federica; Landoni, Monica; Musto, Cataldo; Starke, Alain D.
Human Factors in User Modeling for Intelligent Systems Book Chapter
In: pp. 3–42, A Human-Centered Perspective of Intelligent Personalized Environments and Systems, 2024.
@inbook{Alain_humanF24,
title = {Human Factors in User Modeling for Intelligent Systems},
author = {Maria Soledad Pera and Federica Cena and Monica Landoni and Cataldo Musto and Alain D. Starke},
url = {https://mediafutures.no/pera2024-book-chapter-holistic-user-modeling/},
year = {2024},
date = {2024-05-01},
urldate = {2024-05-01},
pages = {3–42},
edition = {A Human-Centered Perspective of Intelligent Personalized Environments and Systems},
series = {Human–Computer Interaction Series.},
abstract = {In the current digital landscape, humans take center stage. This has caused a paradigm shift in the realm of intelligent technologies, prompting researchers and (industry) practitioners to reflect on the challenges and complexities involved in understanding the (potential) users of the technologies they develop. In this chapter, we provide an overview of human factors in user modeling, a core component of human-centered intelligent solutions. We discuss critical concepts, dimensions, and theories that inform the design of user models that are more attuned to human characteristics. Additionally, we emphasize the need for a comprehensive user model that simultaneously considers multiple factors to represent the intricacies of individuals’ interests and behaviors. Such a holistic model can, in turn, shape the design of intelligent solutions that are better able to adapt and cater to a wide range of user groups.},
keywords = {},
pubstate = {published},
tppubtype = {inbook}
}
2023
Knudsen, Erik; Starke, Alain D.; Trattner, Christoph
Association for Computing Machinery (ACM) RecSys ’23, 2023.
@conference{inra2023-1,
title = {Topical Preference Trumps Other Features in News Recommendation: A Conjoint Analysis on a Representative Sample from Norway},
author = {Erik Knudsen and Alain D. Starke and Christoph Trattner },
url = {https://mediafutures.no/inra2023-1/},
year = {2023},
date = {2023-09-18},
urldate = {2023-09-18},
booktitle = {Association for Computing Machinery (ACM) RecSys ’23},
keywords = {},
pubstate = {published},
tppubtype = {conference}
}
Seddik, Khadiga; Knudsen, Erik; Trilling, Damian; Trattner, Christoph
Understanding How News Recommender Systems Influence Selective Exposure Conference
Association for Computing Machinery (ACM) RecSys ’23, 2023.
@conference{behavrec2023,
title = {Understanding How News Recommender Systems Influence Selective Exposure},
author = {Khadiga Seddik and Erik Knudsen and Damian Trilling and Christoph Trattner },
url = {https://mediafutures.no/behavrec2023/},
year = {2023},
date = {2023-09-18},
urldate = {2023-09-18},
booktitle = {Association for Computing Machinery (ACM) RecSys ’23},
keywords = {},
pubstate = {published},
tppubtype = {conference}
}
Klimashevskaia, Anastasiia; Elahi, Mehdi; Jannach, Dietmar; Skjærven, Lars; Tessem, Astrid; Trattner, Christoph
Evaluating The Effects of Calibrated Popularity Bias Mitigation: A Field Study Conference
Association for Computing Machinery (ACM) RecSys ’23, 2023.
@conference{RecSys_2023_LBR,
title = {Evaluating The Effects of Calibrated Popularity Bias Mitigation: A Field Study},
author = {Anastasiia Klimashevskaia and Mehdi Elahi and Dietmar Jannach and Lars Skjærven and Astrid Tessem and Christoph Trattner},
url = {https://mediafutures.no/recsys_2023_lbr/},
year = {2023},
date = {2023-09-18},
booktitle = {Association for Computing Machinery (ACM) RecSys ’23},
keywords = {},
pubstate = {published},
tppubtype = {conference}
}
Majjodi, Ayoub El; Starke, Alain D.; Trattner, Christoph
Association for Computing Machinery (ACM) RecSys ’23, 2023.
@conference{inra2023,
title = {The Interplay between Food Knowledge, Nudges, and Preference Elicitation Methods Determines the Evaluation of a Recipe Recommender System},
author = {Ayoub El Majjodi and Alain D. Starke and Christoph Trattner },
url = {https://mediafutures.no/intrs2023-2/},
year = {2023},
date = {2023-09-18},
urldate = {2023-09-18},
booktitle = {Association for Computing Machinery (ACM) RecSys ’23},
keywords = {},
pubstate = {published},
tppubtype = {conference}
}
Starke, Alain; Emami, Kimia; Makarová, Andrea; Ferwerda, Bruce
Using Visual and Linguistic Framing to Support Sustainable Decisions in an Online Store Conference
Association for Computing Machinery (ACM) RecSys ’23,, 2023.
@conference{intrs23_session3,
title = {Using Visual and Linguistic Framing to Support Sustainable Decisions in an Online Store},
author = {Alain Starke and Kimia Emami and Andrea Makarová and Bruce Ferwerda },
url = {https://mediafutures.no/intrs23_session3_paper_2/},
year = {2023},
date = {2023-09-18},
urldate = {2023-09-18},
booktitle = {Association for Computing Machinery (ACM) RecSys ’23,},
keywords = {},
pubstate = {published},
tppubtype = {conference}
}
Jeng, Jia Hua; Starke, Alain D.; Trattner, Christoph
Towards Attitudinal Change in News Recommender Systems: A Pilot Study on Climate Change Workshop
2023.
@workshop{Jeng2023,
title = {Towards Attitudinal Change in News Recommender Systems: A Pilot Study on Climate Change},
author = {Jia Hua Jeng and Alain D. Starke and Christoph Trattner},
url = {https://mediafutures.no/jeng2023-towards-attitudinal-change-in-news2908-2/},
year = {2023},
date = {2023-04-18},
urldate = {2023-04-18},
abstract = {Personalized recommender systems facilitate decision-making in various domains by presenting content closely aligned with users’ preferences.
However, personalization can lead to unintended consequences. In news, selective information exposure and consumption might amplify
polarization, as users are empowered to seek out information that is in line with their own attitudes and viewpoints. However, personalization in
terms of algorithmic content and persuasive technology could also help to narrow the gap between polarized user attitudes and news consumption
patterns. This paper presents a pilot study on climate change news. We examined the relation between users’ level of environmental concern, their preferences
for news articles, and news article content. We aimed to capture a news article’s viewpoint through sentiment analysis. Users (N = 180)
were asked to read and evaluate 10 news articles from the Washington Post. We found a positive correlation between users’ level of environmental
concern and whether they liked the article. In contrast, no significant correlation was found between sentiment and environmental concern.
We argue why a different type of news article analysis than sentiment is needed. Finally, we present our research agenda on how persuasive technology
might help to support more exploration of news article viewpoints in the future.},
keywords = {},
pubstate = {published},
tppubtype = {workshop}
}
However, personalization can lead to unintended consequences. In news, selective information exposure and consumption might amplify
polarization, as users are empowered to seek out information that is in line with their own attitudes and viewpoints. However, personalization in
terms of algorithmic content and persuasive technology could also help to narrow the gap between polarized user attitudes and news consumption
patterns. This paper presents a pilot study on climate change news. We examined the relation between users’ level of environmental concern, their preferences
for news articles, and news article content. We aimed to capture a news article’s viewpoint through sentiment analysis. Users (N = 180)
were asked to read and evaluate 10 news articles from the Washington Post. We found a positive correlation between users’ level of environmental
concern and whether they liked the article. In contrast, no significant correlation was found between sentiment and environmental concern.
We argue why a different type of news article analysis than sentiment is needed. Finally, we present our research agenda on how persuasive technology
might help to support more exploration of news article viewpoints in the future.
2022
Majjodi, Ayoub El; Starke, Alain D.; Trattner, Christoph
Nudging Towards Health? Examining the Merits of Nutrition Labels and Personalization in a Recipe Recommender System, 2022.
@conference{Majjodi2022,
title = {Nudging Towards Health? Examining the Merits of Nutrition Labels and Personalization in a Recipe Recommender System},
author = { Ayoub El Majjodi and Alain D. Starke and Christoph Trattner
},
url = {https://dl.acm.org/doi/10.1145/3503252.3531312?fbclid=IwAR0eb6MPuISpVs9Vfkd-ww_KN7EjbMbiGdDQnPxjayogfKbHFgkSgeLdaxs},
year = {2022},
date = {2022-07-03},
urldate = {2022-07-03},
booktitle = {Nudging Towards Health? Examining the Merits of Nutrition Labels and Personalization in a Recipe Recommender System},
abstract = {Food recommender systems show personalized recipes to users based on content liked previously. Despite their potential, often recommended (popular) recipes in previous studies have turned out to be unhealthy, negatively contributing to prevalent obesity problems worldwide. Changing how foods are presented through digital nudges might help, but these are usually examined in non-personalized contexts, such as a brick-and-mortar supermarket. This study seeks to support healthy food choices in a personalized interface by adding front-of-package nutrition labels to recipes in a food recommender system. After performing an offline evaluation, we conducted an online study (N = 600) with six different recommender interfaces, based on a 2 (non-personalized vs. personalized recipe advice) x 3 (No Label, Multiple Traffic Light, Nutri-Score) between-subjects design. We found that recipe choices made in the non-personalized scenario were healthier, while the use of nutrition labels (our digital nudge) reduced choice difficulty when the content was personalized.},
keywords = {},
pubstate = {published},
tppubtype = {conference}
}
Elahi, Mehdi; Starke, Alain D.; Ioini, Nabil El; Lambrix, Anna Alexander; Trattner, Christoph
Developing and Evaluating a University Recommender System Journal Article
In: Frontiers in Artificial Intelligence , 2022.
@article{Elahi2022,
title = {Developing and Evaluating a University Recommender System},
author = {Mehdi Elahi and Alain D. Starke and Nabil El Ioini and Anna Alexander Lambrix and Christoph Trattner},
url = {https://www.frontiersin.org/articles/10.3389/frai.2021.796268/full},
doi = {https://doi.org/10.3389/frai.2021.796268},
year = {2022},
date = {2022-02-02},
journal = {Frontiers in Artificial Intelligence },
abstract = {A challenge for many young adults is to find the right institution to follow higher education. Global university rankings are a commonly used, but inefficient tool, for they do not consider a person's preferences and needs. For example, some persons pursue prestige in their higher education, while others prefer proximity. This paper develops and evaluates a university recommender system, eliciting user preferences as ratings to build predictive models and to generate personalized university ranking lists. In Study 1, we performed offline evaluation on a rating dataset to determine which recommender approaches had the highest predictive value. In Study 2, we selected three algorithms to produce different university recommendation lists in our online tool, asking our users to compare and evaluate them in terms of different metrics (Accuracy, Diversity, Perceived Personalization, Satisfaction, and Novelty). We show that a SVD algorithm scores high on accuracy and perceived personalization, while a KNN algorithm scores better on novelty. We also report findings on preferred university features.},
keywords = {},
pubstate = {published},
tppubtype = {article}
}
2021
Starke, Alain D.; Willemsen, Martijn C.; Trattner, Christoph
Nudging Healthy Choices in Food Search Through Visual Attractiveness Journal Article
In: no. April 2021, pp. 1-18, 2021.
@article{Starke2021,
title = {Nudging Healthy Choices in Food Search Through Visual Attractiveness},
author = {Alain D. Starke and Martijn C. Willemsen and Christoph Trattner},
url = {https://www.frontiersin.org/articles/10.3389/frai.2021.621743/full},
doi = {10.3389/frai.2021.621743},
year = {2021},
date = {2021-04-22},
number = {April 2021},
pages = {1-18},
abstract = {Recipe websites are becoming increasingly popular to support people in their home cooking. However, most of these websites prioritize popular recipes, which tend to be unhealthy. Drawing upon research on visual biases and nudges, this paper investigates whether healthy food choices can be supported in food search by depicting attractive images alongside recipes, as well as by re-ranking search results on health. After modelling the visual attractiveness of recipe images, we asked 239 users to search for specific online recipes and to select those they liked the most. Our analyses revealed that users tended to choose a healthier recipe if a visually attractive image was depicted alongside it, as well as if it was listed at the top of a list of search results. Even though less popular recipes were promoted this way, it did not come at the cost of a user’s level of satisfaction},
keywords = {},
pubstate = {published},
tppubtype = {article}
}
2020
Endriss, Ulle; de Haan, Ronald; Lang, Jerôme; Slavkovik, Marija
The complexity landscape of outcome determination in judgment aggregation Journal Article
In: Journal of Artificial Intelligence Research, vol. 69, pp. 687–731, 2020, (Pre SFI).
@article{Endriss2020,
title = {The complexity landscape of outcome determination in judgment aggregation},
author = {Ulle Endriss and Ronald de Haan and Jerôme Lang and Marija Slavkovik },
url = {https://www.jair.org/index.php/jair/article/view/11970/26619},
doi = {10.1613/jair.1.11970},
year = {2020},
date = {2020-11-04},
journal = {Journal of Artificial Intelligence Research},
volume = {69},
pages = {687–731},
abstract = {We provide a comprehensive analysis of the computational complexity of the outcome determinationproblem for the most important aggregation rules proposed in the literature on logic-based judgmentaggregation. Judgment aggregation is a powerful and flexible framework for studying problems ofcollective decision making that has attracted interest in a range of disciplines, including Legal Theory,Philosophy, Economics, Political Science, and Artificial Intelligence. The problem of computing theoutcome for a given list of individual judgments to be aggregated into a single collective judgment isthe most fundamental algorithmic challenge arising in this context. Our analysis applies to severaldifferent variants of the basic framework of judgment aggregation that have been discussed in theliterature, as well as to a new framework that encompasses all existing such frameworks in terms ofexpressive power and representational succinctness.},
note = {Pre SFI},
keywords = {},
pubstate = {published},
tppubtype = {article}
}
Hazrati, Naieme; Elahi, Mehdi
Addressing the New Item problem in video recommender systems by incorporation of visual features with restricted Boltzmann machines. Journal Article
In: Expert Systems, vol. e12645, pp. 1-20, 2020, (Pre SFI).
@article{Hazrati2020,
title = { Addressing the New Item problem in video recommender systems by incorporation of visual features with restricted Boltzmann machines.},
author = {Naieme Hazrati and Mehdi Elahi},
url = {https://onlinelibrary.wiley.com/doi/epdf/10.1111/exsy.12645},
doi = {https://doi.org/10.1111/exsy.12645},
year = {2020},
date = {2020-10-19},
journal = {Expert Systems},
volume = {e12645},
pages = {1-20},
abstract = {Over the past years, the research of video recommender systems (RSs) has been mainly focussed on the development of novel algorithms. Although beneficial, still any algorithm may fail to recommend video items that the system has no form of data associated to them (New Item Cold Start). This problem occurs when a new item is added to the catalogue of the system and no data are available for that item. In content‐based RSs, the video items are typically represented by semantic attributes, when generating recommendations. These attributes require a group of experts or users for annotation, and still, the generated recommendations might not capture a complete picture of the users' preferences, for example, the visual tastes of users on video style. This article addresses this problem by proposing recommendation based on novel visual features that do not require human annotation and can represent visual aspects of video items. We have designed a novel evaluation methodology considering three realistic scenarios, that is, (a) extreme cold start, (b) moderate cold start and (c) warm‐start scenario. We have conducted a set of comprehensive experiments, and our results have shown the superior performance of recommendations based on visual features, in all of the evaluation scenarios.},
note = {Pre SFI},
keywords = {},
pubstate = {published},
tppubtype = {article}
}
Balog, Krisztian; Radlinski, Filip
Measuring Recommendation Explanation Quality: The Conflicting Goals of Explanations Conference
Proceedings of the 43rd International ACM SIGIR Conference on Research and Development in Information Retrieval (SIGIR '20), New York, 2020, (Pre SFI).
@conference{Balog2020,
title = {Measuring Recommendation Explanation Quality: The Conflicting Goals of Explanations},
author = {Krisztian Balog and Filip Radlinski},
url = {https://dl.acm.org/doi/pdf/10.1145/3397271.3401032},
doi = {https://doi.org/10.1145/3397271.3401032},
year = {2020},
date = {2020-07-01},
booktitle = {Proceedings of the 43rd International ACM SIGIR Conference on Research and Development in Information Retrieval (SIGIR '20)},
pages = {329–338},
address = {New York},
abstract = {Explanations have a large effect on how people respond to recommendations. However, there are many possible intentions a system may have in generating explanations for a given recommendation -from increasing transparency, to enabling a faster decision, to persuading the recipient. As a good explanation for one goal may not be good for others, we address the questions of (1) how to robustly measure if an explanation meets a given goal and (2) how the different goals interact with each other. Specifically, this paper presents a first proposal of how to measure the quality of explanations along seven common goal dimensions catalogued in the literature. We find that the seven goals are not independent, but rather exhibit strong structure. Proposing two novel explanation evaluation designs, we identify challenges in evaluation, and provide more efficient measurement approaches of explanation quality.},
note = {Pre SFI},
keywords = {},
pubstate = {published},
tppubtype = {conference}
}
Soe, Than Htut; Nordberg, Oda Elise; Guribye, Frode; Slavkovik, Marija
Circumvention by design - dark patterns in cookie consents for online news outlets Conference
Proceedings of the 11th Nordic Conference on Human-Computer Interaction, 2020, (Pre SFI).
@conference{Soe2020,
title = {Circumvention by design - dark patterns in cookie consents for online news outlets},
author = {Than Htut Soe and Oda Elise Nordberg and Frode Guribye and Marija Slavkovik},
url = {https://dl.acm.org/doi/pdf/10.1145/3419249.3420132},
doi = {10.1145/3419249.3420132},
year = {2020},
date = {2020-06-24},
booktitle = {Proceedings of the 11th Nordic Conference on Human-Computer Interaction},
abstract = {To ensure that users of online services understand what data are collected and how they are used in algorithmic decision-making, the European Union's General Data Protection Regulation (GDPR) specifies informed consent as a minimal requirement. For online news outlets consent is commonly elicited through interface design elements in the form of a pop-up. We have manually analyzed 300 data collection consent notices from news outlets that are built to ensure compliance with GDPR. The analysis uncovered a variety of strategies or dark patterns that circumvent the intent of GDPR by design. We further study the presence and variety of these dark patterns in these "cookie consents" and use our observations to specify the concept of dark pattern in the context of consent elicitation.},
note = {Pre SFI},
keywords = {},
pubstate = {published},
tppubtype = {conference}
}
Nunes, Ingrid; Jannach, Dietmar
A Systematic Review and Taxonomy of Explanations in Decision Support and Recommender Systems Journal Article
In: User-Modeling and User-Adapted Interaction, vol. 27, no. 3-5, pp. 393-444, 2020, (Pre SFI).
@article{Nunes2020,
title = {A Systematic Review and Taxonomy of Explanations in Decision Support and Recommender Systems},
author = {Ingrid Nunes and Dietmar Jannach},
url = {https://arxiv.org/pdf/2006.08672.pdf},
doi = {10.1007/s11257-017-9195-0},
year = {2020},
date = {2020-06-15},
journal = {User-Modeling and User-Adapted Interaction},
volume = {27},
number = {3-5},
pages = {393-444},
abstract = {With the recent advances in the field of artificial intelligence, an increasing number of decision-making tasks are delegated to software systems. A key requirement for the success and adoption of such systems is that users must trust system choices or even fully automated decisions. To achieve this, explanation facilities have been widely investigated as a means of establishing trust in these systems since the early years of expert systems. With today's increasingly sophisticated machine learning algorithms, new challenges in the context of explanations, accountability, and trust towards such systems constantly arise. In this work, we systematically review the literature on explanations in advice-giving systems. This is a family of systems that includes recommender systems, which is one of the most successful classes of advice-giving software in practice. We investigate the purposes of explanations as well as how they are generated, presented to users, and evaluated. As a result, we derive a novel comprehensive taxonomy of aspects to be considered when designing explanation facilities for current and future decision support systems. The taxonomy includes a variety of different facets, such as explanation objective, responsiveness, content and presentation. Moreover, we identified several challenges that remain unaddressed so far, for example related to fine-grained issues associated with the presentation of explanations and how explanation facilities are evaluated.},
note = {Pre SFI},
keywords = {},
pubstate = {published},
tppubtype = {article}
}
Sjøvaag, Helle; Owren, Thomas; Borgen, Turid
Strategic and Organisational fit in Corporate News Markets: A Principal-agent Approach to Studying Newspaper Mergers Journal Article
In: Journalism Practice, pp. 1-18, 2020, (Pre SFI).
@article{Sjøvaag2020,
title = {Strategic and Organisational fit in Corporate News Markets: A Principal-agent Approach to Studying Newspaper Mergers},
author = {Helle Sjøvaag and Thomas Owren and Turid Borgen},
url = {https://www.tandfonline.com/doi/epub/10.1080/17512786.2020.1772097?needAccess=true},
doi = {https://doi.org/10.1080/17512786.2020.1772097},
year = {2020},
date = {2020-05-20},
journal = {Journalism Practice},
pages = {1-18},
abstract = {This article analyses strategic and organisational fit in corporate newspaper mergers in the context of the digitalisation of local newspaper markets. Using the 2019 acquisition of Nordsjø Media by Amedia in Norway as case, we analyse how eight editors-in-chief perceive the process of incorporating small, low-frequency, print-oriented monopolistic newspapers into one of Scandinavia’s largest newspaper chains. The semi-structured interviews were analysed in light of perceived strategic and organisational fit in a principal-agent theoretical framework, the aim of which is to shed light on corporate ownership effects in consolidated newspaper markets. The analysis reveals the precarity of independent ownership in digitising news markets, to which corporatisation emerges as a necessary and welcomed solution. We find the strategic fit as perceived by editors to be tied to technological resources and scale economics, while organisational fit is hampered by the speed and pressure of corporatisation processes. While these results largely support findings from previous acquisition studies in the news industries, the contribution of this analysis lies primarily with the necessity of scale required by the technological transformation that forces independent newspapers to submit to larger chain operations and how it influences considerations of fit in disruptive digital news markets.},
note = {Pre SFI},
keywords = {},
pubstate = {published},
tppubtype = {article}
}
Zhang, Shuo; Balog, Krisztian
Web Table Extraction, Retrieval, and Augmentation: A Survey Journal Article
In: ACM Transactions on Intelligent Systems and Technology (TIST), vol. 11, no. 2, pp. 1-35, 2020, (Pre SFI).
@article{Zhang2020,
title = {Web Table Extraction, Retrieval, and Augmentation: A Survey},
author = {Shuo Zhang and Krisztian Balog},
url = {https://arxiv.org/pdf/2002.00207.pdf},
year = {2020},
date = {2020-02-01},
journal = {ACM Transactions on Intelligent Systems and Technology (TIST)},
volume = {11},
number = {2},
pages = {1-35},
abstract = {Tables are a powerful and popular tool for organizing and manipulating data. A vast number of tables can be found on the Web, which represents a valuable knowledge resource. The objective of this survey is to synthesize and present two decades of research on web tables. In particular, we organize existing literature into six main categories of information access tasks: table extraction, table interpretation, table search, question answering, knowledge base augmentation, and table augmentation. For each of these tasks, we identify and describe seminal approaches, present relevant resources, and point out interdependencies among the different tasks.},
note = {Pre SFI},
keywords = {},
pubstate = {published},
tppubtype = {article}
}
2019
Dacrema, Maurizio Ferrari; Cremonesi, Paolo; Jannach, Dietmar
Are We Really Making Much Progress? A Worrying Analysis of Recent Neural Recommendation Approaches Conference
Proceedings of the 2019 ACM Conference on Recommender Systems (RecSys 2019), Copenhagen, 2019, (Pre SFI).
@conference{Dacrema2019,
title = {Are We Really Making Much Progress? A Worrying Analysis of Recent Neural Recommendation Approaches},
author = {Maurizio Ferrari Dacrema and Paolo Cremonesi and Dietmar Jannach},
url = {https://arxiv.org/pdf/1907.06902.pdf},
year = {2019},
date = {2019-08-16},
booktitle = {Proceedings of the 2019 ACM Conference on Recommender Systems (RecSys 2019)},
address = {Copenhagen},
abstract = {Deep learning techniques have become the method of choice for
researchers working on algorithmic aspects of recommender systems. With the strongly increased interest in machine learning in
general, it has, as a result, become difficult to keep track of what
represents the state-of-the-art at the moment, e.g., for top-n recommendation tasks. At the same time, several recent publications
point out problems in today’s research practice in applied machine
learning, e.g., in terms of the reproducibility of the results or the
choice of the baselines when proposing new models.
In this work, we report the results of a systematic analysis of algorithmic proposals for top-n recommendation tasks. Specifically,
we considered 18 algorithms that were presented at top-level research conferences in the last years. Only 7 of them could be reproduced with reasonable effort. For these methods, it however
turned out that 6 of them can often be outperformed with comparably simple heuristic methods, e.g., based on nearest-neighbor or
graph-based techniques. The remaining one clearly outperformed
the baselines but did not consistently outperform a well-tuned nonneural linear ranking method. Overall, our work sheds light on a
number of potential problems in today’s machine learning scholarship and calls for improved scientific practices in this area.},
note = {Pre SFI},
keywords = {},
pubstate = {published},
tppubtype = {conference}
}
researchers working on algorithmic aspects of recommender systems. With the strongly increased interest in machine learning in
general, it has, as a result, become difficult to keep track of what
represents the state-of-the-art at the moment, e.g., for top-n recommendation tasks. At the same time, several recent publications
point out problems in today’s research practice in applied machine
learning, e.g., in terms of the reproducibility of the results or the
choice of the baselines when proposing new models.
In this work, we report the results of a systematic analysis of algorithmic proposals for top-n recommendation tasks. Specifically,
we considered 18 algorithms that were presented at top-level research conferences in the last years. Only 7 of them could be reproduced with reasonable effort. For these methods, it however
turned out that 6 of them can often be outperformed with comparably simple heuristic methods, e.g., based on nearest-neighbor or
graph-based techniques. The remaining one clearly outperformed
the baselines but did not consistently outperform a well-tuned nonneural linear ranking method. Overall, our work sheds light on a
number of potential problems in today’s machine learning scholarship and calls for improved scientific practices in this area.
de Haan, Ronald; Slavkovik, Marija
Answer set programming for judgment aggregation Conference
Proceedings of the Twenty-Eighth International Joint Conference on Artificial Intelligence, International Joint Conferences on Artificial Intelligence, 2019, (Pre SFI).
@conference{deHaan2019,
title = {Answer set programming for judgment aggregation},
author = {Ronald de Haan and Marija Slavkovik},
url = {https://www.ijcai.org/Proceedings/2019/0231.pdf},
year = {2019},
date = {2019-08-10},
booktitle = {Proceedings of the Twenty-Eighth International Joint Conference on Artificial Intelligence},
pages = {1668-1674},
publisher = {International Joint Conferences on Artificial Intelligence},
abstract = {Judgment aggregation (JA) studies how to aggregate truth valuations on logically related issues. Computing the outcome of aggregation procedures is notoriously computationally hard, which is the likely reason that no implementation of them exists as of yet. However, even hard problems sometimes need to be solved. The worst-case computational complexity of answer set programming (ASP) matches that of most problems in judgment aggregation. We take advantage of this and propose a natural and modular encoding of various judgment aggregation procedures and related problems in JA into ASP. With these encodings, we achieve two results: (1) paving the way towards constructing a wide range of new benchmark instances (from JA) for answer set solving algorithms; and (2) providing an automated tool for researchers in the area of judgment aggregation.},
note = {Pre SFI},
keywords = {},
pubstate = {published},
tppubtype = {conference}
}
Balog, Krisztian; Radlinski, Filip; Arakelyan, Shushan
Transparent, Scrutable and Explainable User Models for Personalized Recommendation Conference
Proceedings of the 42nd International ACM SIGIR Conference on Research and Development in Information Retrieval (SIGIR’19), 2019, (Pre SFI).
@conference{Balog2019,
title = {Transparent, Scrutable and Explainable User Models for Personalized Recommendation},
author = {Krisztian Balog and Filip Radlinski and Shushan Arakelyan},
url = {https://dl.acm.org/doi/pdf/10.1145/3331184.3331211},
doi = {https://doi.org/10.1145/3331184.3331211},
year = {2019},
date = {2019-07-25},
booktitle = {Proceedings of the 42nd International ACM SIGIR Conference on Research and Development in Information Retrieval (SIGIR’19)},
pages = {265–274},
abstract = {Most recommender systems base their recommendations on implicit or explicit item-level feedback provided by users. These item ratings are combined into a complex user model, which then predicts the suitability of other items. While effective, such methods have limited scrutability and transparency. For instance, if a user's interests change, then many item ratings would usually need to be modified to significantly shift the user's recommendations. Similarly, explaining how the system characterizes the user is impossible, short of presenting the entire list of known item ratings. In this paper, we present a new set-based recommendation technique that permits the user model to be explicitly presented to users in natural language, empowering users to understand recommendations made and improve the recommendations dynamically. While performing comparably to traditional collaborative filtering techniques in a standard static setting, our approach allows users to efficiently improve recommendations. Further, it makes it easier for the model to be validated and adjusted, building user trust and understanding.},
note = {Pre SFI},
keywords = {},
pubstate = {published},
tppubtype = {conference}
}
Sjøvaag, Helle
Journalism between the state and the market Book
Routledge, New York, 2019, ISBN: 9781138543348, (Pre SFI).
@book{Sjøvaag2019,
title = {Journalism between the state and the market},
author = {Helle Sjøvaag},
url = {https://www.researchgate.net/publication/332449932_Journalism_Between_the_State_and_the_Market},
isbn = {9781138543348},
year = {2019},
date = {2019-04-24},
publisher = {Routledge},
address = {New York},
abstract = {Using the Nordic media model as an empirical backdrop, Journalism Between the State and the Market defines and analyzes journalism’s fundamental problem: its shifting location between the state and the market.
This book examines how this distance is decreasing as journalism steps closer to both the market (algorithmically monetizing audiences) and the state (lobbying governments for subsidies and attacking public service broadcasting). The book analyzes journalism’s negotiated position between the market and the state in the age of disruptions, offering a theoretical foundation that seeks to account for the structural conditions of journalism in the digital age.
For scholars, graduates and students in journalism, news sociology and media and communication studies, Journalism Between the State and the Market provides a theoretical perspective that can be used as a valuable tool when studying and observing the current developments in journalism.},
note = {Pre SFI},
keywords = {},
pubstate = {published},
tppubtype = {book}
}
This book examines how this distance is decreasing as journalism steps closer to both the market (algorithmically monetizing audiences) and the state (lobbying governments for subsidies and attacking public service broadcasting). The book analyzes journalism’s negotiated position between the market and the state in the age of disruptions, offering a theoretical foundation that seeks to account for the structural conditions of journalism in the digital age.
For scholars, graduates and students in journalism, news sociology and media and communication studies, Journalism Between the State and the Market provides a theoretical perspective that can be used as a valuable tool when studying and observing the current developments in journalism.
Liao, Beishui; Slavkovik, Marija; van der Torre, Leendert
Building Jiminy Cricket: An architecture for moral agreements among stakeholders Conference
Proceedings of the 2019 AAAI/ACM Conference on AI, Ethics, and Society, 2019, (Pre SFI).
@conference{Liao2019,
title = {Building Jiminy Cricket: An architecture for moral agreements among stakeholders},
author = {Beishui Liao and Marija Slavkovik and Leendert van der Torre},
url = {https://arxiv.org/pdf/1812.04741.pdf},
year = {2019},
date = {2019-03-07},
booktitle = {Proceedings of the 2019 AAAI/ACM Conference on AI, Ethics, and Society},
pages = {147–153},
abstract = {An autonomous system is constructed by a manufacturer, operates in a society subject to norms and laws, and is interacting with end-users. We address the
challenge of how the moral values and views of all stakeholders can be integrated
and reflected in the moral behaviour of the autonomous system. We propose an artificial moral agent architecture that uses techniques from normative systems and
formal argumentation to reach moral agreements among stakeholders. We show
how our architecture can be used not only for ethical practical reasoning and collaborative decision-making, but also for the explanation of such moral behavior.},
note = {Pre SFI},
keywords = {},
pubstate = {published},
tppubtype = {conference}
}
challenge of how the moral values and views of all stakeholders can be integrated
and reflected in the moral behaviour of the autonomous system. We propose an artificial moral agent architecture that uses techniques from normative systems and
formal argumentation to reach moral agreements among stakeholders. We show
how our architecture can be used not only for ethical practical reasoning and collaborative decision-making, but also for the explanation of such moral behavior.
2018
Sjøvaag, Helle; Pedersen, Truls André; Owren, Thomas
Is public service broadcasting a threat to commercial media? Journal Article
In: Media, Culture & Society, vol. 46, no. 1, pp. 808-827, 2018, (Pre SFI).
@article{Sjøvaag2018c,
title = {Is public service broadcasting a threat to commercial media?},
author = {Helle Sjøvaag and Truls André Pedersen and Thomas Owren},
url = {https://journals.sagepub.com/doi/pdf/10.1177/0163443718818354},
doi = {https://doi.org/10.1177/0163443718818354},
year = {2018},
date = {2018-12-20},
journal = {Media, Culture & Society},
volume = {46},
number = {1},
pages = {808-827},
abstract = {This article asks to what extent public service broadcasting’s online news service resembles that of commercial media. The context of this inquiry is claims of ‘out-crowding’ facing public service broadcasters across Europe. In Norway, commercial players in this debate accuse the public service broadcaster, NRK, of being too similar to competitors in the private sector for commercial operators to attain sustainable revenues in the online realm. To ascertain the extent to what these claims are warranted, this article compares NRK’s online content with that of nine commercial competitors in national and local markets, using a hybrid methodological approach combining quantitative content analysis with Latent Dirichlet allocation, analysing in excess of 115,000 documents. Findings show that commercial operators resemble each other more than they do NRK, indicating closer competition in the commercial segment than between the public service broadcaster and market players.},
note = {Pre SFI},
keywords = {},
pubstate = {published},
tppubtype = {article}
}
Dyrkolbotn, Sjur; Pedersen, Truls; Slavkovik, Marija
On the distinction between implicit and explicit ethical agency Conference
Proceedings of the 2018 AAAI/ACM Conference on AI, Ethics, and Society (AIES '18), 2018, (Pre SFI).
@conference{Dyrkolbotn2018,
title = {On the distinction between implicit and explicit ethical agency},
author = {Sjur Dyrkolbotn and Truls Pedersen and Marija Slavkovik},
url = {https://dl.acm.org/doi/pdf/10.1145/3278721.3278769},
doi = {https://doi.org/10.1145/3278721.3278769},
year = {2018},
date = {2018-12-01},
booktitle = {Proceedings of the 2018 AAAI/ACM Conference on AI, Ethics, and Society (AIES '18)},
pages = {74–80},
abstract = {With recent advances in artificial intelligence and the rapidly increasing importance of autonomous intelligent systems in society, it is becoming clear that artificial agents will have to be designed to comply with complex ethical standards. As we work to develop moral machines, we also push the boundaries of existing legal categories. The most pressing question is what kind of ethical decision-making our machines are actually able to engage in. Both in law and in ethics, the concept of agency forms a basis for further legal and ethical categorisations, pertaining to decision-making ability. Hence, without a cross-disciplinary understanding of what we mean by ethical agency in machines, the question of responsibility and liability cannot be clearly addressed. Here we make first steps towards a comprehensive definition, by suggesting ways to distinguish between implicit and explicit forms of ethical agency.},
note = {Pre SFI},
keywords = {},
pubstate = {published},
tppubtype = {conference}
}
Sjøvaag, Helle; Pedersen, Truls André
Female voices in the news: Structural conditions of gender representations in Norwegian newspapers Journal Article
In: Journalism & Mass Communication Quarterly, vol. 96, no. 1, pp. 215-238, 2018, (Pre SFI).
@article{Sjøvaag2018b,
title = {Female voices in the news: Structural conditions of gender representations in Norwegian newspapers},
author = {Helle Sjøvaag and Truls André Pedersen},
url = {https://journals.sagepub.com/doi/pdf/10.1177/1077699018789885},
doi = {https://doi.org/10.1177/1077699018789885},
year = {2018},
date = {2018-08-21},
journal = {Journalism & Mass Communication Quarterly},
volume = {96},
number = {1},
pages = {215-238},
abstract = {The article presents a hybrid analysis combining manual content analysis of 9,131 sources in 5,544 news stories across 75 publications, with computational gender recognition producing 551,102 names from 320,228 articles across 125 newspapers. The article investigates the significance of structural features for the presence of women in the news. Results show female sources are only equal to men as ordinary citizens and children, and only in lifestyle content. Among the structural features examined, only local distribution and a circulation less than 5,000 exhibit improvements in female representation. Ownership, distribution frequency, market position, and direct press support had little or no effect.},
note = {Pre SFI},
keywords = {},
pubstate = {published},
tppubtype = {article}
}
Sjøvaag, Helle; Stavelin, Eirik; Karlsson, Michael; Kammer, Aske
The hyperlinked Scandinavian news ecology: The unequal terms forged by the structural properties of digitalisation Journal Article
In: Digital Journalism, vol. 7, no. 4, pp. 507-531, 2018, (Pre SFI).
@article{Sjøvaag2018,
title = {The hyperlinked Scandinavian news ecology: The unequal terms forged by the structural properties of digitalisation},
author = {Helle Sjøvaag and Eirik Stavelin and Michael Karlsson and Aske Kammer},
url = {https://www.tandfonline.com/doi/epub/10.1080/21670811.2018.1454335?needAccess=true},
doi = {https://doi.org/10.1080/21670811.2018.1454335},
year = {2018},
date = {2018-04-06},
journal = {Digital Journalism},
volume = {7},
number = {4},
pages = {507-531},
abstract = {The article presents a network analysis of 22,861,013 geocoded external hyperlinks, collected from 230 Danish, 220 Norwegian and 208 Swedish news websites in 2016. The analysis asks what the structural properties of the Scandinavian media systems—including its geography and ownership structures—mean for news outlets’ centrality within the hyperlinked news ecology. The analysis finds that whereas incumbent legacy media occupy central positions, about one third of the network is absent from the hyperlinked interaction, primarily local, independently owned newspapers. A multiple linear regression analysis shows that national distribution and corporate ownership correlates to network centrality more than other predictors. As brokers in the network consist of the large, legacy, capital-based news organisations, hyperlink connectivity is primarily characterised by proximity to the centres of power, corporate ownership, agenda setting incumbency and national distribution.},
note = {Pre SFI},
keywords = {},
pubstate = {published},
tppubtype = {article}
}
Ludewig, Malte; Jannach, Dietmar
Evaluation of Session-based Recommendation Algorithms Journal Article
In: User-Modeling and User-Adapted Interaction, vol. 28, no. 4-5, pp. 331-390, 2018, (Pre SFI).
@article{Ludewig2018,
title = {Evaluation of Session-based Recommendation Algorithms},
author = {Malte Ludewig and Dietmar Jannach},
url = {https://arxiv.org/pdf/1803.09587.pdf},
doi = {10.1007/s11257-018-9209-6},
year = {2018},
date = {2018-03-26},
journal = {User-Modeling and User-Adapted Interaction},
volume = {28},
number = {4-5},
pages = {331-390},
abstract = {Recommender systems help users find relevant items of interest, for example on e-commerce or media streaming sites. Most academic research is concerned with approaches that personalize the recommendations according to long-term user profiles. In many real-world applications, however, such long-term profiles often do not exist and recommendations therefore have to be made solely based on the observed behavior of a user during an ongoing session. Given the high practical relevance of the problem, an increased interest in this problem can be observed in recent years, leading to a number of proposals for session-based recommendation algorithms that typically aim to predict the user's immediate next actions. In this work, we present the results of an in-depth performance comparison of a number of such algorithms, using a variety of datasets and evaluation measures. Our comparison includes the most recent approaches based on recurrent neural networks like GRU4REC, factorized Markov model approaches such as FISM or FOSSIL, as well as simpler methods based, e.g., on nearest neighbor schemes. Our experiments reveal that algorithms of this latter class, despite their sometimes almost trivial nature, often perform equally well or significantly better than today's more complex approaches based on deep neural networks. Our results therefore suggest that there is substantial room for improvement regarding the development of more sophisticated session-based recommendation algorithms.},
note = {Pre SFI},
keywords = {},
pubstate = {published},
tppubtype = {article}
}
Quadrana, Massimo; Cremonesi, Paolo; Jannach, Dietmar
Sequence-Aware Recommender Systems Journal Article
In: ACM Computing Surveys, vol. 51, no. 4, pp. 1-35, 2018, (Pre SFI).
@article{Quadrana2018,
title = {Sequence-Aware Recommender Systems},
author = {Massimo Quadrana and Paolo Cremonesi and Dietmar Jannach},
url = {https://arxiv.org/pdf/1802.08452.pdf},
year = {2018},
date = {2018-02-23},
journal = {ACM Computing Surveys},
volume = {51},
number = {4},
pages = {1-35},
abstract = {Recommender systems are one of the most successful applications of data mining and machine learning technology in practice. Academic research in the field is historically often based on the matrix completion problem formulation, where for each user-item-pair only one interaction (e.g., a rating) is considered. In many application domains, however, multiple user-item interactions of different types can be recorded over time. And, a number of recent works have shown that this information can be used to build richer individual user models and to discover additional behavioral patterns that can be leveraged in the recommendation process. In this work we review existing works that consider information from such sequentially-ordered user- item interaction logs in the recommendation process. Based on this review, we propose a categorization of the corresponding recommendation tasks and goals, summarize existing algorithmic solutions, discuss methodological approaches when benchmarking what we call sequence-aware recommender systems, and outline open challenges in the area.},
note = {Pre SFI},
keywords = {},
pubstate = {published},
tppubtype = {article}
}
Zhang, Shuo; Balog, Krisztian
Ad Hoc Table Retrieval using Semantic Similarity Conference
Proceedings of The Web Conference 2018 (WWW’18), 2018, (Pre SFI).
@conference{Zhang2018,
title = {Ad Hoc Table Retrieval using Semantic Similarity},
author = {Shuo Zhang and Krisztian Balog},
url = {https://arxiv.org/pdf/1802.06159.pdf},
doi = {10.1145/3178876.3186067},
year = {2018},
date = {2018-02-16},
booktitle = {Proceedings of The Web Conference 2018 (WWW’18)},
pages = {1553-1562},
abstract = {We introduce and address the problem of ad hoc table retrieval: answering a keyword query with a ranked list of tables. This task is not only interesting on its own account, but is also being used as a core component in many other table-based information access scenarios, such as table completion or table mining. The main novel contribution of this work is a method for performing semantic matching between queries and tables. Specifically, we (i) represent queries and tables in multiple semantic spaces (both discrete sparse and continuous dense vector representations) and (ii) introduce various similarity measures for matching those semantic representations. We consider all possible combinations of semantic representations and similarity measures and use these as features in a supervised learning model. Using a purpose-built test collection based on Wikipedia tables, we demonstrate significant and substantial improvements over a state-of-the-art baseline.},
note = {Pre SFI},
keywords = {},
pubstate = {published},
tppubtype = {conference}
}
Balog, Krisztian
1, Springer, 2018, ISBN: 978-3-319-93935-3, (Pre SFI).
@book{Balog2018,
title = {Entity-Oriented Search},
author = {Krisztian Balog},
url = {https://www.springer.com/gp/book/9783319939339},
doi = {10.1007/978-3-319-93935-3},
isbn = {978-3-319-93935-3},
year = {2018},
date = {2018-01-01},
volume = {39},
publisher = {Springer},
edition = {1},
abstract = {This open access book covers all facets of entity-oriented search—where “search” can be interpreted in the broadest sense of information access—from a unified point of view, and provides a coherent and comprehensive overview of the state of the art. It represents the first synthesis of research in this broad and rapidly developing area. Selected topics are discussed in-depth, the goal being to establish fundamental techniques and methods as a basis for future research and development. Additional topics are treated at a survey level only, containing numerous pointers to the relevant literature. A roadmap for future research, based on open issues and challenges identified along the way, rounds out the book.},
note = {Pre SFI},
keywords = {},
pubstate = {published},
tppubtype = {book}
}
2017
Elahi, Mehdi; Deldjoo, Yashar; Moghaddam, Farshad Bakhshandegan; Cella, Leonardo; Cerada, Stefano; Cremonesi, Paolo
Exploring the semantic gap for movie recommendations Conference
Proceedings of the Eleventh ACM Conference on Recommender Systems, Association for Computing Machinery New York, 2017, (Pre SFI).
@conference{Elahi2017,
title = {Exploring the semantic gap for movie recommendations},
author = {Mehdi Elahi and Yashar Deldjoo and Farshad Bakhshandegan Moghaddam and Leonardo Cella and Stefano Cerada and Paolo Cremonesi },
url = {https://dl.acm.org/doi/pdf/10.1145/3109859.3109908},
doi = {https://doi.org/10.1145/3109859.3109908},
year = {2017},
date = {2017-08-01},
booktitle = {Proceedings of the Eleventh ACM Conference on Recommender Systems},
pages = {326–330},
address = {New York},
organization = {Association for Computing Machinery},
abstract = {In the last years, there has been much attention given to the semantic gap problem in multimedia retrieval systems. Much effort has been devoted to bridge this gap by building tools for the extraction of high-level, semantics-based features from multimedia content, as low-level features are not considered useful because they deal primarily with representing the perceived content rather than the semantics of it.
In this paper, we explore a different point of view by leveraging the gap between low-level and high-level features. We experiment with a recent approach for movie recommendation that extract low-level Mise-en-Scéne features from multimedia content and combine it with high-level features provided by the wisdom of the crowd.
To this end, we first performed an offline performance assessment by implementing a pure content-based recommender system with three different versions of the same algorithm, respectively based on (i) conventional movie attributes, (ii) mise-en-scene features, and (iii) a hybrid method that interleaves recommendations based on movie attributes and mise-en-scene features. In a second study, we designed an empirical study involving 100 subjects and collected data regarding the quality perceived by the users. Results from both studies show that the introduction of mise-en-scéne features in conjunction with traditional movie attributes improves both offline and online quality of recommendations.},
note = {Pre SFI},
keywords = {},
pubstate = {published},
tppubtype = {conference}
}
In this paper, we explore a different point of view by leveraging the gap between low-level and high-level features. We experiment with a recent approach for movie recommendation that extract low-level Mise-en-Scéne features from multimedia content and combine it with high-level features provided by the wisdom of the crowd.
To this end, we first performed an offline performance assessment by implementing a pure content-based recommender system with three different versions of the same algorithm, respectively based on (i) conventional movie attributes, (ii) mise-en-scene features, and (iii) a hybrid method that interleaves recommendations based on movie attributes and mise-en-scene features. In a second study, we designed an empirical study involving 100 subjects and collected data regarding the quality perceived by the users. Results from both studies show that the introduction of mise-en-scéne features in conjunction with traditional movie attributes improves both offline and online quality of recommendations.
2016
Jannach, Dietmar; Tuzhilin, Alexander; Zanker, Markus
Recommender Systems - Beyond Matrix Completion Journal Article
In: Communications of the ACM, vol. 59, no. 11, pp. 94-102, 2016, (Pre SFI).
@article{Jannach2016,
title = {Recommender Systems - Beyond Matrix Completion},
author = {Dietmar Jannach and Alexander Tuzhilin and Markus Zanker },
url = {https://www.researchgate.net/publication/309600906_Recommender_systems---_beyond_matrix_completion},
doi = {10.1145/2891406},
year = {2016},
date = {2016-10-01},
journal = {Communications of the ACM},
volume = {59},
number = {11},
pages = {94-102},
abstract = {Recommender systems have become a natural part of the user experience in today's online world. These systems are able to deliver value both for users and providers and are one prominent example where the output of academic research has a direct impact on the advancements in industry. In this article, we have briefy reviewed the history of this multidis-ciplinary field and looked at recent efforts in the research community to consider the variety of factors that may influence the long-term success of a recommender system. The list of open issues and success factors is still far from complete and new challenges arise constantly that require further research. For example, the huge amounts of user data and preference signals that become available through the Social Web and the Internet of Things not only leads to technical challenges such as scalability, but also to societal questions concerning user privacy. Based on our reflections on the developments in the field, we finally emphasize the need for a more holistic research approach that combines the insights of different disciplines. We urge that research focuses even more on practical problems that matter and are truly suited to increase the utility of recommendations from the viewpoint of the users.
},
note = {Pre SFI},
keywords = {},
pubstate = {published},
tppubtype = {article}
}
Elahi, Mehdi; Ricci, Francesco; Rubens, Neil
A survey of active learning in collaborative filtering recommender systems Journal Article
In: Computer Science Review, vol. 20, pp. 29-50, 2016, (Pre SFI).
@article{Elahi2016,
title = {A survey of active learning in collaborative filtering recommender systems},
author = {Mehdi Elahi and Francesco Ricci and Neil Rubens},
url = {https://reader.elsevier.com/reader/sd/pii/S1574013715300150?token=EA12A462FC07F42733F4F13375217A57D3FDC7F6047C133156CB1F4E4487DF24C5366547DF4530A25942F690233F2E30},
doi = {10.1016/j.cosrev.2016.05.002},
year = {2016},
date = {2016-06-02},
journal = {Computer Science Review},
volume = {20},
pages = {29-50},
abstract = {In collaborative filtering recommender systems user’s preferences are expressed as ratings for items, and each additional rating extends the knowledge of the system and affects the system’s recommendation accuracy. In general, the more ratings are elicited from the users, the more effective the recommendations are. However, the usefulness of each rating may vary significantly, i.e., different ratings may bring a different amount and type of information about the user’s tastes. Hence, specific techniques, which are defined as “active learning strategies”, can be used to selectively choose the items to be presented to the user for rating. In fact, an active learning strategy identifies and adopts criteria for obtaining data that better reflects users’ preferences and enables to generate better recommendations.
So far, a variety of active learning strategies have been proposed in the literature. In this article, we survey recent strategies by grouping them with respect to two distinct dimensions: personalization, i.e., whether the system selected items are different for different users or not, and, hybridization, i.e., whether active learning is guided by a single criterion (heuristic) or by multiple criteria. In addition, we present a comprehensive overview of the evaluation methods and metrics that have been employed by the research community in order to test active learning strategies for collaborative filtering. Finally, we compare the surveyed strategies and provide guidelines for their usage in recommender systems.},
note = {Pre SFI},
keywords = {},
pubstate = {published},
tppubtype = {article}
}
So far, a variety of active learning strategies have been proposed in the literature. In this article, we survey recent strategies by grouping them with respect to two distinct dimensions: personalization, i.e., whether the system selected items are different for different users or not, and, hybridization, i.e., whether active learning is guided by a single criterion (heuristic) or by multiple criteria. In addition, we present a comprehensive overview of the evaluation methods and metrics that have been employed by the research community in order to test active learning strategies for collaborative filtering. Finally, we compare the surveyed strategies and provide guidelines for their usage in recommender systems.
Tobias, Ignacio Fernandez; Braunhofer, Matthias; Elahi, Mehdi; Ricci, Francesco; Cantador, Ivan
Alleviating the new user problem in collaborative filtering by exploiting personality information Journal Article
In: User Modeling and User-Adapted Interaction, vol. 26, no. 2-3, pp. 221-255, 2016, (Pre SFI).
@article{Tobias2016,
title = {Alleviating the new user problem in collaborative filtering by exploiting personality information},
author = {Ignacio Fernandez Tobias and Matthias Braunhofer and Mehdi Elahi and Francesco Ricci and Ivan Cantador},
url = {https://www.researchgate.net/publication/285574429_Alleviating_the_New_User_Problem_in_Collaborative_Filtering_by_Exploiting_Personality_Information},
doi = {10.1007/s11257-016-9172-z},
year = {2016},
date = {2016-06-01},
journal = {User Modeling and User-Adapted Interaction},
volume = {26},
number = {2-3},
pages = {221-255},
abstract = {The new user problem in recommender systems is still challenging, and there is not yet a unique solution that can be applied in any domain or situation. In this paper we analyze viable solutions to the new user problem in collaborative filtering (CF) that are based on the exploitation of user personality information: (a) personality-based CF, which directly improves the recommendation prediction model by incorporating user personality information, (b) personality-based active learning, which utilizes personality information for identifying additional useful preference data in the target recommendation domain to be elicited from the user, and (c) personality-based cross-domain recommendation, which exploits personality information to better use user preference data from auxiliary domains which can be used to compensate the lack of user preference data in the target domain. We benchmark the effectiveness of these methods on large datasets that span several domains, namely movies, music and books. Our results show that personality-aware methods achieve performance improvements that range from 6 to 94 % for users completely new to the system, while increasing the novelty of the recommended items by 3–40 % with respect to the non-personalized popularity baseline. We also discuss the limitations of our approach and the situations in which the proposed methods can be better applied, hence providing guidelines for researchers and practitioners in the field.},
note = {Pre SFI},
keywords = {},
pubstate = {published},
tppubtype = {article}
}
2015
Rubens, Neil; Elahi, Mehdi; Sugiyama, Masashi; Kaplan, Dain
Active learning in recommender systems Book Chapter
In: Ricci, Francesco; Rokach, Lior; Shapira, Bracha (Ed.): pp. 809-846, Springer, 2015, ISBN: 978-1-4899-7637-6, (Pre SFI).
@inbook{Rubens2015,
title = {Active learning in recommender systems},
author = {Neil Rubens and Mehdi Elahi and Masashi Sugiyama and Dain Kaplan},
editor = {Francesco Ricci and Lior Rokach and Bracha Shapira},
url = {https://link.springer.com/chapter/10.1007/978-1-4899-7637-6_24},
doi = {10.1007/978-1-4899-7637-6_24},
isbn = {978-1-4899-7637-6},
year = {2015},
date = {2015-01-01},
pages = {809-846},
publisher = {Springer},
abstract = {In Recommender Systems (RS), a user’s preferences are expressed in terms of rated items, where incorporating each rating may improve the RS’s predictive accuracy. In addition to a user rating items at-will (a passive process), RSs may also actively elicit the user to rate items, a process known as Active Learning (AL). However, the number of interactions between the RS and the user is still limited. One aim of AL is therefore the selection of items whose ratings are likely to provide the most information about the user’s preferences. In this chapter, we provide an overview of AL within RSs, discuss general objectives and considerations, and then summarize a variety of methods commonly employed. AL methods are categorized based on our interpretation of their primary motivation/goal, and then sub-classified into two commonly classified types, instance-based and model-based, for easier comprehension. We conclude the chapter by outlining ways in which AL methods could be evaluated, and provide a brief summary of methods performance.},
note = {Pre SFI},
keywords = {},
pubstate = {published},
tppubtype = {inbook}
}
0000
Abdollahpouri, Himan; Elahi, Mehdi; Mansoury, Masoud; Sahebi, Shaghayegh; Nazari, Zahra; Chaney, Allison; Loni, Babak
MORS 2021: 1st Workshop on Multi-Objective Recommender Systems. Proceedings
0000.
@proceedings{Abdollahpouri2021,
title = {MORS 2021: 1st Workshop on Multi-Objective Recommender Systems.},
author = {Himan Abdollahpouri and Mehdi Elahi and Masoud Mansoury and Shaghayegh Sahebi and Zahra Nazari and Allison Chaney and Babak Loni},
journal = {In Fifteenth ACM Conference on Recommender Systems.},
pages = {787-788},
keywords = {},
pubstate = {published},
tppubtype = {proceedings}
}
/ Publications
2024
Rosnes, Daniel; Starke, Alain; Trattner, Christoph
ACM UMAP '24, 2024.
@conference{umap2024Daniel,
title = {Shaping the Future of Content-based News Recommenders: Insights from Evaluating Feature-Specific Similarity Metrics},
author = {Daniel Rosnes and Alain Starke and Christoph Trattner },
url = {https://mediafutures.no/umap2024/},
year = {2024},
date = {2024-07-01},
booktitle = {ACM UMAP '24},
abstract = {In news media, recommender system technology faces several domain-specific challenges. The continuous stream of new content and users deems content-based recommendation strategies, based on similar-item retrieval, to remain popular. However, a persistent challenge is to select relevant features and corresponding similarity functions, and whether this depends on the specific context. We evaluated feature-specific similarity metrics using human similarity judgments across national and local news domains. We performed an online experiment ($N = 141$) where we asked participants to judge the similarity between pairs of randomly sampled news articles. We had three contributions: (1) comparing novel metrics based on large language models to ones traditionally used in news recommendations, (2) exploring differences in similarity judgments across national and local news domains, and (3) examining which content-based strategies were perceived as appropriate in the news domain. Our results showed that one of the novel large language model based metrics (SBERT) was highly correlated with human judgments, while there were only small, most non-significant differences across national and local news domains. Finally, we found that while it may be possible to automatically recommend similar news using feature-specific metrics, their representativeness and appropriateness varied. We explain how our findings can guide the design of future content-based and hybrid recommender strategies in the news domain.},
keywords = {},
pubstate = {published},
tppubtype = {conference}
}
Jeng, Jia Hua; Kasangu, Gloria Anne Babile; Starke, Alain D.; Trattner, Christoph
Emotional Reframing of Economic News using a Large Language Model Conference
ACM UMAP 2024, 2024.
@conference{emorefram24,
title = {Emotional Reframing of Economic News using a Large Language Model},
author = {Jia Hua Jeng and Gloria Anne Babile Kasangu and Alain D. Starke and Christoph Trattner},
url = {https://mediafutures.no/umap2024___jeng_alain_gloria_christoph__workshop_-3/},
year = {2024},
date = {2024-07-01},
urldate = {2024-07-01},
booktitle = {ACM UMAP 2024},
abstract = {News media framing can shape public perception and potentially polarize views. Emotional language can exacerbate these framing effects, as a user’s emotional state can be an important contextual factor to use in news recommendation. Our research explores the relation between emotional framing techniques and the emotional states of readers, as well as readers’ perceived trust in specific news articles. Users (N = 200) had to read three economic news articles from the Washington Post. We used ChatGPT-4 to reframe news articles with specific emotional languages (Anger, Fear, Hope), compared to a neutral baseline reframed by a human journalist. Our results revealed that negative framing (Anger, Fear) elicited stronger negative emotional states among users than the neutral baseline, while Hope led to little changes overall. In contrast, perceived trust levels varied little across the different conditions. We discuss the implications of our findings and how emotional framing could affect societal polarization issues},
keywords = {},
pubstate = {published},
tppubtype = {conference}
}
Starke, Alain D.; Bremnes, Anders Sandvik; Knudsen, Erik; Trilling, Damian; Trattner, Christoph
ACM UMAP 2024, 2024.
@conference{percepvsreal24,
title = {Perception versus Reality: Evaluating User Awareness of Political Selective Exposure in News Recommender Systems},
author = {Alain D. Starke and Anders Sandvik Bremnes and Erik Knudsen and Damian Trilling and Christoph Trattner},
url = {https://mediafutures.no/umap2024___erik_alain_damian_anders_christoph/},
year = {2024},
date = {2024-07-01},
urldate = {2024-07-01},
booktitle = {ACM UMAP 2024},
abstract = {News Recommender Systems (NRSs) have become increasingly pivotal in shaping the news landscape, particularly in how news is disseminated. This has also led to concerns about information diversity, especially regarding selective exposure in the realm of political news. Users may not recognize that news content presented to them is subject to selective exposure, through users that incorporate political beliefs. Within the U.S. two-party system, our research explores the interactions between NRSs and users’ ability to discern news articles that align with their political biases. We performed an online experiment (N = 160) to address the issue of user awareness and self-recognition of selective exposure within NRSs. Users were asked to select any number of news articles that matched their political orientation (i.e., Democrat or Republican) from a list of 50 news articles (5 Democrat, 5 Republican, 40 filler articles), which were either ranked saliently towards their political orientation or randomly. Contrary to expectations, our findings reveal no significant difference in article selection between participants exposed to a baseline random order and those who where presented with the more salient and easy to select version. We did observe that Republicans performed worse than Democrats in identifying aligning articles, based on precision and recall metrics.},
keywords = {},
pubstate = {published},
tppubtype = {conference}
}
Mahmood, Bilal; Elahi, Mehdi; Touileb, Samia; Steskal, Lubos; Trattner, Christoph
Incorporating Editorial Feedback in the Evaluation of News Recommender Systems Conference
ACM UMAP 2024, 2024.
@conference{incoed24,
title = {Incorporating Editorial Feedback in the Evaluation of News Recommender Systems},
author = {Bilal Mahmood and Mehdi Elahi and Samia Touileb and Lubos Steskal and Christoph Trattner},
url = {https://mediafutures.no/lbr_umap_editorial_component_in_nrs/},
year = {2024},
date = {2024-07-01},
urldate = {2024-07-01},
booktitle = {ACM UMAP 2024},
abstract = {Research in the recommender systems field typically applies a rather traditional evaluation methodology when assessing the quality of recommendations. This methodology heavily relies on incorporating different forms of user feedback (e.g., clicks) representing the specific needs and interests of the users. While this methodology may offer various benefits, it may fail to comprehensively project the complexities of certain application domains, such as the news domain. This domain is distinct from other domains primarily due to the strong influence of editorial control in the news delivery process. Incorporation of this role can profoundly impact how the relevance of news articles is measured when recommended to the users. Despite its critical importance, there appears to be a research gap in investigating the dynamics between the roles of editorial control and personalization in the community of recommender systems. In this paper, we address this gap by conducting experiments where the relevance of recommendations is assessed from an editorial perspective. We received a real-world dataset from TV 2, one of the largest editor-managed commercial media houses in Norway, which includes editors’ feedback on how news articles are being related. In our experiment, we considered a scenario where algorithm-generated recommendations, using the K-Nearest Neighbor (KNN) model, employing various text embedding models to encode different sections of the news articles (e.g., title, lead title, body text, and full text), are compared against the editorial feedback. The results are promising, demonstrating the effectiveness of the recommendation in fulfilling the editorial prospects.},
keywords = {},
pubstate = {published},
tppubtype = {conference}
}
Klimashevskaia, Anastasiia; Jannach, Dietmar; Elahi, Mehdi; Trattner, Christoph
A Survey on Popularity Bias in Recommender Systems Journal Article Forthcoming
In: User Modeling and User-Adapted Interaction (UMUAI), Forthcoming.
@article{biasanas24,
title = {A Survey on Popularity Bias in Recommender Systems},
author = {Anastasiia Klimashevskaia and Dietmar Jannach and Mehdi Elahi and Christoph Trattner},
url = {https://mediafutures.no/popularitybias_literature_review-5/},
year = {2024},
date = {2024-06-13},
journal = {User Modeling and User-Adapted Interaction (UMUAI)},
abstract = {Recommender systems help people find relevant content in a personalized way. One main promise of such systems is that they are able to increase the visibility of items in the long tail, i.e., the lesser-
known items in a catalogue. Existing research, however, suggests that in many situations today’s recommendation algorithms instead exhibit a popularity bias, meaning that they often focus on rather popular items in their recommendations. Such a bias may not only lead to the limited value of the recommendations for consumers and providers in the short run, but it may also cause undesired reinforcement effects over time. In this paper, we discuss the potential reasons for popularity bias and review existing approaches to detect, quantify and mitigate popularity bias in
recommender systems. Our survey, therefore, includes both an overview
of the computational metrics used in the literature as well as a review of the main technical approaches to reduce the bias. Furthermore, we critically discuss today’s literature, where we observe that the research is almost entirely based on computational experiments and on certain
assumptions regarding the practical effects of including long-tail items in the recommendations.},
keywords = {},
pubstate = {forthcoming},
tppubtype = {article}
}
known items in a catalogue. Existing research, however, suggests that in many situations today’s recommendation algorithms instead exhibit a popularity bias, meaning that they often focus on rather popular items in their recommendations. Such a bias may not only lead to the limited value of the recommendations for consumers and providers in the short run, but it may also cause undesired reinforcement effects over time. In this paper, we discuss the potential reasons for popularity bias and review existing approaches to detect, quantify and mitigate popularity bias in
recommender systems. Our survey, therefore, includes both an overview
of the computational metrics used in the literature as well as a review of the main technical approaches to reduce the bias. Furthermore, we critically discuss today’s literature, where we observe that the research is almost entirely based on computational experiments and on certain
assumptions regarding the practical effects of including long-tail items in the recommendations.
Starke, Alain D.; Willemsen, Martijn C.
In: pp. 221–259, A Human-Centered Perspective of Intelligent Personalized Environments and Systems, 2024.
@inbook{Alain_pysch24,
title = {Psychologically Informed Design of Energy Recommender Systems: Are Nudges Still Effective in Tailored Choice Environments?},
author = {Alain D. Starke and Martijn C. Willemsen},
url = {https://mediafutures.no/starke2024-book-chapter-psych-informed-energy-recsys-4/},
year = {2024},
date = {2024-05-01},
urldate = {2024-05-01},
pages = {221–259},
edition = {A Human-Centered Perspective of Intelligent Personalized Environments and Systems},
series = {Human–Computer Interaction Series},
keywords = {},
pubstate = {published},
tppubtype = {inbook}
}
Pera, Maria Soledad; Cena, Federica; Landoni, Monica; Musto, Cataldo; Starke, Alain D.
Human Factors in User Modeling for Intelligent Systems Book Chapter
In: pp. 3–42, A Human-Centered Perspective of Intelligent Personalized Environments and Systems, 2024.
@inbook{Alain_humanF24,
title = {Human Factors in User Modeling for Intelligent Systems},
author = {Maria Soledad Pera and Federica Cena and Monica Landoni and Cataldo Musto and Alain D. Starke},
url = {https://mediafutures.no/pera2024-book-chapter-holistic-user-modeling/},
year = {2024},
date = {2024-05-01},
urldate = {2024-05-01},
pages = {3–42},
edition = {A Human-Centered Perspective of Intelligent Personalized Environments and Systems},
series = {Human–Computer Interaction Series.},
abstract = {In the current digital landscape, humans take center stage. This has caused a paradigm shift in the realm of intelligent technologies, prompting researchers and (industry) practitioners to reflect on the challenges and complexities involved in understanding the (potential) users of the technologies they develop. In this chapter, we provide an overview of human factors in user modeling, a core component of human-centered intelligent solutions. We discuss critical concepts, dimensions, and theories that inform the design of user models that are more attuned to human characteristics. Additionally, we emphasize the need for a comprehensive user model that simultaneously considers multiple factors to represent the intricacies of individuals’ interests and behaviors. Such a holistic model can, in turn, shape the design of intelligent solutions that are better able to adapt and cater to a wide range of user groups.},
keywords = {},
pubstate = {published},
tppubtype = {inbook}
}
2023
Knudsen, Erik; Starke, Alain D.; Trattner, Christoph
Association for Computing Machinery (ACM) RecSys ’23, 2023.
@conference{inra2023-1,
title = {Topical Preference Trumps Other Features in News Recommendation: A Conjoint Analysis on a Representative Sample from Norway},
author = {Erik Knudsen and Alain D. Starke and Christoph Trattner },
url = {https://mediafutures.no/inra2023-1/},
year = {2023},
date = {2023-09-18},
urldate = {2023-09-18},
booktitle = {Association for Computing Machinery (ACM) RecSys ’23},
keywords = {},
pubstate = {published},
tppubtype = {conference}
}
Seddik, Khadiga; Knudsen, Erik; Trilling, Damian; Trattner, Christoph
Understanding How News Recommender Systems Influence Selective Exposure Conference
Association for Computing Machinery (ACM) RecSys ’23, 2023.
@conference{behavrec2023,
title = {Understanding How News Recommender Systems Influence Selective Exposure},
author = {Khadiga Seddik and Erik Knudsen and Damian Trilling and Christoph Trattner },
url = {https://mediafutures.no/behavrec2023/},
year = {2023},
date = {2023-09-18},
urldate = {2023-09-18},
booktitle = {Association for Computing Machinery (ACM) RecSys ’23},
keywords = {},
pubstate = {published},
tppubtype = {conference}
}
Klimashevskaia, Anastasiia; Elahi, Mehdi; Jannach, Dietmar; Skjærven, Lars; Tessem, Astrid; Trattner, Christoph
Evaluating The Effects of Calibrated Popularity Bias Mitigation: A Field Study Conference
Association for Computing Machinery (ACM) RecSys ’23, 2023.
@conference{RecSys_2023_LBR,
title = {Evaluating The Effects of Calibrated Popularity Bias Mitigation: A Field Study},
author = {Anastasiia Klimashevskaia and Mehdi Elahi and Dietmar Jannach and Lars Skjærven and Astrid Tessem and Christoph Trattner},
url = {https://mediafutures.no/recsys_2023_lbr/},
year = {2023},
date = {2023-09-18},
booktitle = {Association for Computing Machinery (ACM) RecSys ’23},
keywords = {},
pubstate = {published},
tppubtype = {conference}
}
Majjodi, Ayoub El; Starke, Alain D.; Trattner, Christoph
Association for Computing Machinery (ACM) RecSys ’23, 2023.
@conference{inra2023,
title = {The Interplay between Food Knowledge, Nudges, and Preference Elicitation Methods Determines the Evaluation of a Recipe Recommender System},
author = {Ayoub El Majjodi and Alain D. Starke and Christoph Trattner },
url = {https://mediafutures.no/intrs2023-2/},
year = {2023},
date = {2023-09-18},
urldate = {2023-09-18},
booktitle = {Association for Computing Machinery (ACM) RecSys ’23},
keywords = {},
pubstate = {published},
tppubtype = {conference}
}
Starke, Alain; Emami, Kimia; Makarová, Andrea; Ferwerda, Bruce
Using Visual and Linguistic Framing to Support Sustainable Decisions in an Online Store Conference
Association for Computing Machinery (ACM) RecSys ’23,, 2023.
@conference{intrs23_session3,
title = {Using Visual and Linguistic Framing to Support Sustainable Decisions in an Online Store},
author = {Alain Starke and Kimia Emami and Andrea Makarová and Bruce Ferwerda },
url = {https://mediafutures.no/intrs23_session3_paper_2/},
year = {2023},
date = {2023-09-18},
urldate = {2023-09-18},
booktitle = {Association for Computing Machinery (ACM) RecSys ’23,},
keywords = {},
pubstate = {published},
tppubtype = {conference}
}
Jeng, Jia Hua; Starke, Alain D.; Trattner, Christoph
Towards Attitudinal Change in News Recommender Systems: A Pilot Study on Climate Change Workshop
2023.
@workshop{Jeng2023,
title = {Towards Attitudinal Change in News Recommender Systems: A Pilot Study on Climate Change},
author = {Jia Hua Jeng and Alain D. Starke and Christoph Trattner},
url = {https://mediafutures.no/jeng2023-towards-attitudinal-change-in-news2908-2/},
year = {2023},
date = {2023-04-18},
urldate = {2023-04-18},
abstract = {Personalized recommender systems facilitate decision-making in various domains by presenting content closely aligned with users’ preferences.
However, personalization can lead to unintended consequences. In news, selective information exposure and consumption might amplify
polarization, as users are empowered to seek out information that is in line with their own attitudes and viewpoints. However, personalization in
terms of algorithmic content and persuasive technology could also help to narrow the gap between polarized user attitudes and news consumption
patterns. This paper presents a pilot study on climate change news. We examined the relation between users’ level of environmental concern, their preferences
for news articles, and news article content. We aimed to capture a news article’s viewpoint through sentiment analysis. Users (N = 180)
were asked to read and evaluate 10 news articles from the Washington Post. We found a positive correlation between users’ level of environmental
concern and whether they liked the article. In contrast, no significant correlation was found between sentiment and environmental concern.
We argue why a different type of news article analysis than sentiment is needed. Finally, we present our research agenda on how persuasive technology
might help to support more exploration of news article viewpoints in the future.},
keywords = {},
pubstate = {published},
tppubtype = {workshop}
}
However, personalization can lead to unintended consequences. In news, selective information exposure and consumption might amplify
polarization, as users are empowered to seek out information that is in line with their own attitudes and viewpoints. However, personalization in
terms of algorithmic content and persuasive technology could also help to narrow the gap between polarized user attitudes and news consumption
patterns. This paper presents a pilot study on climate change news. We examined the relation between users’ level of environmental concern, their preferences
for news articles, and news article content. We aimed to capture a news article’s viewpoint through sentiment analysis. Users (N = 180)
were asked to read and evaluate 10 news articles from the Washington Post. We found a positive correlation between users’ level of environmental
concern and whether they liked the article. In contrast, no significant correlation was found between sentiment and environmental concern.
We argue why a different type of news article analysis than sentiment is needed. Finally, we present our research agenda on how persuasive technology
might help to support more exploration of news article viewpoints in the future.
2022
Majjodi, Ayoub El; Starke, Alain D.; Trattner, Christoph
Nudging Towards Health? Examining the Merits of Nutrition Labels and Personalization in a Recipe Recommender System, 2022.
@conference{Majjodi2022,
title = {Nudging Towards Health? Examining the Merits of Nutrition Labels and Personalization in a Recipe Recommender System},
author = { Ayoub El Majjodi and Alain D. Starke and Christoph Trattner
},
url = {https://dl.acm.org/doi/10.1145/3503252.3531312?fbclid=IwAR0eb6MPuISpVs9Vfkd-ww_KN7EjbMbiGdDQnPxjayogfKbHFgkSgeLdaxs},
year = {2022},
date = {2022-07-03},
urldate = {2022-07-03},
booktitle = {Nudging Towards Health? Examining the Merits of Nutrition Labels and Personalization in a Recipe Recommender System},
abstract = {Food recommender systems show personalized recipes to users based on content liked previously. Despite their potential, often recommended (popular) recipes in previous studies have turned out to be unhealthy, negatively contributing to prevalent obesity problems worldwide. Changing how foods are presented through digital nudges might help, but these are usually examined in non-personalized contexts, such as a brick-and-mortar supermarket. This study seeks to support healthy food choices in a personalized interface by adding front-of-package nutrition labels to recipes in a food recommender system. After performing an offline evaluation, we conducted an online study (N = 600) with six different recommender interfaces, based on a 2 (non-personalized vs. personalized recipe advice) x 3 (No Label, Multiple Traffic Light, Nutri-Score) between-subjects design. We found that recipe choices made in the non-personalized scenario were healthier, while the use of nutrition labels (our digital nudge) reduced choice difficulty when the content was personalized.},
keywords = {},
pubstate = {published},
tppubtype = {conference}
}
Elahi, Mehdi; Starke, Alain D.; Ioini, Nabil El; Lambrix, Anna Alexander; Trattner, Christoph
Developing and Evaluating a University Recommender System Journal Article
In: Frontiers in Artificial Intelligence , 2022.
@article{Elahi2022,
title = {Developing and Evaluating a University Recommender System},
author = {Mehdi Elahi and Alain D. Starke and Nabil El Ioini and Anna Alexander Lambrix and Christoph Trattner},
url = {https://www.frontiersin.org/articles/10.3389/frai.2021.796268/full},
doi = {https://doi.org/10.3389/frai.2021.796268},
year = {2022},
date = {2022-02-02},
journal = {Frontiers in Artificial Intelligence },
abstract = {A challenge for many young adults is to find the right institution to follow higher education. Global university rankings are a commonly used, but inefficient tool, for they do not consider a person's preferences and needs. For example, some persons pursue prestige in their higher education, while others prefer proximity. This paper develops and evaluates a university recommender system, eliciting user preferences as ratings to build predictive models and to generate personalized university ranking lists. In Study 1, we performed offline evaluation on a rating dataset to determine which recommender approaches had the highest predictive value. In Study 2, we selected three algorithms to produce different university recommendation lists in our online tool, asking our users to compare and evaluate them in terms of different metrics (Accuracy, Diversity, Perceived Personalization, Satisfaction, and Novelty). We show that a SVD algorithm scores high on accuracy and perceived personalization, while a KNN algorithm scores better on novelty. We also report findings on preferred university features.},
keywords = {},
pubstate = {published},
tppubtype = {article}
}
2021
Starke, Alain D.; Willemsen, Martijn C.; Trattner, Christoph
Nudging Healthy Choices in Food Search Through Visual Attractiveness Journal Article
In: no. April 2021, pp. 1-18, 2021.
@article{Starke2021,
title = {Nudging Healthy Choices in Food Search Through Visual Attractiveness},
author = {Alain D. Starke and Martijn C. Willemsen and Christoph Trattner},
url = {https://www.frontiersin.org/articles/10.3389/frai.2021.621743/full},
doi = {10.3389/frai.2021.621743},
year = {2021},
date = {2021-04-22},
number = {April 2021},
pages = {1-18},
abstract = {Recipe websites are becoming increasingly popular to support people in their home cooking. However, most of these websites prioritize popular recipes, which tend to be unhealthy. Drawing upon research on visual biases and nudges, this paper investigates whether healthy food choices can be supported in food search by depicting attractive images alongside recipes, as well as by re-ranking search results on health. After modelling the visual attractiveness of recipe images, we asked 239 users to search for specific online recipes and to select those they liked the most. Our analyses revealed that users tended to choose a healthier recipe if a visually attractive image was depicted alongside it, as well as if it was listed at the top of a list of search results. Even though less popular recipes were promoted this way, it did not come at the cost of a user’s level of satisfaction},
keywords = {},
pubstate = {published},
tppubtype = {article}
}
2020
Endriss, Ulle; de Haan, Ronald; Lang, Jerôme; Slavkovik, Marija
The complexity landscape of outcome determination in judgment aggregation Journal Article
In: Journal of Artificial Intelligence Research, vol. 69, pp. 687–731, 2020, (Pre SFI).
@article{Endriss2020,
title = {The complexity landscape of outcome determination in judgment aggregation},
author = {Ulle Endriss and Ronald de Haan and Jerôme Lang and Marija Slavkovik },
url = {https://www.jair.org/index.php/jair/article/view/11970/26619},
doi = {10.1613/jair.1.11970},
year = {2020},
date = {2020-11-04},
journal = {Journal of Artificial Intelligence Research},
volume = {69},
pages = {687–731},
abstract = {We provide a comprehensive analysis of the computational complexity of the outcome determinationproblem for the most important aggregation rules proposed in the literature on logic-based judgmentaggregation. Judgment aggregation is a powerful and flexible framework for studying problems ofcollective decision making that has attracted interest in a range of disciplines, including Legal Theory,Philosophy, Economics, Political Science, and Artificial Intelligence. The problem of computing theoutcome for a given list of individual judgments to be aggregated into a single collective judgment isthe most fundamental algorithmic challenge arising in this context. Our analysis applies to severaldifferent variants of the basic framework of judgment aggregation that have been discussed in theliterature, as well as to a new framework that encompasses all existing such frameworks in terms ofexpressive power and representational succinctness.},
note = {Pre SFI},
keywords = {},
pubstate = {published},
tppubtype = {article}
}
Hazrati, Naieme; Elahi, Mehdi
Addressing the New Item problem in video recommender systems by incorporation of visual features with restricted Boltzmann machines. Journal Article
In: Expert Systems, vol. e12645, pp. 1-20, 2020, (Pre SFI).
@article{Hazrati2020,
title = { Addressing the New Item problem in video recommender systems by incorporation of visual features with restricted Boltzmann machines.},
author = {Naieme Hazrati and Mehdi Elahi},
url = {https://onlinelibrary.wiley.com/doi/epdf/10.1111/exsy.12645},
doi = {https://doi.org/10.1111/exsy.12645},
year = {2020},
date = {2020-10-19},
journal = {Expert Systems},
volume = {e12645},
pages = {1-20},
abstract = {Over the past years, the research of video recommender systems (RSs) has been mainly focussed on the development of novel algorithms. Although beneficial, still any algorithm may fail to recommend video items that the system has no form of data associated to them (New Item Cold Start). This problem occurs when a new item is added to the catalogue of the system and no data are available for that item. In content‐based RSs, the video items are typically represented by semantic attributes, when generating recommendations. These attributes require a group of experts or users for annotation, and still, the generated recommendations might not capture a complete picture of the users' preferences, for example, the visual tastes of users on video style. This article addresses this problem by proposing recommendation based on novel visual features that do not require human annotation and can represent visual aspects of video items. We have designed a novel evaluation methodology considering three realistic scenarios, that is, (a) extreme cold start, (b) moderate cold start and (c) warm‐start scenario. We have conducted a set of comprehensive experiments, and our results have shown the superior performance of recommendations based on visual features, in all of the evaluation scenarios.},
note = {Pre SFI},
keywords = {},
pubstate = {published},
tppubtype = {article}
}
Balog, Krisztian; Radlinski, Filip
Measuring Recommendation Explanation Quality: The Conflicting Goals of Explanations Conference
Proceedings of the 43rd International ACM SIGIR Conference on Research and Development in Information Retrieval (SIGIR '20), New York, 2020, (Pre SFI).
@conference{Balog2020,
title = {Measuring Recommendation Explanation Quality: The Conflicting Goals of Explanations},
author = {Krisztian Balog and Filip Radlinski},
url = {https://dl.acm.org/doi/pdf/10.1145/3397271.3401032},
doi = {https://doi.org/10.1145/3397271.3401032},
year = {2020},
date = {2020-07-01},
booktitle = {Proceedings of the 43rd International ACM SIGIR Conference on Research and Development in Information Retrieval (SIGIR '20)},
pages = {329–338},
address = {New York},
abstract = {Explanations have a large effect on how people respond to recommendations. However, there are many possible intentions a system may have in generating explanations for a given recommendation -from increasing transparency, to enabling a faster decision, to persuading the recipient. As a good explanation for one goal may not be good for others, we address the questions of (1) how to robustly measure if an explanation meets a given goal and (2) how the different goals interact with each other. Specifically, this paper presents a first proposal of how to measure the quality of explanations along seven common goal dimensions catalogued in the literature. We find that the seven goals are not independent, but rather exhibit strong structure. Proposing two novel explanation evaluation designs, we identify challenges in evaluation, and provide more efficient measurement approaches of explanation quality.},
note = {Pre SFI},
keywords = {},
pubstate = {published},
tppubtype = {conference}
}
Soe, Than Htut; Nordberg, Oda Elise; Guribye, Frode; Slavkovik, Marija
Circumvention by design - dark patterns in cookie consents for online news outlets Conference
Proceedings of the 11th Nordic Conference on Human-Computer Interaction, 2020, (Pre SFI).
@conference{Soe2020,
title = {Circumvention by design - dark patterns in cookie consents for online news outlets},
author = {Than Htut Soe and Oda Elise Nordberg and Frode Guribye and Marija Slavkovik},
url = {https://dl.acm.org/doi/pdf/10.1145/3419249.3420132},
doi = {10.1145/3419249.3420132},
year = {2020},
date = {2020-06-24},
booktitle = {Proceedings of the 11th Nordic Conference on Human-Computer Interaction},
abstract = {To ensure that users of online services understand what data are collected and how they are used in algorithmic decision-making, the European Union's General Data Protection Regulation (GDPR) specifies informed consent as a minimal requirement. For online news outlets consent is commonly elicited through interface design elements in the form of a pop-up. We have manually analyzed 300 data collection consent notices from news outlets that are built to ensure compliance with GDPR. The analysis uncovered a variety of strategies or dark patterns that circumvent the intent of GDPR by design. We further study the presence and variety of these dark patterns in these "cookie consents" and use our observations to specify the concept of dark pattern in the context of consent elicitation.},
note = {Pre SFI},
keywords = {},
pubstate = {published},
tppubtype = {conference}
}
Nunes, Ingrid; Jannach, Dietmar
A Systematic Review and Taxonomy of Explanations in Decision Support and Recommender Systems Journal Article
In: User-Modeling and User-Adapted Interaction, vol. 27, no. 3-5, pp. 393-444, 2020, (Pre SFI).
@article{Nunes2020,
title = {A Systematic Review and Taxonomy of Explanations in Decision Support and Recommender Systems},
author = {Ingrid Nunes and Dietmar Jannach},
url = {https://arxiv.org/pdf/2006.08672.pdf},
doi = {10.1007/s11257-017-9195-0},
year = {2020},
date = {2020-06-15},
journal = {User-Modeling and User-Adapted Interaction},
volume = {27},
number = {3-5},
pages = {393-444},
abstract = {With the recent advances in the field of artificial intelligence, an increasing number of decision-making tasks are delegated to software systems. A key requirement for the success and adoption of such systems is that users must trust system choices or even fully automated decisions. To achieve this, explanation facilities have been widely investigated as a means of establishing trust in these systems since the early years of expert systems. With today's increasingly sophisticated machine learning algorithms, new challenges in the context of explanations, accountability, and trust towards such systems constantly arise. In this work, we systematically review the literature on explanations in advice-giving systems. This is a family of systems that includes recommender systems, which is one of the most successful classes of advice-giving software in practice. We investigate the purposes of explanations as well as how they are generated, presented to users, and evaluated. As a result, we derive a novel comprehensive taxonomy of aspects to be considered when designing explanation facilities for current and future decision support systems. The taxonomy includes a variety of different facets, such as explanation objective, responsiveness, content and presentation. Moreover, we identified several challenges that remain unaddressed so far, for example related to fine-grained issues associated with the presentation of explanations and how explanation facilities are evaluated.},
note = {Pre SFI},
keywords = {},
pubstate = {published},
tppubtype = {article}
}
Sjøvaag, Helle; Owren, Thomas; Borgen, Turid
Strategic and Organisational fit in Corporate News Markets: A Principal-agent Approach to Studying Newspaper Mergers Journal Article
In: Journalism Practice, pp. 1-18, 2020, (Pre SFI).
@article{Sjøvaag2020,
title = {Strategic and Organisational fit in Corporate News Markets: A Principal-agent Approach to Studying Newspaper Mergers},
author = {Helle Sjøvaag and Thomas Owren and Turid Borgen},
url = {https://www.tandfonline.com/doi/epub/10.1080/17512786.2020.1772097?needAccess=true},
doi = {https://doi.org/10.1080/17512786.2020.1772097},
year = {2020},
date = {2020-05-20},
journal = {Journalism Practice},
pages = {1-18},
abstract = {This article analyses strategic and organisational fit in corporate newspaper mergers in the context of the digitalisation of local newspaper markets. Using the 2019 acquisition of Nordsjø Media by Amedia in Norway as case, we analyse how eight editors-in-chief perceive the process of incorporating small, low-frequency, print-oriented monopolistic newspapers into one of Scandinavia’s largest newspaper chains. The semi-structured interviews were analysed in light of perceived strategic and organisational fit in a principal-agent theoretical framework, the aim of which is to shed light on corporate ownership effects in consolidated newspaper markets. The analysis reveals the precarity of independent ownership in digitising news markets, to which corporatisation emerges as a necessary and welcomed solution. We find the strategic fit as perceived by editors to be tied to technological resources and scale economics, while organisational fit is hampered by the speed and pressure of corporatisation processes. While these results largely support findings from previous acquisition studies in the news industries, the contribution of this analysis lies primarily with the necessity of scale required by the technological transformation that forces independent newspapers to submit to larger chain operations and how it influences considerations of fit in disruptive digital news markets.},
note = {Pre SFI},
keywords = {},
pubstate = {published},
tppubtype = {article}
}
Zhang, Shuo; Balog, Krisztian
Web Table Extraction, Retrieval, and Augmentation: A Survey Journal Article
In: ACM Transactions on Intelligent Systems and Technology (TIST), vol. 11, no. 2, pp. 1-35, 2020, (Pre SFI).
@article{Zhang2020,
title = {Web Table Extraction, Retrieval, and Augmentation: A Survey},
author = {Shuo Zhang and Krisztian Balog},
url = {https://arxiv.org/pdf/2002.00207.pdf},
year = {2020},
date = {2020-02-01},
journal = {ACM Transactions on Intelligent Systems and Technology (TIST)},
volume = {11},
number = {2},
pages = {1-35},
abstract = {Tables are a powerful and popular tool for organizing and manipulating data. A vast number of tables can be found on the Web, which represents a valuable knowledge resource. The objective of this survey is to synthesize and present two decades of research on web tables. In particular, we organize existing literature into six main categories of information access tasks: table extraction, table interpretation, table search, question answering, knowledge base augmentation, and table augmentation. For each of these tasks, we identify and describe seminal approaches, present relevant resources, and point out interdependencies among the different tasks.},
note = {Pre SFI},
keywords = {},
pubstate = {published},
tppubtype = {article}
}
2019
Dacrema, Maurizio Ferrari; Cremonesi, Paolo; Jannach, Dietmar
Are We Really Making Much Progress? A Worrying Analysis of Recent Neural Recommendation Approaches Conference
Proceedings of the 2019 ACM Conference on Recommender Systems (RecSys 2019), Copenhagen, 2019, (Pre SFI).
@conference{Dacrema2019,
title = {Are We Really Making Much Progress? A Worrying Analysis of Recent Neural Recommendation Approaches},
author = {Maurizio Ferrari Dacrema and Paolo Cremonesi and Dietmar Jannach},
url = {https://arxiv.org/pdf/1907.06902.pdf},
year = {2019},
date = {2019-08-16},
booktitle = {Proceedings of the 2019 ACM Conference on Recommender Systems (RecSys 2019)},
address = {Copenhagen},
abstract = {Deep learning techniques have become the method of choice for
researchers working on algorithmic aspects of recommender systems. With the strongly increased interest in machine learning in
general, it has, as a result, become difficult to keep track of what
represents the state-of-the-art at the moment, e.g., for top-n recommendation tasks. At the same time, several recent publications
point out problems in today’s research practice in applied machine
learning, e.g., in terms of the reproducibility of the results or the
choice of the baselines when proposing new models.
In this work, we report the results of a systematic analysis of algorithmic proposals for top-n recommendation tasks. Specifically,
we considered 18 algorithms that were presented at top-level research conferences in the last years. Only 7 of them could be reproduced with reasonable effort. For these methods, it however
turned out that 6 of them can often be outperformed with comparably simple heuristic methods, e.g., based on nearest-neighbor or
graph-based techniques. The remaining one clearly outperformed
the baselines but did not consistently outperform a well-tuned nonneural linear ranking method. Overall, our work sheds light on a
number of potential problems in today’s machine learning scholarship and calls for improved scientific practices in this area.},
note = {Pre SFI},
keywords = {},
pubstate = {published},
tppubtype = {conference}
}
researchers working on algorithmic aspects of recommender systems. With the strongly increased interest in machine learning in
general, it has, as a result, become difficult to keep track of what
represents the state-of-the-art at the moment, e.g., for top-n recommendation tasks. At the same time, several recent publications
point out problems in today’s research practice in applied machine
learning, e.g., in terms of the reproducibility of the results or the
choice of the baselines when proposing new models.
In this work, we report the results of a systematic analysis of algorithmic proposals for top-n recommendation tasks. Specifically,
we considered 18 algorithms that were presented at top-level research conferences in the last years. Only 7 of them could be reproduced with reasonable effort. For these methods, it however
turned out that 6 of them can often be outperformed with comparably simple heuristic methods, e.g., based on nearest-neighbor or
graph-based techniques. The remaining one clearly outperformed
the baselines but did not consistently outperform a well-tuned nonneural linear ranking method. Overall, our work sheds light on a
number of potential problems in today’s machine learning scholarship and calls for improved scientific practices in this area.
de Haan, Ronald; Slavkovik, Marija
Answer set programming for judgment aggregation Conference
Proceedings of the Twenty-Eighth International Joint Conference on Artificial Intelligence, International Joint Conferences on Artificial Intelligence, 2019, (Pre SFI).
@conference{deHaan2019,
title = {Answer set programming for judgment aggregation},
author = {Ronald de Haan and Marija Slavkovik},
url = {https://www.ijcai.org/Proceedings/2019/0231.pdf},
year = {2019},
date = {2019-08-10},
booktitle = {Proceedings of the Twenty-Eighth International Joint Conference on Artificial Intelligence},
pages = {1668-1674},
publisher = {International Joint Conferences on Artificial Intelligence},
abstract = {Judgment aggregation (JA) studies how to aggregate truth valuations on logically related issues. Computing the outcome of aggregation procedures is notoriously computationally hard, which is the likely reason that no implementation of them exists as of yet. However, even hard problems sometimes need to be solved. The worst-case computational complexity of answer set programming (ASP) matches that of most problems in judgment aggregation. We take advantage of this and propose a natural and modular encoding of various judgment aggregation procedures and related problems in JA into ASP. With these encodings, we achieve two results: (1) paving the way towards constructing a wide range of new benchmark instances (from JA) for answer set solving algorithms; and (2) providing an automated tool for researchers in the area of judgment aggregation.},
note = {Pre SFI},
keywords = {},
pubstate = {published},
tppubtype = {conference}
}
Balog, Krisztian; Radlinski, Filip; Arakelyan, Shushan
Transparent, Scrutable and Explainable User Models for Personalized Recommendation Conference
Proceedings of the 42nd International ACM SIGIR Conference on Research and Development in Information Retrieval (SIGIR’19), 2019, (Pre SFI).
@conference{Balog2019,
title = {Transparent, Scrutable and Explainable User Models for Personalized Recommendation},
author = {Krisztian Balog and Filip Radlinski and Shushan Arakelyan},
url = {https://dl.acm.org/doi/pdf/10.1145/3331184.3331211},
doi = {https://doi.org/10.1145/3331184.3331211},
year = {2019},
date = {2019-07-25},
booktitle = {Proceedings of the 42nd International ACM SIGIR Conference on Research and Development in Information Retrieval (SIGIR’19)},
pages = {265–274},
abstract = {Most recommender systems base their recommendations on implicit or explicit item-level feedback provided by users. These item ratings are combined into a complex user model, which then predicts the suitability of other items. While effective, such methods have limited scrutability and transparency. For instance, if a user's interests change, then many item ratings would usually need to be modified to significantly shift the user's recommendations. Similarly, explaining how the system characterizes the user is impossible, short of presenting the entire list of known item ratings. In this paper, we present a new set-based recommendation technique that permits the user model to be explicitly presented to users in natural language, empowering users to understand recommendations made and improve the recommendations dynamically. While performing comparably to traditional collaborative filtering techniques in a standard static setting, our approach allows users to efficiently improve recommendations. Further, it makes it easier for the model to be validated and adjusted, building user trust and understanding.},
note = {Pre SFI},
keywords = {},
pubstate = {published},
tppubtype = {conference}
}
Sjøvaag, Helle
Journalism between the state and the market Book
Routledge, New York, 2019, ISBN: 9781138543348, (Pre SFI).
@book{Sjøvaag2019,
title = {Journalism between the state and the market},
author = {Helle Sjøvaag},
url = {https://www.researchgate.net/publication/332449932_Journalism_Between_the_State_and_the_Market},
isbn = {9781138543348},
year = {2019},
date = {2019-04-24},
publisher = {Routledge},
address = {New York},
abstract = {Using the Nordic media model as an empirical backdrop, Journalism Between the State and the Market defines and analyzes journalism’s fundamental problem: its shifting location between the state and the market.
This book examines how this distance is decreasing as journalism steps closer to both the market (algorithmically monetizing audiences) and the state (lobbying governments for subsidies and attacking public service broadcasting). The book analyzes journalism’s negotiated position between the market and the state in the age of disruptions, offering a theoretical foundation that seeks to account for the structural conditions of journalism in the digital age.
For scholars, graduates and students in journalism, news sociology and media and communication studies, Journalism Between the State and the Market provides a theoretical perspective that can be used as a valuable tool when studying and observing the current developments in journalism.},
note = {Pre SFI},
keywords = {},
pubstate = {published},
tppubtype = {book}
}
This book examines how this distance is decreasing as journalism steps closer to both the market (algorithmically monetizing audiences) and the state (lobbying governments for subsidies and attacking public service broadcasting). The book analyzes journalism’s negotiated position between the market and the state in the age of disruptions, offering a theoretical foundation that seeks to account for the structural conditions of journalism in the digital age.
For scholars, graduates and students in journalism, news sociology and media and communication studies, Journalism Between the State and the Market provides a theoretical perspective that can be used as a valuable tool when studying and observing the current developments in journalism.
Liao, Beishui; Slavkovik, Marija; van der Torre, Leendert
Building Jiminy Cricket: An architecture for moral agreements among stakeholders Conference
Proceedings of the 2019 AAAI/ACM Conference on AI, Ethics, and Society, 2019, (Pre SFI).
@conference{Liao2019,
title = {Building Jiminy Cricket: An architecture for moral agreements among stakeholders},
author = {Beishui Liao and Marija Slavkovik and Leendert van der Torre},
url = {https://arxiv.org/pdf/1812.04741.pdf},
year = {2019},
date = {2019-03-07},
booktitle = {Proceedings of the 2019 AAAI/ACM Conference on AI, Ethics, and Society},
pages = {147–153},
abstract = {An autonomous system is constructed by a manufacturer, operates in a society subject to norms and laws, and is interacting with end-users. We address the
challenge of how the moral values and views of all stakeholders can be integrated
and reflected in the moral behaviour of the autonomous system. We propose an artificial moral agent architecture that uses techniques from normative systems and
formal argumentation to reach moral agreements among stakeholders. We show
how our architecture can be used not only for ethical practical reasoning and collaborative decision-making, but also for the explanation of such moral behavior.},
note = {Pre SFI},
keywords = {},
pubstate = {published},
tppubtype = {conference}
}
challenge of how the moral values and views of all stakeholders can be integrated
and reflected in the moral behaviour of the autonomous system. We propose an artificial moral agent architecture that uses techniques from normative systems and
formal argumentation to reach moral agreements among stakeholders. We show
how our architecture can be used not only for ethical practical reasoning and collaborative decision-making, but also for the explanation of such moral behavior.
2018
Sjøvaag, Helle; Pedersen, Truls André; Owren, Thomas
Is public service broadcasting a threat to commercial media? Journal Article
In: Media, Culture & Society, vol. 46, no. 1, pp. 808-827, 2018, (Pre SFI).
@article{Sjøvaag2018c,
title = {Is public service broadcasting a threat to commercial media?},
author = {Helle Sjøvaag and Truls André Pedersen and Thomas Owren},
url = {https://journals.sagepub.com/doi/pdf/10.1177/0163443718818354},
doi = {https://doi.org/10.1177/0163443718818354},
year = {2018},
date = {2018-12-20},
journal = {Media, Culture & Society},
volume = {46},
number = {1},
pages = {808-827},
abstract = {This article asks to what extent public service broadcasting’s online news service resembles that of commercial media. The context of this inquiry is claims of ‘out-crowding’ facing public service broadcasters across Europe. In Norway, commercial players in this debate accuse the public service broadcaster, NRK, of being too similar to competitors in the private sector for commercial operators to attain sustainable revenues in the online realm. To ascertain the extent to what these claims are warranted, this article compares NRK’s online content with that of nine commercial competitors in national and local markets, using a hybrid methodological approach combining quantitative content analysis with Latent Dirichlet allocation, analysing in excess of 115,000 documents. Findings show that commercial operators resemble each other more than they do NRK, indicating closer competition in the commercial segment than between the public service broadcaster and market players.},
note = {Pre SFI},
keywords = {},
pubstate = {published},
tppubtype = {article}
}
Dyrkolbotn, Sjur; Pedersen, Truls; Slavkovik, Marija
On the distinction between implicit and explicit ethical agency Conference
Proceedings of the 2018 AAAI/ACM Conference on AI, Ethics, and Society (AIES '18), 2018, (Pre SFI).
@conference{Dyrkolbotn2018,
title = {On the distinction between implicit and explicit ethical agency},
author = {Sjur Dyrkolbotn and Truls Pedersen and Marija Slavkovik},
url = {https://dl.acm.org/doi/pdf/10.1145/3278721.3278769},
doi = {https://doi.org/10.1145/3278721.3278769},
year = {2018},
date = {2018-12-01},
booktitle = {Proceedings of the 2018 AAAI/ACM Conference on AI, Ethics, and Society (AIES '18)},
pages = {74–80},
abstract = {With recent advances in artificial intelligence and the rapidly increasing importance of autonomous intelligent systems in society, it is becoming clear that artificial agents will have to be designed to comply with complex ethical standards. As we work to develop moral machines, we also push the boundaries of existing legal categories. The most pressing question is what kind of ethical decision-making our machines are actually able to engage in. Both in law and in ethics, the concept of agency forms a basis for further legal and ethical categorisations, pertaining to decision-making ability. Hence, without a cross-disciplinary understanding of what we mean by ethical agency in machines, the question of responsibility and liability cannot be clearly addressed. Here we make first steps towards a comprehensive definition, by suggesting ways to distinguish between implicit and explicit forms of ethical agency.},
note = {Pre SFI},
keywords = {},
pubstate = {published},
tppubtype = {conference}
}
Sjøvaag, Helle; Pedersen, Truls André
Female voices in the news: Structural conditions of gender representations in Norwegian newspapers Journal Article
In: Journalism & Mass Communication Quarterly, vol. 96, no. 1, pp. 215-238, 2018, (Pre SFI).
@article{Sjøvaag2018b,
title = {Female voices in the news: Structural conditions of gender representations in Norwegian newspapers},
author = {Helle Sjøvaag and Truls André Pedersen},
url = {https://journals.sagepub.com/doi/pdf/10.1177/1077699018789885},
doi = {https://doi.org/10.1177/1077699018789885},
year = {2018},
date = {2018-08-21},
journal = {Journalism & Mass Communication Quarterly},
volume = {96},
number = {1},
pages = {215-238},
abstract = {The article presents a hybrid analysis combining manual content analysis of 9,131 sources in 5,544 news stories across 75 publications, with computational gender recognition producing 551,102 names from 320,228 articles across 125 newspapers. The article investigates the significance of structural features for the presence of women in the news. Results show female sources are only equal to men as ordinary citizens and children, and only in lifestyle content. Among the structural features examined, only local distribution and a circulation less than 5,000 exhibit improvements in female representation. Ownership, distribution frequency, market position, and direct press support had little or no effect.},
note = {Pre SFI},
keywords = {},
pubstate = {published},
tppubtype = {article}
}
Sjøvaag, Helle; Stavelin, Eirik; Karlsson, Michael; Kammer, Aske
The hyperlinked Scandinavian news ecology: The unequal terms forged by the structural properties of digitalisation Journal Article
In: Digital Journalism, vol. 7, no. 4, pp. 507-531, 2018, (Pre SFI).
@article{Sjøvaag2018,
title = {The hyperlinked Scandinavian news ecology: The unequal terms forged by the structural properties of digitalisation},
author = {Helle Sjøvaag and Eirik Stavelin and Michael Karlsson and Aske Kammer},
url = {https://www.tandfonline.com/doi/epub/10.1080/21670811.2018.1454335?needAccess=true},
doi = {https://doi.org/10.1080/21670811.2018.1454335},
year = {2018},
date = {2018-04-06},
journal = {Digital Journalism},
volume = {7},
number = {4},
pages = {507-531},
abstract = {The article presents a network analysis of 22,861,013 geocoded external hyperlinks, collected from 230 Danish, 220 Norwegian and 208 Swedish news websites in 2016. The analysis asks what the structural properties of the Scandinavian media systems—including its geography and ownership structures—mean for news outlets’ centrality within the hyperlinked news ecology. The analysis finds that whereas incumbent legacy media occupy central positions, about one third of the network is absent from the hyperlinked interaction, primarily local, independently owned newspapers. A multiple linear regression analysis shows that national distribution and corporate ownership correlates to network centrality more than other predictors. As brokers in the network consist of the large, legacy, capital-based news organisations, hyperlink connectivity is primarily characterised by proximity to the centres of power, corporate ownership, agenda setting incumbency and national distribution.},
note = {Pre SFI},
keywords = {},
pubstate = {published},
tppubtype = {article}
}
Ludewig, Malte; Jannach, Dietmar
Evaluation of Session-based Recommendation Algorithms Journal Article
In: User-Modeling and User-Adapted Interaction, vol. 28, no. 4-5, pp. 331-390, 2018, (Pre SFI).
@article{Ludewig2018,
title = {Evaluation of Session-based Recommendation Algorithms},
author = {Malte Ludewig and Dietmar Jannach},
url = {https://arxiv.org/pdf/1803.09587.pdf},
doi = {10.1007/s11257-018-9209-6},
year = {2018},
date = {2018-03-26},
journal = {User-Modeling and User-Adapted Interaction},
volume = {28},
number = {4-5},
pages = {331-390},
abstract = {Recommender systems help users find relevant items of interest, for example on e-commerce or media streaming sites. Most academic research is concerned with approaches that personalize the recommendations according to long-term user profiles. In many real-world applications, however, such long-term profiles often do not exist and recommendations therefore have to be made solely based on the observed behavior of a user during an ongoing session. Given the high practical relevance of the problem, an increased interest in this problem can be observed in recent years, leading to a number of proposals for session-based recommendation algorithms that typically aim to predict the user's immediate next actions. In this work, we present the results of an in-depth performance comparison of a number of such algorithms, using a variety of datasets and evaluation measures. Our comparison includes the most recent approaches based on recurrent neural networks like GRU4REC, factorized Markov model approaches such as FISM or FOSSIL, as well as simpler methods based, e.g., on nearest neighbor schemes. Our experiments reveal that algorithms of this latter class, despite their sometimes almost trivial nature, often perform equally well or significantly better than today's more complex approaches based on deep neural networks. Our results therefore suggest that there is substantial room for improvement regarding the development of more sophisticated session-based recommendation algorithms.},
note = {Pre SFI},
keywords = {},
pubstate = {published},
tppubtype = {article}
}
Quadrana, Massimo; Cremonesi, Paolo; Jannach, Dietmar
Sequence-Aware Recommender Systems Journal Article
In: ACM Computing Surveys, vol. 51, no. 4, pp. 1-35, 2018, (Pre SFI).
@article{Quadrana2018,
title = {Sequence-Aware Recommender Systems},
author = {Massimo Quadrana and Paolo Cremonesi and Dietmar Jannach},
url = {https://arxiv.org/pdf/1802.08452.pdf},
year = {2018},
date = {2018-02-23},
journal = {ACM Computing Surveys},
volume = {51},
number = {4},
pages = {1-35},
abstract = {Recommender systems are one of the most successful applications of data mining and machine learning technology in practice. Academic research in the field is historically often based on the matrix completion problem formulation, where for each user-item-pair only one interaction (e.g., a rating) is considered. In many application domains, however, multiple user-item interactions of different types can be recorded over time. And, a number of recent works have shown that this information can be used to build richer individual user models and to discover additional behavioral patterns that can be leveraged in the recommendation process. In this work we review existing works that consider information from such sequentially-ordered user- item interaction logs in the recommendation process. Based on this review, we propose a categorization of the corresponding recommendation tasks and goals, summarize existing algorithmic solutions, discuss methodological approaches when benchmarking what we call sequence-aware recommender systems, and outline open challenges in the area.},
note = {Pre SFI},
keywords = {},
pubstate = {published},
tppubtype = {article}
}
Zhang, Shuo; Balog, Krisztian
Ad Hoc Table Retrieval using Semantic Similarity Conference
Proceedings of The Web Conference 2018 (WWW’18), 2018, (Pre SFI).
@conference{Zhang2018,
title = {Ad Hoc Table Retrieval using Semantic Similarity},
author = {Shuo Zhang and Krisztian Balog},
url = {https://arxiv.org/pdf/1802.06159.pdf},
doi = {10.1145/3178876.3186067},
year = {2018},
date = {2018-02-16},
booktitle = {Proceedings of The Web Conference 2018 (WWW’18)},
pages = {1553-1562},
abstract = {We introduce and address the problem of ad hoc table retrieval: answering a keyword query with a ranked list of tables. This task is not only interesting on its own account, but is also being used as a core component in many other table-based information access scenarios, such as table completion or table mining. The main novel contribution of this work is a method for performing semantic matching between queries and tables. Specifically, we (i) represent queries and tables in multiple semantic spaces (both discrete sparse and continuous dense vector representations) and (ii) introduce various similarity measures for matching those semantic representations. We consider all possible combinations of semantic representations and similarity measures and use these as features in a supervised learning model. Using a purpose-built test collection based on Wikipedia tables, we demonstrate significant and substantial improvements over a state-of-the-art baseline.},
note = {Pre SFI},
keywords = {},
pubstate = {published},
tppubtype = {conference}
}
Balog, Krisztian
1, Springer, 2018, ISBN: 978-3-319-93935-3, (Pre SFI).
@book{Balog2018,
title = {Entity-Oriented Search},
author = {Krisztian Balog},
url = {https://www.springer.com/gp/book/9783319939339},
doi = {10.1007/978-3-319-93935-3},
isbn = {978-3-319-93935-3},
year = {2018},
date = {2018-01-01},
volume = {39},
publisher = {Springer},
edition = {1},
abstract = {This open access book covers all facets of entity-oriented search—where “search” can be interpreted in the broadest sense of information access—from a unified point of view, and provides a coherent and comprehensive overview of the state of the art. It represents the first synthesis of research in this broad and rapidly developing area. Selected topics are discussed in-depth, the goal being to establish fundamental techniques and methods as a basis for future research and development. Additional topics are treated at a survey level only, containing numerous pointers to the relevant literature. A roadmap for future research, based on open issues and challenges identified along the way, rounds out the book.},
note = {Pre SFI},
keywords = {},
pubstate = {published},
tppubtype = {book}
}
2017
Elahi, Mehdi; Deldjoo, Yashar; Moghaddam, Farshad Bakhshandegan; Cella, Leonardo; Cerada, Stefano; Cremonesi, Paolo
Exploring the semantic gap for movie recommendations Conference
Proceedings of the Eleventh ACM Conference on Recommender Systems, Association for Computing Machinery New York, 2017, (Pre SFI).
@conference{Elahi2017,
title = {Exploring the semantic gap for movie recommendations},
author = {Mehdi Elahi and Yashar Deldjoo and Farshad Bakhshandegan Moghaddam and Leonardo Cella and Stefano Cerada and Paolo Cremonesi },
url = {https://dl.acm.org/doi/pdf/10.1145/3109859.3109908},
doi = {https://doi.org/10.1145/3109859.3109908},
year = {2017},
date = {2017-08-01},
booktitle = {Proceedings of the Eleventh ACM Conference on Recommender Systems},
pages = {326–330},
address = {New York},
organization = {Association for Computing Machinery},
abstract = {In the last years, there has been much attention given to the semantic gap problem in multimedia retrieval systems. Much effort has been devoted to bridge this gap by building tools for the extraction of high-level, semantics-based features from multimedia content, as low-level features are not considered useful because they deal primarily with representing the perceived content rather than the semantics of it.
In this paper, we explore a different point of view by leveraging the gap between low-level and high-level features. We experiment with a recent approach for movie recommendation that extract low-level Mise-en-Scéne features from multimedia content and combine it with high-level features provided by the wisdom of the crowd.
To this end, we first performed an offline performance assessment by implementing a pure content-based recommender system with three different versions of the same algorithm, respectively based on (i) conventional movie attributes, (ii) mise-en-scene features, and (iii) a hybrid method that interleaves recommendations based on movie attributes and mise-en-scene features. In a second study, we designed an empirical study involving 100 subjects and collected data regarding the quality perceived by the users. Results from both studies show that the introduction of mise-en-scéne features in conjunction with traditional movie attributes improves both offline and online quality of recommendations.},
note = {Pre SFI},
keywords = {},
pubstate = {published},
tppubtype = {conference}
}
In this paper, we explore a different point of view by leveraging the gap between low-level and high-level features. We experiment with a recent approach for movie recommendation that extract low-level Mise-en-Scéne features from multimedia content and combine it with high-level features provided by the wisdom of the crowd.
To this end, we first performed an offline performance assessment by implementing a pure content-based recommender system with three different versions of the same algorithm, respectively based on (i) conventional movie attributes, (ii) mise-en-scene features, and (iii) a hybrid method that interleaves recommendations based on movie attributes and mise-en-scene features. In a second study, we designed an empirical study involving 100 subjects and collected data regarding the quality perceived by the users. Results from both studies show that the introduction of mise-en-scéne features in conjunction with traditional movie attributes improves both offline and online quality of recommendations.
2016
Jannach, Dietmar; Tuzhilin, Alexander; Zanker, Markus
Recommender Systems - Beyond Matrix Completion Journal Article
In: Communications of the ACM, vol. 59, no. 11, pp. 94-102, 2016, (Pre SFI).
@article{Jannach2016,
title = {Recommender Systems - Beyond Matrix Completion},
author = {Dietmar Jannach and Alexander Tuzhilin and Markus Zanker },
url = {https://www.researchgate.net/publication/309600906_Recommender_systems---_beyond_matrix_completion},
doi = {10.1145/2891406},
year = {2016},
date = {2016-10-01},
journal = {Communications of the ACM},
volume = {59},
number = {11},
pages = {94-102},
abstract = {Recommender systems have become a natural part of the user experience in today's online world. These systems are able to deliver value both for users and providers and are one prominent example where the output of academic research has a direct impact on the advancements in industry. In this article, we have briefy reviewed the history of this multidis-ciplinary field and looked at recent efforts in the research community to consider the variety of factors that may influence the long-term success of a recommender system. The list of open issues and success factors is still far from complete and new challenges arise constantly that require further research. For example, the huge amounts of user data and preference signals that become available through the Social Web and the Internet of Things not only leads to technical challenges such as scalability, but also to societal questions concerning user privacy. Based on our reflections on the developments in the field, we finally emphasize the need for a more holistic research approach that combines the insights of different disciplines. We urge that research focuses even more on practical problems that matter and are truly suited to increase the utility of recommendations from the viewpoint of the users.
},
note = {Pre SFI},
keywords = {},
pubstate = {published},
tppubtype = {article}
}
Elahi, Mehdi; Ricci, Francesco; Rubens, Neil
A survey of active learning in collaborative filtering recommender systems Journal Article
In: Computer Science Review, vol. 20, pp. 29-50, 2016, (Pre SFI).
@article{Elahi2016,
title = {A survey of active learning in collaborative filtering recommender systems},
author = {Mehdi Elahi and Francesco Ricci and Neil Rubens},
url = {https://reader.elsevier.com/reader/sd/pii/S1574013715300150?token=EA12A462FC07F42733F4F13375217A57D3FDC7F6047C133156CB1F4E4487DF24C5366547DF4530A25942F690233F2E30},
doi = {10.1016/j.cosrev.2016.05.002},
year = {2016},
date = {2016-06-02},
journal = {Computer Science Review},
volume = {20},
pages = {29-50},
abstract = {In collaborative filtering recommender systems user’s preferences are expressed as ratings for items, and each additional rating extends the knowledge of the system and affects the system’s recommendation accuracy. In general, the more ratings are elicited from the users, the more effective the recommendations are. However, the usefulness of each rating may vary significantly, i.e., different ratings may bring a different amount and type of information about the user’s tastes. Hence, specific techniques, which are defined as “active learning strategies”, can be used to selectively choose the items to be presented to the user for rating. In fact, an active learning strategy identifies and adopts criteria for obtaining data that better reflects users’ preferences and enables to generate better recommendations.
So far, a variety of active learning strategies have been proposed in the literature. In this article, we survey recent strategies by grouping them with respect to two distinct dimensions: personalization, i.e., whether the system selected items are different for different users or not, and, hybridization, i.e., whether active learning is guided by a single criterion (heuristic) or by multiple criteria. In addition, we present a comprehensive overview of the evaluation methods and metrics that have been employed by the research community in order to test active learning strategies for collaborative filtering. Finally, we compare the surveyed strategies and provide guidelines for their usage in recommender systems.},
note = {Pre SFI},
keywords = {},
pubstate = {published},
tppubtype = {article}
}
So far, a variety of active learning strategies have been proposed in the literature. In this article, we survey recent strategies by grouping them with respect to two distinct dimensions: personalization, i.e., whether the system selected items are different for different users or not, and, hybridization, i.e., whether active learning is guided by a single criterion (heuristic) or by multiple criteria. In addition, we present a comprehensive overview of the evaluation methods and metrics that have been employed by the research community in order to test active learning strategies for collaborative filtering. Finally, we compare the surveyed strategies and provide guidelines for their usage in recommender systems.
Tobias, Ignacio Fernandez; Braunhofer, Matthias; Elahi, Mehdi; Ricci, Francesco; Cantador, Ivan
Alleviating the new user problem in collaborative filtering by exploiting personality information Journal Article
In: User Modeling and User-Adapted Interaction, vol. 26, no. 2-3, pp. 221-255, 2016, (Pre SFI).
@article{Tobias2016,
title = {Alleviating the new user problem in collaborative filtering by exploiting personality information},
author = {Ignacio Fernandez Tobias and Matthias Braunhofer and Mehdi Elahi and Francesco Ricci and Ivan Cantador},
url = {https://www.researchgate.net/publication/285574429_Alleviating_the_New_User_Problem_in_Collaborative_Filtering_by_Exploiting_Personality_Information},
doi = {10.1007/s11257-016-9172-z},
year = {2016},
date = {2016-06-01},
journal = {User Modeling and User-Adapted Interaction},
volume = {26},
number = {2-3},
pages = {221-255},
abstract = {The new user problem in recommender systems is still challenging, and there is not yet a unique solution that can be applied in any domain or situation. In this paper we analyze viable solutions to the new user problem in collaborative filtering (CF) that are based on the exploitation of user personality information: (a) personality-based CF, which directly improves the recommendation prediction model by incorporating user personality information, (b) personality-based active learning, which utilizes personality information for identifying additional useful preference data in the target recommendation domain to be elicited from the user, and (c) personality-based cross-domain recommendation, which exploits personality information to better use user preference data from auxiliary domains which can be used to compensate the lack of user preference data in the target domain. We benchmark the effectiveness of these methods on large datasets that span several domains, namely movies, music and books. Our results show that personality-aware methods achieve performance improvements that range from 6 to 94 % for users completely new to the system, while increasing the novelty of the recommended items by 3–40 % with respect to the non-personalized popularity baseline. We also discuss the limitations of our approach and the situations in which the proposed methods can be better applied, hence providing guidelines for researchers and practitioners in the field.},
note = {Pre SFI},
keywords = {},
pubstate = {published},
tppubtype = {article}
}
2015
Rubens, Neil; Elahi, Mehdi; Sugiyama, Masashi; Kaplan, Dain
Active learning in recommender systems Book Chapter
In: Ricci, Francesco; Rokach, Lior; Shapira, Bracha (Ed.): pp. 809-846, Springer, 2015, ISBN: 978-1-4899-7637-6, (Pre SFI).
@inbook{Rubens2015,
title = {Active learning in recommender systems},
author = {Neil Rubens and Mehdi Elahi and Masashi Sugiyama and Dain Kaplan},
editor = {Francesco Ricci and Lior Rokach and Bracha Shapira},
url = {https://link.springer.com/chapter/10.1007/978-1-4899-7637-6_24},
doi = {10.1007/978-1-4899-7637-6_24},
isbn = {978-1-4899-7637-6},
year = {2015},
date = {2015-01-01},
pages = {809-846},
publisher = {Springer},
abstract = {In Recommender Systems (RS), a user’s preferences are expressed in terms of rated items, where incorporating each rating may improve the RS’s predictive accuracy. In addition to a user rating items at-will (a passive process), RSs may also actively elicit the user to rate items, a process known as Active Learning (AL). However, the number of interactions between the RS and the user is still limited. One aim of AL is therefore the selection of items whose ratings are likely to provide the most information about the user’s preferences. In this chapter, we provide an overview of AL within RSs, discuss general objectives and considerations, and then summarize a variety of methods commonly employed. AL methods are categorized based on our interpretation of their primary motivation/goal, and then sub-classified into two commonly classified types, instance-based and model-based, for easier comprehension. We conclude the chapter by outlining ways in which AL methods could be evaluated, and provide a brief summary of methods performance.},
note = {Pre SFI},
keywords = {},
pubstate = {published},
tppubtype = {inbook}
}
0000
Abdollahpouri, Himan; Elahi, Mehdi; Mansoury, Masoud; Sahebi, Shaghayegh; Nazari, Zahra; Chaney, Allison; Loni, Babak
MORS 2021: 1st Workshop on Multi-Objective Recommender Systems. Proceedings
0000.
@proceedings{Abdollahpouri2021,
title = {MORS 2021: 1st Workshop on Multi-Objective Recommender Systems.},
author = {Himan Abdollahpouri and Mehdi Elahi and Masoud Mansoury and Shaghayegh Sahebi and Zahra Nazari and Allison Chaney and Babak Loni},
journal = {In Fifteenth ACM Conference on Recommender Systems.},
pages = {787-788},
keywords = {},
pubstate = {published},
tppubtype = {proceedings}
}
Find us
Lars Hilles gate 30
5008 Bergen
Norway
Contact us
MediaFutures
Office@mediafutures.no
Responsible Editor:
Centre Director Prof. Dr. Christoph Trattner
Christoph.Trattner@uib.no
To learn more about the University of Bergen's cookie policy, see the link below.
Copyright © University of Bergen 2024