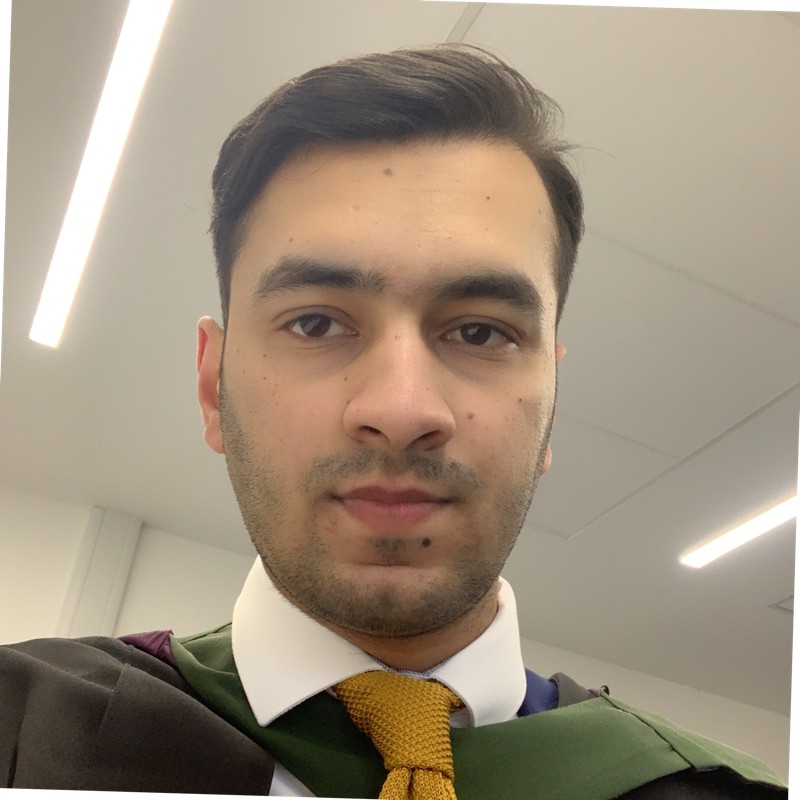
Sohail Ahmed Khan
PhD Candidate
2024
Khan, Sohail Ahmed; Dierickx, Laurence; Furuly, Jan-Gunnar; Vold, Henrik Brattli; Tahseen, Rano; Linden, Carl-Gustav; Dang-Nguyen, Duc-Tien
Debunking War Information Disorder: A Case Study in Assessing the Use of Multimedia Verification Tools Journal Article
In: Journal of the Association for Information Science and Technology, 2024.
@article{warinfo24,
title = {Debunking War Information Disorder: A Case Study in Assessing the Use of Multimedia Verification Tools},
author = {Sohail Ahmed Khan and Laurence Dierickx and Jan-Gunnar Furuly and Henrik Brattli Vold and Rano Tahseen and Carl-Gustav Linden and Duc-Tien Dang-Nguyen},
url = {https://mediafutures.no/jasist_verifyingwarconflict-compressed/},
year = {2024},
date = {2024-11-11},
journal = {Journal of the Association for Information Science and Technology},
abstract = {This paper investigates the use of multimedia verification, in particular, computational tools and Open-source Intelligence (OSINT) methods, for verifying online multimedia content in the context of the ongoing wars in Ukraine and Gaza. Our study examines the workflows and tools used by several fact-checkers and journalists working at Faktisk, a Norwegian fact-checking organisation. Our study showcases the effectiveness of diverse resources, including AI tools, geolocation tools, internet archives, and social media monitoring platforms, in enabling journalists and fact-checkers to efficiently process and corroborate evidence, ensuring the dissemination of accurate information. This research provides an in-depth analysis of the role of computational tools and OSINT methods for multimedia verification. It also underscores the potentials of currently available technology, and highlights its limitations while providing guidance for future development of digital multimedia verification tools and frameworks.},
keywords = {},
pubstate = {published},
tppubtype = {article}
}
Majjodi, Ayoub El; Khan, Sohail Ahmed; Starke, Alain D.; Elahi, Mehdi; Trattner, Christoph
Advancing Visual Food Attractiveness Predictions for Healthy Food Recommender Systems Conference
The ACM Conference on Recommender Systems (RecSys) 2024, 2024.
@conference{visualfood24,
title = {Advancing Visual Food Attractiveness Predictions for Healthy Food Recommender Systems},
author = {Ayoub El Majjodi and Sohail Ahmed Khan and Alain D. Starke and Mehdi Elahi and Christoph Trattner},
url = {https://mediafutures.no/healthrecsys-2024-ayoub-1/},
year = {2024},
date = {2024-09-17},
booktitle = {The ACM Conference on Recommender Systems (RecSys) 2024},
abstract = {The visual representation of food has a significant influence on
how people choose food in the real world but also in a digital food
recommender scenario. Previous studies on that matter show that
small change in visual features can change human decision-making,
regardless of whether the food is healthy or not. This paper reports
on a study that aims to understand further how users perceive
the attractiveness of food images in the digital world. In an online
mixed-methods survey (N=192), users provided visual attractive-
ness ratings on a 7-point scale and provided textual assessments
of the visual attractiveness of food images. We found a robust
correlation between fundamental visual features (e.g., contrast, col-
orfulness) and perceived image attractiveness. The analysis also
revealed that cooking skills predicted food image attractiveness
among user factors. Regarding food image dimensions, appearance
and perceived healthiness emerged to be significantly correlated
with user ratings for food image attractiveness.},
keywords = {},
pubstate = {published},
tppubtype = {conference}
}
how people choose food in the real world but also in a digital food
recommender scenario. Previous studies on that matter show that
small change in visual features can change human decision-making,
regardless of whether the food is healthy or not. This paper reports
on a study that aims to understand further how users perceive
the attractiveness of food images in the digital world. In an online
mixed-methods survey (N=192), users provided visual attractive-
ness ratings on a 7-point scale and provided textual assessments
of the visual attractiveness of food images. We found a robust
correlation between fundamental visual features (e.g., contrast, col-
orfulness) and perceived image attractiveness. The analysis also
revealed that cooking skills predicted food image attractiveness
among user factors. Regarding food image dimensions, appearance
and perceived healthiness emerged to be significantly correlated
with user ratings for food image attractiveness.
Dang-Nguyen, Duc-Tien; Khan, Sohail Ahmed; Riegler, Michael; Halvorsen, Pål; Tran, Anh-Duy; Dao, Minh-Son; Tran, Minh-Triet
Overview of the Grand Challenge on Detecting Cheapfakes Proceedings Article
In: Proceedings of the 2024 International Conference on Multimedia Retrieval (ICMR’24), 2024.
@inproceedings{deteccheapfakes,
title = {Overview of the Grand Challenge on Detecting Cheapfakes},
author = {Duc-Tien Dang-Nguyen and Sohail Ahmed Khan and Michael Riegler and Pål Halvorsen and Anh-Duy Tran and Minh-Son Dao and Minh-Triet Tran},
url = {https://mediafutures.no/grand_challenge_on_detecting_cheapfakes-2/},
year = {2024},
date = {2024-06-10},
urldate = {2024-06-10},
booktitle = {Proceedings of the 2024 International Conference on Multimedia Retrieval (ICMR’24)},
abstract = {Cheapfake is a recently coined term that encompasses non-AI ("cheap") manipulations of multimedia content. Cheapfakes are known to be more prevalent than deepfakes. Cheapfake media can be created using editing software for image/video manipulations, or even without using any software, by simply altering the context of an image/video by sharing the media alongside misleading claims. This alteration of context is referred to as out-of-context (OOC) misuse of media. OOC media is much harder to detect than fake media, since the images and videos are not tampered. In this challenge, we focus on detecting OOC images, and more specifically the misuse of real photographs with conflicting image captions in news items. The aim of this challenge is to develop and benchmark models that can be used to detect whether given samples (news image and associated captions) are OOC, based on the recently compiled COSMOS dataset.},
keywords = {},
pubstate = {published},
tppubtype = {inproceedings}
}
Khan, Sohail Ahmed; Dang-Nguyen, Duc-Tien
CLIPping the Deception: Adapting Vision-Language Models for Universal Deepfake Detection Conference
ACM International Conference on Multimedia Retrieval (ICMR), 2024.
@conference{CLIpingSohail24,
title = {CLIPping the Deception: Adapting Vision-Language Models for Universal Deepfake Detection},
author = {Sohail Ahmed Khan and Duc-Tien Dang-Nguyen},
url = {https://mediafutures.no/icmr24/},
year = {2024},
date = {2024-04-07},
booktitle = {ACM International Conference on Multimedia Retrieval (ICMR)},
journal = {ACM International Conference on Multimedia Retrieval (ICMR)},
abstract = {The recent advancements in Generative Adversarial Networks (GANs) and the emergence of Diffusion models have significantly streamlined the production of highly realistic and widely accessible synthetic content. As a result, there is a pressing need for effective general purpose detection mechanisms to mitigate the potential risks posed by deepfakes. In this paper, we explore the effectiveness of pre-trained vision-language models (VLMs) when paired with recent adaptation methods for universal deepfake detection. Following previous studies in this domain, we employ only a single dataset (ProGAN) in order to adapt CLIP for deepfake detection. However, in contrast to prior research, which rely solely on the visual part of CLIP while ignoring its textual component, our analysis reveals that retaining the text part is crucial. Consequently, the simple and lightweight Prompt Tuning based adaptation strategy that we employ outperforms the previous SOTA approach by 5.01% mAP and 6.61% accuracy while utilizing less than one third of the training data (200k images as compared to 720k). To assess the real-world applicability of our proposed models, we conduct a comprehensive evaluation across various scenarios. This involves rigorous testing on images sourced from 21 distinct datasets, including those generated by GANs-based, Diffusion-based and Commercial tools.},
keywords = {},
pubstate = {published},
tppubtype = {conference}
}
2023
Khan, Sohail Ahmed; Dang-Nguyen, Duc-Tien
IEEE Access, 2023.
@conference{deepfakede,
title = {Deepfake Detection: Analysing Model Generalisation Across Architectures, Datasets and Pre-Training Paradigms},
author = {Sohail Ahmed Khan and Duc-Tien Dang-Nguyen},
url = {https://mediafutures.no/ieee_access_2023___deepfake_comparative_analysis/},
year = {2023},
date = {2023-12-15},
booktitle = {IEEE Access},
abstract = {As deepfake technology gains traction, the need for reliable detection systems is crucial. Recent research has introduced various deep learning-based detection systems, yet they exhibit limitations in generalizing effectively across diverse data distributions that differ from the training data. Our study focuses on understanding the generalization challenges by exploring specific aspects such as deep learning model architecture, pre-training strategy and datasets. Through a comprehensive comparative analysis, we evaluate multiple supervised and self-supervised deep learning models for deepfake detection.
Specifically, we evaluate eight supervised deep learning architectures and two transformer-based models pre-trained using self-supervised strategies (DINO, CLIP) on four different deepfake detection benchmarks (FakeAVCeleb, CelebDF-V2, DFDC and FaceForensics++). Our analysis includes intra-dataset and inter-dataset evaluations, examining the best performing models, generalisation capabilities and impact of augmentations. We also investigate the trade-off between model size, efficiency and performance. Our main goal is to provide insights into the effectiveness of different deep learning architectures (transformers, CNNs), training strategies (supervised, self-supervised) and deepfake detection benchmarks. Through our extensive analysis, we established that Transformer models outperform CNN models in deepfake detection. Also, we show that FaceForensics++ and DFDC datasets equip models with comparably better generalisation capabilities, as compared to FakeAVCeleb and CelebDF-V2 datasets. Our analysis also show that image augmentations can be helpful in achieving better performance, at least for the Transformer models.},
keywords = {},
pubstate = {published},
tppubtype = {conference}
}
Specifically, we evaluate eight supervised deep learning architectures and two transformer-based models pre-trained using self-supervised strategies (DINO, CLIP) on four different deepfake detection benchmarks (FakeAVCeleb, CelebDF-V2, DFDC and FaceForensics++). Our analysis includes intra-dataset and inter-dataset evaluations, examining the best performing models, generalisation capabilities and impact of augmentations. We also investigate the trade-off between model size, efficiency and performance. Our main goal is to provide insights into the effectiveness of different deep learning architectures (transformers, CNNs), training strategies (supervised, self-supervised) and deepfake detection benchmarks. Through our extensive analysis, we established that Transformer models outperform CNN models in deepfake detection. Also, we show that FaceForensics++ and DFDC datasets equip models with comparably better generalisation capabilities, as compared to FakeAVCeleb and CelebDF-V2 datasets. Our analysis also show that image augmentations can be helpful in achieving better performance, at least for the Transformer models.
Sheikhi, Ghazaal; Touileb, Samia; Khan, Sohail Ahmed
2023.
@conference{Sheikhi2023,
title = {Automated Claim Detection for Fact-checking: A Case Study using Norwegian Pre-trained Language Models},
author = {Ghazaal Sheikhi and Samia Touileb and Sohail Ahmed Khan },
url = {https://mediafutures.no/2023_nodalida-1_1/},
year = {2023},
date = {2023-05-24},
urldate = {2023-05-24},
abstract = {We investigate to what extent pre-trained language models can be used for automated claim detection for fact-checking in a low resource setting. We explore this idea by fine-tuning four Norwegian pre-trained language models to perform the binary classification task of determining if a claim should be discarded or upheld to be further processed by human fact-checkers. We conduct a set of experiments to compare the performance of the language models, and provide a simple baseline model using SVM with tf-idf features. Since we are focusing on claim detection, the recall score for the upheld class is to be emphasized over other performance measures. Our experiments indicate that the language models are superior to the baseline system in terms of F1, while the baseline model results in the highest precision. However, the two Norwegian models, NorBERT2 and NB-BERT_large, give respectively superior F1 and recall values. We argue that large language models could be successfully employed to solve the automated claim detection problem. The choice of the model depends on the desired end-goal. Moreover, our error analysis shows that language models are generally less sensitive to the changes in claim length and source than the SVM model.},
keywords = {},
pubstate = {published},
tppubtype = {conference}
}
Khan, Sohail Ahmed; Sheikhi, Ghazaal; Opdahl, Andreas L.; Rabbi, Fazle; Stoppel, Sergej; Trattner, Christoph; Dang-Nguyen, Duc-Tien
Visual User-Generated Content Verification in Journalism: An Overview Journal Article
In: IEEE Access, 2023.
@article{KHAN2023,
title = {Visual User-Generated Content Verification in Journalism: An Overview},
author = {Sohail Ahmed Khan and Ghazaal Sheikhi and Andreas L. Opdahl and Fazle Rabbi and Sergej Stoppel and Christoph Trattner and Duc-Tien Dang-Nguyen},
url = {https://mediafutures.no/e0ret1-visual_user-generated_content_verification_in_journalism_an_overview/},
year = {2023},
date = {2023-01-16},
urldate = {2023-01-16},
journal = {IEEE Access},
abstract = {Over the past few years, social media has become an indispensable part of the news generation and dissemination cycle on the global stage. These digital channels along with the easy-to-use editing tools have unfortunately created a medium for spreading mis-/disinformation containing visual content. Media practitioners and fact-checkers continue to struggle with scrutinising and debunking visual user-generated content (UGC) quickly and thoroughly as verification of visual content requires a high level of expertise and could be exceedingly complex amid the existing computational tools employed in newsrooms. The aim of this study is to present a forward-looking perspective on how visual UGC verification in journalism can be transformed by multimedia forensics research. We elaborate on a comprehensive overview of the five elements of the UGC verification and propose multimedia forensics as the sixth element. In addition, different types of visual content forgeries and detection approaches proposed by the computer science research community are explained. Finally, a mapping of the available verification tools media practitioners rely on is created along with their limitations and future research directions to gain the confidence of media professionals in using multimedia forensics tools in their day-to-day routine.},
keywords = {},
pubstate = {published},
tppubtype = {article}
}
2022
Khan, Sohail Ahmed; Dang-Nguyen, Duc-Tien
Hybrid Transformer Network for Deepfake Detection Conference
Hybrid Transformer Network for Deepfake Detection, 2022.
@conference{Khan2022,
title = {Hybrid Transformer Network for Deepfake Detection},
author = {Sohail Ahmed Khan and Duc-Tien Dang-Nguyen},
url = {https://mediafutures.no/2208-05820-compressed-2/},
year = {2022},
date = {2022-08-11},
booktitle = {Hybrid Transformer Network for Deepfake Detection},
keywords = {},
pubstate = {published},
tppubtype = {conference}
}