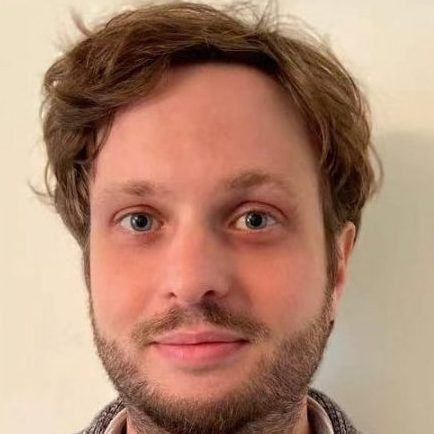
Pete Andrews
PhD Candidate
University of Bergen
Pete Andrews is a Ph.D. candidate in WP4 Media Content Interaction & Accessibility. He studied an MSc in Advanced Multimedia Design and 3D technologies at Brunel University, London. After completing his MSc, he studied courses in Data Science and Data mining at the Chinese Academy of Sciences, Beijing. Peter has a professional background in medical imaging and multimedia. During this time he was a member of the Academy for Healthcare Sciences (AHCS) and institute of Medical Illustrators (IMI).
2024
Andrews, Peter; Nordberg, Oda Elise; Guribye, Frode; Fjeld, Morten; Borch, Njål
Designing for Automated Sports Commentary Systems Conference
IMX'24, 2024.
@conference{designing_for_automated24,
title = {Designing for Automated Sports Commentary Systems},
author = {Peter Andrews and Oda Elise Nordberg and Frode Guribye and Morten Fjeld and Njål Borch },
url = {https://mediafutures.no/designing_for_automated_sports_commentary_systems-2/},
year = {2024},
date = {2024-06-12},
booktitle = {IMX'24},
abstract = {Advancements in Natural Language Processing (NLP) and Computer Vision (CV) are revolutionizing how we experience sports broadcasting. Traditionally, sports commentary has played a crucial role in enhancing viewer understanding and engagement with live games. Yet, the prospects of automated commentary, especially in light of these technological advancements and their impact on viewers’ experience, remain largely unexplored. This paper elaborates upon an innovative automated commentary system that integrates NLP and CV to provide a multimodal experience, combining auditory feedback through text-to-speech and visual cues, known as italicizing, for real-time in-game commentary. The system supports color commentary, which aims to inform the viewer of information surrounding the game by pulling additional content from a database. Moreover, it also supports play-by-play commentary covering in-game developments derived from an event system based on CV. As the system reinvents the role of commentary in sports video, we must consider the design and implications of multimodal artificial commentators. A focused user study with eight participants aimed at understanding the design implications of such multimodal artificial commentators reveals critical insights. Key findings emphasize the importance of language precision, content relevance, and delivery style in automated commentary, underscoring the necessity for personalization to meet diverse viewer preferences. Our results validate the potential value and effectiveness of multimodal feedback and derive design considerations, particularly in personalizing content to revolutionize the role of commentary in sports broadcasts.},
keywords = {},
pubstate = {published},
tppubtype = {conference}
}
Advancements in Natural Language Processing (NLP) and Computer Vision (CV) are revolutionizing how we experience sports broadcasting. Traditionally, sports commentary has played a crucial role in enhancing viewer understanding and engagement with live games. Yet, the prospects of automated commentary, especially in light of these technological advancements and their impact on viewers’ experience, remain largely unexplored. This paper elaborates upon an innovative automated commentary system that integrates NLP and CV to provide a multimodal experience, combining auditory feedback through text-to-speech and visual cues, known as italicizing, for real-time in-game commentary. The system supports color commentary, which aims to inform the viewer of information surrounding the game by pulling additional content from a database. Moreover, it also supports play-by-play commentary covering in-game developments derived from an event system based on CV. As the system reinvents the role of commentary in sports video, we must consider the design and implications of multimodal artificial commentators. A focused user study with eight participants aimed at understanding the design implications of such multimodal artificial commentators reveals critical insights. Key findings emphasize the importance of language precision, content relevance, and delivery style in automated commentary, underscoring the necessity for personalization to meet diverse viewer preferences. Our results validate the potential value and effectiveness of multimodal feedback and derive design considerations, particularly in personalizing content to revolutionize the role of commentary in sports broadcasts.
Andrews, Peter; Borch, Njål; Fjeld, Morten
ACM ICMIP, 2024.
@conference{Footyvision1,
title = {FootyVision: Multi-Object Tracking, Localisation, and Augmentation of Players and Ball in Football Video},
author = {Peter Andrews and Njål Borch and Morten Fjeld},
url = {https://mediafutures.no/peterandrews-footyvision-icmip24-final/},
year = {2024},
date = {2024-04-20},
booktitle = {ACM ICMIP},
abstract = {Football video content analysis is a rapidly evolving field aiming to enrich the viewing experience of football matches. Current research often focuses on specific tasks like player and/or ball detection, tracking, and localisation in top-down views. Our study strives to integrate these efforts into a comprehensive Multi-Object Tracking (MOT) model capable of handling perspective transformations. Our framework, FootyVision, employs a YOLOv7 backbone trained on an extended player and ball dataset. The MOT module builds a gallery and assigns identities via the Hungarian algorithm based on feature embeddings, bounding box intersection over union, distance, and velocity. A novel component of our model is the perspective transformation module that leverages activation maps from the YOLOv7 backbone to compute homographies using lines, intersection points, and ellipses. This method effectively adapts to dynamic and uncalibrated video data, even in viewpoints with limited visual information. In terms of performance, FootyVision sets new benchmarks. The model achieves a mean average precision (mAP) of 95.7% and an F1-score of 95.5% in object detection. For MOT, it demonstrates robust capabilities, with an IDF1 score of approximately 93% on both ISSIA and SoccerNet datasets. For SoccerNet, it reaches a MOTA of 94.04% and shows competitive results for ISSIA. Additionally, FootyVision scores a HOTA(0) of 93.1% and an overall HOTA of 72.16% for the SoccerNet dataset. Our ablation study confirms the effectiveness of the selected tracking features and identifies key attributes for further improvement. While the model excels in maintaining track accuracy throughout the testing dataset, we recognise the potential to enhance spatial-location accuracy.},
keywords = {},
pubstate = {published},
tppubtype = {conference}
}
Football video content analysis is a rapidly evolving field aiming to enrich the viewing experience of football matches. Current research often focuses on specific tasks like player and/or ball detection, tracking, and localisation in top-down views. Our study strives to integrate these efforts into a comprehensive Multi-Object Tracking (MOT) model capable of handling perspective transformations. Our framework, FootyVision, employs a YOLOv7 backbone trained on an extended player and ball dataset. The MOT module builds a gallery and assigns identities via the Hungarian algorithm based on feature embeddings, bounding box intersection over union, distance, and velocity. A novel component of our model is the perspective transformation module that leverages activation maps from the YOLOv7 backbone to compute homographies using lines, intersection points, and ellipses. This method effectively adapts to dynamic and uncalibrated video data, even in viewpoints with limited visual information. In terms of performance, FootyVision sets new benchmarks. The model achieves a mean average precision (mAP) of 95.7% and an F1-score of 95.5% in object detection. For MOT, it demonstrates robust capabilities, with an IDF1 score of approximately 93% on both ISSIA and SoccerNet datasets. For SoccerNet, it reaches a MOTA of 94.04% and shows competitive results for ISSIA. Additionally, FootyVision scores a HOTA(0) of 93.1% and an overall HOTA of 72.16% for the SoccerNet dataset. Our ablation study confirms the effectiveness of the selected tracking features and identifies key attributes for further improvement. While the model excels in maintaining track accuracy throughout the testing dataset, we recognise the potential to enhance spatial-location accuracy.
Andrews, Peter; Nordberg, Oda Elise; Guribye, Frode; Fujita, Kazuyuki; Fjeld, Morten; Borch, Njål
AiCommentator: A Multimodal Conversational Agent for Embedded Visualization in Football Viewing Conference
Intelligent User Interfaces (IUI), 2024.
@conference{AIComment,
title = {AiCommentator: A Multimodal Conversational Agent for Embedded Visualization in Football Viewing},
author = {Peter Andrews and Oda Elise Nordberg and Frode Guribye and Kazuyuki Fujita and Morten Fjeld and Njål Borch},
url = {https://mediafutures.no/acm_iui_24_aicommentator_peterandrews-1/},
year = {2024},
date = {2024-03-18},
urldate = {2024-03-18},
booktitle = {Intelligent User Interfaces (IUI)},
journal = {Intelligent User Interfaces (IUI)},
abstract = {Traditionally, sports commentators provide viewers with diverse information, encompassing in-game developments and player performances. Yet young adult football viewers increasingly use mobile devices for deeper insights during football matches. Such insights into players on the pitch and performance statistics support viewers’ understanding of game stakes, creating a more engaging viewing experience. Inspired by commentators’ traditional roles and to incorporate information into a single platform, we developed AiCommentator, a Multimodal Conversational Agent (MCA) for embedded visualization and conversational interactions in football broadcast video. AiCommentator integrates embedded visualization, either with an automated non-interactive or with a responsive interactive commentary mode. Our system builds upon multimodal techniques, integrating computer vision and large language models, to demonstrate ways for designing tailored, interactive sports-viewing content. AiCommentator’s event system infers game states based on a multi-object tracking algorithm and computer vision backend, facilitating automated responsive commentary. We address three key topics: evaluating young adults’ satisfaction and immersion across the two viewing modes, enhancing viewer understanding of in-game events and players on the pitch, and devising methods to present this information in a usable manner. In a mixed-method evaluation (n=16) of AiCommentator, we found that the participants appreciated aspects of both system modes but preferred the interactive mode, expressing a higher degree of engagement and satisfaction. Our paper reports on our development of AiCommentator and presents the results from our user study, demonstrating the promise of interactive MCA for a more engaging sports viewing experience. Systems like AiCommentator could be pivotal in transforming the interactivity and accessibility of sports content, revolutionizing how sports viewers engage with video content.},
keywords = {},
pubstate = {published},
tppubtype = {conference}
}
Traditionally, sports commentators provide viewers with diverse information, encompassing in-game developments and player performances. Yet young adult football viewers increasingly use mobile devices for deeper insights during football matches. Such insights into players on the pitch and performance statistics support viewers’ understanding of game stakes, creating a more engaging viewing experience. Inspired by commentators’ traditional roles and to incorporate information into a single platform, we developed AiCommentator, a Multimodal Conversational Agent (MCA) for embedded visualization and conversational interactions in football broadcast video. AiCommentator integrates embedded visualization, either with an automated non-interactive or with a responsive interactive commentary mode. Our system builds upon multimodal techniques, integrating computer vision and large language models, to demonstrate ways for designing tailored, interactive sports-viewing content. AiCommentator’s event system infers game states based on a multi-object tracking algorithm and computer vision backend, facilitating automated responsive commentary. We address three key topics: evaluating young adults’ satisfaction and immersion across the two viewing modes, enhancing viewer understanding of in-game events and players on the pitch, and devising methods to present this information in a usable manner. In a mixed-method evaluation (n=16) of AiCommentator, we found that the participants appreciated aspects of both system modes but preferred the interactive mode, expressing a higher degree of engagement and satisfaction. Our paper reports on our development of AiCommentator and presents the results from our user study, demonstrating the promise of interactive MCA for a more engaging sports viewing experience. Systems like AiCommentator could be pivotal in transforming the interactivity and accessibility of sports content, revolutionizing how sports viewers engage with video content.