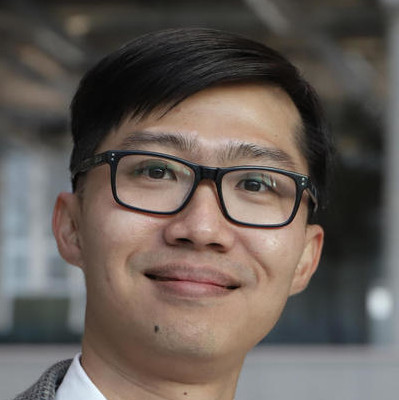
Duc-Tien Dang-Nguyen
Task Leader
2024
Khan, Sohail Ahmed; Dierickx, Laurence; Furuly, Jan-Gunnar; Vold, Henrik Brattli; Tahseen, Rano; Linden, Carl-Gustav; Dang-Nguyen, Duc-Tien
Debunking War Information Disorder: A Case Study in Assessing the Use of Multimedia Verification Tools Journal Article
In: Journal of the Association for Information Science and Technology, 2024.
@article{warinfo24,
title = {Debunking War Information Disorder: A Case Study in Assessing the Use of Multimedia Verification Tools},
author = {Sohail Ahmed Khan and Laurence Dierickx and Jan-Gunnar Furuly and Henrik Brattli Vold and Rano Tahseen and Carl-Gustav Linden and Duc-Tien Dang-Nguyen},
url = {https://mediafutures.no/jasist_verifyingwarconflict-compressed/},
year = {2024},
date = {2024-11-11},
journal = {Journal of the Association for Information Science and Technology},
abstract = {This paper investigates the use of multimedia verification, in particular, computational tools and Open-source Intelligence (OSINT) methods, for verifying online multimedia content in the context of the ongoing wars in Ukraine and Gaza. Our study examines the workflows and tools used by several fact-checkers and journalists working at Faktisk, a Norwegian fact-checking organisation. Our study showcases the effectiveness of diverse resources, including AI tools, geolocation tools, internet archives, and social media monitoring platforms, in enabling journalists and fact-checkers to efficiently process and corroborate evidence, ensuring the dissemination of accurate information. This research provides an in-depth analysis of the role of computational tools and OSINT methods for multimedia verification. It also underscores the potentials of currently available technology, and highlights its limitations while providing guidance for future development of digital multimedia verification tools and frameworks.},
keywords = {},
pubstate = {published},
tppubtype = {article}
}
Dang-Nguyen, Duc-Tien; Khan, Sohail Ahmed; Riegler, Michael; Halvorsen, Pål; Tran, Anh-Duy; Dao, Minh-Son; Tran, Minh-Triet
Overview of the Grand Challenge on Detecting Cheapfakes Proceedings Article
In: Proceedings of the 2024 International Conference on Multimedia Retrieval (ICMR’24), 2024.
@inproceedings{deteccheapfakes,
title = {Overview of the Grand Challenge on Detecting Cheapfakes},
author = {Duc-Tien Dang-Nguyen and Sohail Ahmed Khan and Michael Riegler and Pål Halvorsen and Anh-Duy Tran and Minh-Son Dao and Minh-Triet Tran},
url = {https://mediafutures.no/grand_challenge_on_detecting_cheapfakes-2/},
year = {2024},
date = {2024-06-10},
urldate = {2024-06-10},
booktitle = {Proceedings of the 2024 International Conference on Multimedia Retrieval (ICMR’24)},
abstract = {Cheapfake is a recently coined term that encompasses non-AI ("cheap") manipulations of multimedia content. Cheapfakes are known to be more prevalent than deepfakes. Cheapfake media can be created using editing software for image/video manipulations, or even without using any software, by simply altering the context of an image/video by sharing the media alongside misleading claims. This alteration of context is referred to as out-of-context (OOC) misuse of media. OOC media is much harder to detect than fake media, since the images and videos are not tampered. In this challenge, we focus on detecting OOC images, and more specifically the misuse of real photographs with conflicting image captions in news items. The aim of this challenge is to develop and benchmark models that can be used to detect whether given samples (news image and associated captions) are OOC, based on the recently compiled COSMOS dataset.},
keywords = {},
pubstate = {published},
tppubtype = {inproceedings}
}
Khan, Sohail Ahmed; Dang-Nguyen, Duc-Tien
CLIPping the Deception: Adapting Vision-Language Models for Universal Deepfake Detection Conference
ACM International Conference on Multimedia Retrieval (ICMR), 2024.
@conference{CLIpingSohail24,
title = {CLIPping the Deception: Adapting Vision-Language Models for Universal Deepfake Detection},
author = {Sohail Ahmed Khan and Duc-Tien Dang-Nguyen},
url = {https://mediafutures.no/icmr24/},
year = {2024},
date = {2024-04-07},
booktitle = {ACM International Conference on Multimedia Retrieval (ICMR)},
journal = {ACM International Conference on Multimedia Retrieval (ICMR)},
abstract = {The recent advancements in Generative Adversarial Networks (GANs) and the emergence of Diffusion models have significantly streamlined the production of highly realistic and widely accessible synthetic content. As a result, there is a pressing need for effective general purpose detection mechanisms to mitigate the potential risks posed by deepfakes. In this paper, we explore the effectiveness of pre-trained vision-language models (VLMs) when paired with recent adaptation methods for universal deepfake detection. Following previous studies in this domain, we employ only a single dataset (ProGAN) in order to adapt CLIP for deepfake detection. However, in contrast to prior research, which rely solely on the visual part of CLIP while ignoring its textual component, our analysis reveals that retaining the text part is crucial. Consequently, the simple and lightweight Prompt Tuning based adaptation strategy that we employ outperforms the previous SOTA approach by 5.01% mAP and 6.61% accuracy while utilizing less than one third of the training data (200k images as compared to 720k). To assess the real-world applicability of our proposed models, we conduct a comprehensive evaluation across various scenarios. This involves rigorous testing on images sourced from 21 distinct datasets, including those generated by GANs-based, Diffusion-based and Commercial tools.},
keywords = {},
pubstate = {published},
tppubtype = {conference}
}
2023
Khan, Sohail Ahmed; Dang-Nguyen, Duc-Tien
IEEE Access, 2023.
@conference{deepfakede,
title = {Deepfake Detection: Analysing Model Generalisation Across Architectures, Datasets and Pre-Training Paradigms},
author = {Sohail Ahmed Khan and Duc-Tien Dang-Nguyen},
url = {https://mediafutures.no/ieee_access_2023___deepfake_comparative_analysis/},
year = {2023},
date = {2023-12-15},
booktitle = {IEEE Access},
abstract = {As deepfake technology gains traction, the need for reliable detection systems is crucial. Recent research has introduced various deep learning-based detection systems, yet they exhibit limitations in generalizing effectively across diverse data distributions that differ from the training data. Our study focuses on understanding the generalization challenges by exploring specific aspects such as deep learning model architecture, pre-training strategy and datasets. Through a comprehensive comparative analysis, we evaluate multiple supervised and self-supervised deep learning models for deepfake detection.
Specifically, we evaluate eight supervised deep learning architectures and two transformer-based models pre-trained using self-supervised strategies (DINO, CLIP) on four different deepfake detection benchmarks (FakeAVCeleb, CelebDF-V2, DFDC and FaceForensics++). Our analysis includes intra-dataset and inter-dataset evaluations, examining the best performing models, generalisation capabilities and impact of augmentations. We also investigate the trade-off between model size, efficiency and performance. Our main goal is to provide insights into the effectiveness of different deep learning architectures (transformers, CNNs), training strategies (supervised, self-supervised) and deepfake detection benchmarks. Through our extensive analysis, we established that Transformer models outperform CNN models in deepfake detection. Also, we show that FaceForensics++ and DFDC datasets equip models with comparably better generalisation capabilities, as compared to FakeAVCeleb and CelebDF-V2 datasets. Our analysis also show that image augmentations can be helpful in achieving better performance, at least for the Transformer models.},
keywords = {},
pubstate = {published},
tppubtype = {conference}
}
Specifically, we evaluate eight supervised deep learning architectures and two transformer-based models pre-trained using self-supervised strategies (DINO, CLIP) on four different deepfake detection benchmarks (FakeAVCeleb, CelebDF-V2, DFDC and FaceForensics++). Our analysis includes intra-dataset and inter-dataset evaluations, examining the best performing models, generalisation capabilities and impact of augmentations. We also investigate the trade-off between model size, efficiency and performance. Our main goal is to provide insights into the effectiveness of different deep learning architectures (transformers, CNNs), training strategies (supervised, self-supervised) and deepfake detection benchmarks. Through our extensive analysis, we established that Transformer models outperform CNN models in deepfake detection. Also, we show that FaceForensics++ and DFDC datasets equip models with comparably better generalisation capabilities, as compared to FakeAVCeleb and CelebDF-V2 datasets. Our analysis also show that image augmentations can be helpful in achieving better performance, at least for the Transformer models.
Opdahl, Andreas L.; Tessem, Bjørnar; Dang-Nguyen, Duc-Tien; Motta, Enrico; Setty, Vinay; Throndsen, Eivind; Tverberg, Are; Trattner, Christoph
Trustworthy Journalism Through AI Journal Article
In: Data & Knowledge Engineering (DKE), Elsevier, 2023.
@article{Opdahl2023,
title = {Trustworthy Journalism Through AI},
author = {Andreas L. Opdahl and Bjørnar Tessem and Duc-Tien Dang-Nguyen and Enrico Motta and Vinay Setty and Eivind Throndsen and Are Tverberg and Christoph Trattner},
url = {https://mediafutures.no/1-s2-0-s0169023x23000423-main/},
year = {2023},
date = {2023-04-29},
urldate = {2023-04-29},
journal = {Data & Knowledge Engineering (DKE), Elsevier},
abstract = {Quality journalism has become more important than ever due to the need for quality and trustworthy media outlets that can provide accurate information to the public and help to address and counterbalance the wide and rapid spread of disinformation. At the same time, quality journalism is under pressure due to loss of revenue and competition from alternative information providers. This vision paper discusses how recent advances in Artificial Intelligence (AI), and in Machine Learning (ML) in particular, can be harnessed to support efficient production of high-quality journalism. From a news consumer perspective, the key parameter here concerns the degree of trust that is engendered by quality news production. For this reason, the paper will discuss how AI techniques can be applied to all aspects of news, at all stages of its production cycle, to increase trust.},
keywords = {},
pubstate = {published},
tppubtype = {article}
}
Khan, Sohail Ahmed; Sheikhi, Ghazaal; Opdahl, Andreas L.; Rabbi, Fazle; Stoppel, Sergej; Trattner, Christoph; Dang-Nguyen, Duc-Tien
Visual User-Generated Content Verification in Journalism: An Overview Journal Article
In: IEEE Access, 2023.
@article{KHAN2023,
title = {Visual User-Generated Content Verification in Journalism: An Overview},
author = {Sohail Ahmed Khan and Ghazaal Sheikhi and Andreas L. Opdahl and Fazle Rabbi and Sergej Stoppel and Christoph Trattner and Duc-Tien Dang-Nguyen},
url = {https://mediafutures.no/e0ret1-visual_user-generated_content_verification_in_journalism_an_overview/},
year = {2023},
date = {2023-01-16},
urldate = {2023-01-16},
journal = {IEEE Access},
abstract = {Over the past few years, social media has become an indispensable part of the news generation and dissemination cycle on the global stage. These digital channels along with the easy-to-use editing tools have unfortunately created a medium for spreading mis-/disinformation containing visual content. Media practitioners and fact-checkers continue to struggle with scrutinising and debunking visual user-generated content (UGC) quickly and thoroughly as verification of visual content requires a high level of expertise and could be exceedingly complex amid the existing computational tools employed in newsrooms. The aim of this study is to present a forward-looking perspective on how visual UGC verification in journalism can be transformed by multimedia forensics research. We elaborate on a comprehensive overview of the five elements of the UGC verification and propose multimedia forensics as the sixth element. In addition, different types of visual content forgeries and detection approaches proposed by the computer science research community are explained. Finally, a mapping of the available verification tools media practitioners rely on is created along with their limitations and future research directions to gain the confidence of media professionals in using multimedia forensics tools in their day-to-day routine.},
keywords = {},
pubstate = {published},
tppubtype = {article}
}
2022
Opdahl, Andreas L.; Al-Moslmi, Tareq; Dang-Nguyen, Duc-Tien; Ocaña, Marc Gallofré
Semantic Knowledge Graphs for the News: A Review Journal Article
In: ACM Computing Surveys, vol. 55, iss. 7, pp. 1-38, 2022.
@article{Opdahl2022,
title = {Semantic Knowledge Graphs for the News: A Review},
author = {Andreas L. Opdahl and Tareq Al-Moslmi and Duc-Tien Dang-Nguyen and Marc Gallofré Ocaña},
url = {https://mediafutures.no/3543508/},
year = {2022},
date = {2022-12-15},
urldate = {2022-12-15},
journal = {ACM Computing Surveys},
volume = {55},
issue = {7},
pages = {1-38},
abstract = {ICT platforms for news production, distribution, and consumption must exploit the ever-growing availability of digital data. These data originate from different sources and in different formats; they arrive at different velocities and in different volumes. Semantic knowledge graphs (KGs) is an established technique for integrating such heterogeneous information. It is therefore well-aligned with the needs of news producers and distributors, and it is likely to become increasingly important for the news industry. This article reviews the research on using semantic knowledge graphs for production, distribution, and consumption of news. The purpose is to present an overview of the field; to investigate what it means; and to suggest opportunities and needs for further research and development.},
keywords = {},
pubstate = {published},
tppubtype = {article}
}
Khan, Sohail Ahmed; Dang-Nguyen, Duc-Tien
Hybrid Transformer Network for Deepfake Detection Conference
Hybrid Transformer Network for Deepfake Detection, 2022.
@conference{Khan2022,
title = {Hybrid Transformer Network for Deepfake Detection},
author = {Sohail Ahmed Khan and Duc-Tien Dang-Nguyen},
url = {https://mediafutures.no/2208-05820-compressed-2/},
year = {2022},
date = {2022-08-11},
booktitle = {Hybrid Transformer Network for Deepfake Detection},
keywords = {},
pubstate = {published},
tppubtype = {conference}
}
2021
Tverberg, Are; Agasøster, Ingrid; Grønbæck, Mads; Monsen, Marius; Strand, Robert; Eikeland, Kristian; Throndsen, Eivind; Westvang, Lars; Knudsen, Tove B.; Fiskerud, Eivind; Skår, Rune; Stoppel, Sergej; Berven, Arne; Pedersen, Glenn Skare; Macklin, Paul; Cuomo, Kenneth; Vredenberg, Loek; Tolonen, Kristian; Opdahl, Andreas L.; Tessem, Bjørnar; Veres, Csaba; Dang-Nguyen, Duc-Tien; Motta, Enrico; Setty, Vinay Jayarama
WP3 2021 M3.1 Report The industrial expectations to, needs from and wishes for the work package Technical Report
University of Bergen, MediaFutures 2021.
@techreport{Tverberg2021,
title = {WP3 2021 M3.1 Report The industrial expectations to, needs from and wishes for the work package},
author = {Are Tverberg and Ingrid Agasøster and Mads Grønbæck and Marius Monsen and Robert Strand and Kristian Eikeland and Eivind Throndsen and Lars Westvang and Tove B. Knudsen and Eivind Fiskerud and Rune Skår and Sergej Stoppel and Arne Berven and Glenn Skare Pedersen and Paul Macklin and Kenneth Cuomo and Loek Vredenberg and Kristian Tolonen and Andreas L. Opdahl and Bjørnar Tessem and Csaba Veres and Duc-Tien Dang-Nguyen and Enrico Motta and Vinay Jayarama Setty},
url = {https://mediafutures.no/wp3-q2-2021-m3-1-report-by-the-industrial-partners-final-2/},
year = {2021},
date = {2021-07-25},
urldate = {2021-07-25},
institution = {University of Bergen, MediaFutures},
keywords = {},
pubstate = {published},
tppubtype = {techreport}
}
2020
Gurrin, Cathal; Joho, Hideo; Hopfgartner, Frank; Zhou, Liting; Albatal, Rami; Healy, Graham; Dang-Nguyen, Duc-Tien
Experiments in Lifelog Organisation and Retrieval at NTCIR Book Chapter
In: Evaluating Information Retrieval and Access Tasks, Chapter 13, pp. 187-203, Springer, Singapore, 2020, (Pre SFI).
@inbook{Gurrin2020,
title = {Experiments in Lifelog Organisation and Retrieval at NTCIR},
author = {Cathal Gurrin and Hideo Joho and Frank Hopfgartner and Liting Zhou and Rami Albatal and Graham Healy and Duc-Tien Dang-Nguyen},
url = {https://www.researchgate.net/publication/344047066_Experiments_in_Lifelog_Organisation_and_Retrieval_at_NTCIR},
doi = {10.1007/978-981-15-5554-1_13},
year = {2020},
date = {2020-12-01},
urldate = {2020-12-01},
booktitle = {Evaluating Information Retrieval and Access Tasks},
pages = {187-203},
publisher = {Springer},
address = {Singapore},
chapter = {13},
abstract = {Lifelogging can be described as the process by which individuals use various software and hardware devices to gather large archives of multimodal personal data from multiple sources and store them in a personal data archive, called a lifelog. The Lifelog task at NTCIR was a comparative benchmarking exercise with the aim of encouraging research into the organisation and retrieval of data from multimodal lifelogs. The Lifelog task ran for over 4 years from NTCIR-12 until NTCIR-14 (2015.02–2019.06); it supported participants to submit to five subtasks, each tackling a different challenge related to lifelog retrieval. In this chapter, a motivation is given for the Lifelog task and a review of progress since NTCIR-12 is presented. Finally, the lessons learned and challenges within the domain of lifelog retrieval are presented.},
note = {Pre SFI},
keywords = {},
pubstate = {published},
tppubtype = {inbook}
}
Boato, G.; Dang-Nguyen, Duc-Tien; Natale, F. G. B. De
Morphological filter detector for image forensics applications Journal Article
In: IEEE Access, vol. 8, pp. 13549-13560, 2020, (Pre SFI).
@article{Boato2020,
title = {Morphological filter detector for image forensics applications},
author = {G. Boato and Duc-Tien Dang-Nguyen and F.G.B. De Natale},
url = {https://www.researchgate.net/publication/338524511_Morphological_Filter_Detector_for_Image_Forensics_Applications},
doi = {10.1109/ACCESS.2020.2965745},
year = {2020},
date = {2020-01-01},
urldate = {2020-01-01},
journal = {IEEE Access},
volume = {8},
pages = {13549-13560},
abstract = {Mathematical morphology provides a large set of powerful non-linear image operators, widely used for feature extraction, noise removal or image enhancement. Although morphological filters might be used to remove artifacts produced by image manipulations, both on binary and graylevel documents, little effort has been spent towards their forensic identification. In this paper we propose a non-trivial extension of a deterministic approach originally detecting erosion and dilation of binary images. The proposed approach operates on grayscale images and is robust to image compression and other typical attacks. When the image is attacked the method looses its deterministic nature and uses a properly trained SVM classifier, using the original detector as a feature extractor. Extensive tests demonstrate that the proposed method guarantees very high accuracy in filtering detection, providing 100% accuracy in discriminating the presence and the type of morphological filter in raw images of three different datasets. The achieved accuracy is also good after JPEG compression, equal or above 76.8% on all datasets for quality factors above 80. The proposed approach is also able to determine the adopted structuring element for moderate compression factors. Finally, it is robust against noise addition and it can distinguish morphological filter from other filters.},
note = {Pre SFI},
keywords = {},
pubstate = {published},
tppubtype = {article}
}
2018
Dang-Nguyen, Duc-Tien; Riegler, Michael Alexander; Zhou, Liting; Gurrin, Cathal
Challenges and opportunities within personal life archives Conference
Proceedings of the 2018 ACM on International Conference on Multimedia Retrieval, 2018, (Pre SFI).
@conference{Nguyen2018,
title = {Challenges and opportunities within personal life archives},
author = {Duc-Tien Dang-Nguyen and Michael Alexander Riegler and Liting Zhou and Cathal Gurrin},
url = {https://www.researchgate.net/publication/325706506_Challenges_and_Opportunities_within_Personal_Life_Archives},
doi = {10.1145/3206025.3206040},
year = {2018},
date = {2018-06-01},
urldate = {2018-06-01},
booktitle = {Proceedings of the 2018 ACM on International Conference on Multimedia Retrieval},
pages = {335-343},
abstract = {Nowadays, almost everyone holds some form or other of a personal life archive. Automatically maintaining such an archive is an activity that is becoming increasingly common, however without automatic support the users will quickly be overwhelmed by the volume of data and will miss out on the potential benefits that lifelogs provide. In this paper we give an overview of the current status of lifelog research and propose a concept for exploring these archives. We motivate the need for new methodologies for indexing data, organizing content and supporting information access. Finally we will describe challenges to be addressed and give an overview of initial steps that have to be taken, to address the challenges of organising and searching personal life archives.},
note = {Pre SFI},
keywords = {},
pubstate = {published},
tppubtype = {conference}
}
2017
Boididou, Christina; Middleton, Stuart; Jin, Zhiwei; Papadopoulos, Symeon; Dang-Nguyen, Duc-Tien; Boato, G.; Kompatsiaris, Ioannis (Yiannis)
Verifying information with multimedia content on twitter: A comparative study of automated approaches Journal Article
In: Multimedia Tools and Applications, vol. 77, no. 12, pp. 15545-15571, 2017, (Pre SFI).
@article{Boididou2017,
title = {Verifying information with multimedia content on twitter: A comparative study of automated approaches},
author = {Christina Boididou and Stuart Middleton and Zhiwei Jin and Symeon Papadopoulos and Duc-Tien Dang-Nguyen and G. Boato and Ioannis (Yiannis) Kompatsiaris},
url = {https://www.researchgate.net/publication/319859894_Verifying_information_with_multimedia_content_on_twitter_A_comparative_study_of_automated_approaches},
doi = {10.1007/s11042-017-5132-9},
year = {2017},
date = {2017-09-01},
urldate = {2017-09-01},
journal = {Multimedia Tools and Applications},
volume = {77},
number = {12},
pages = {15545-15571},
abstract = {An increasing amount of posts on social media are used for dissem- inating news information and are accompanied by multimedia content. Such content may often be misleading or be digitally manipulated. More often than not, such pieces of content reach the front pages of major news outlets, having a detrimental eect on their credibility. To avoid such eects, there is profound need for automated methods that can help debunk and verify online content in very short time. To this end, we present a comparative study of three such methods that are catered for Twitter, a major social media platform used for news sharing. Those include: a) a method that uses textual patterns to extract
},
note = {Pre SFI},
keywords = {},
pubstate = {published},
tppubtype = {article}
}
Dang-Nguyen, Duc-Tien; Piras, Luca; Giacinto, Giorgio; Boato, G.; Natale, Francesco G. B. DE
Multimodal Retrieval with Diversification and Relevance Feedback for Tourist Attraction Images Journal Article
In: vol. 14, no. 4, pp. 1-24, 2017, (Pre SFI).
@article{Nguyen2017,
title = {Multimodal Retrieval with Diversification and Relevance Feedback for Tourist Attraction Images},
author = {Duc-Tien Dang-Nguyen and Luca Piras and Giorgio Giacinto and G. Boato and Francesco G. B. DE Natale
},
url = {https://www.researchgate.net/publication/319114515_Multimodal_Retrieval_with_Diversification_and_Relevance_Feedback_for_Tourist_Attraction_Images},
doi = {10.1145/3103613},
year = {2017},
date = {2017-08-01},
urldate = {2017-08-01},
booktitle = {ACM Transactions on Multimedia Computing, Communications, and Applications (TOMM)},
volume = {14},
number = {4},
pages = {1-24},
abstract = {In this article, we present a novel framework that can produce a visual description of a tourist attraction by choosing the most diverse pictures from community-contributed datasets, which describe different details of the queried location. The main strength of the proposed approach is its flexibility that permits us to filter out non-relevant images and to obtain a reliable set of diverse and relevant images by first clustering similar images according to their textual descriptions and their visual content and then extracting images from different clusters according to a measure of the user’s credibility. Clustering is based on a two-step process, where textual descriptions are used first and the clusters are then refined according to the visual features. The degree of diversification can be further increased by exploiting users’ judgments on the results produced by the proposed algorithm through a novel approach, where users not only provide a relevance feedback but also a diversity feedback. Experimental results performed on the MediaEval 2015 “Retrieving Diverse Social Images” dataset show that the proposed framework can achieve very good performance both in the case of automatic retrieval of diverse images and in the case of the exploitation of the users’ feedback. The effectiveness of the proposed approach has been also confirmed by a small case study involving a number of real users.},
note = {Pre SFI},
keywords = {},
pubstate = {published},
tppubtype = {article}
}