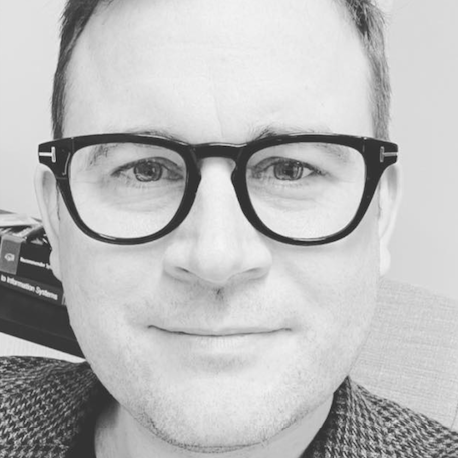
Details
Centre Director
WP6: Centre Management
University of Bergen
Contact
/ Biography
/ Publications
Publications from 2020 and before are not direct results of the SFI MediaFutures, but are key results from our team members working on related topics in MediaFutures.
2024
Rosnes, Daniel; Starke, Alain; Trattner, Christoph
ACM UMAP '24, 2024.
@conference{umap2024Daniel,
title = {Shaping the Future of Content-based News Recommenders: Insights from Evaluating Feature-Specific Similarity Metrics},
author = {Daniel Rosnes and Alain Starke and Christoph Trattner },
url = {https://mediafutures.no/umap2024/},
year = {2024},
date = {2024-07-01},
booktitle = {ACM UMAP '24},
abstract = {In news media, recommender system technology faces several domain-specific challenges. The continuous stream of new content and users deems content-based recommendation strategies, based on similar-item retrieval, to remain popular. However, a persistent challenge is to select relevant features and corresponding similarity functions, and whether this depends on the specific context. We evaluated feature-specific similarity metrics using human similarity judgments across national and local news domains. We performed an online experiment ($N = 141$) where we asked participants to judge the similarity between pairs of randomly sampled news articles. We had three contributions: (1) comparing novel metrics based on large language models to ones traditionally used in news recommendations, (2) exploring differences in similarity judgments across national and local news domains, and (3) examining which content-based strategies were perceived as appropriate in the news domain. Our results showed that one of the novel large language model based metrics (SBERT) was highly correlated with human judgments, while there were only small, most non-significant differences across national and local news domains. Finally, we found that while it may be possible to automatically recommend similar news using feature-specific metrics, their representativeness and appropriateness varied. We explain how our findings can guide the design of future content-based and hybrid recommender strategies in the news domain.},
keywords = {},
pubstate = {published},
tppubtype = {conference}
}
Diakopoulos, Nicholas; Trattner, Christoph; Jannach, Dietmar; Meijer, Irene Costera; Motta, Enrico
Leveraging Professional Ethics for Responsible AI: Applying AI techniques to journalism. Journal Article
In: Communications of the ACM, 2024.
@article{leverag24,
title = {Leveraging Professional Ethics for Responsible AI: Applying AI techniques to journalism.},
author = {Nicholas Diakopoulos and Christoph Trattner and Dietmar Jannach and Irene Costera Meijer and Enrico Motta},
url = {https://mediafutures.no/3625252/https://mediafutures.no/3625252-1/},
year = {2024},
date = {2024-01-23},
urldate = {2024-01-23},
journal = {Communications of the ACM},
keywords = {},
pubstate = {published},
tppubtype = {article}
}
2023
Seddik, Khadiga; Knudsen, Erik; Trilling, Damian; Trattner, Christoph
Understanding How News Recommender Systems Influence Selective Exposure Conference
Association for Computing Machinery (ACM) RecSys ’23, 2023.
@conference{behavrec2023,
title = {Understanding How News Recommender Systems Influence Selective Exposure},
author = {Khadiga Seddik and Erik Knudsen and Damian Trilling and Christoph Trattner },
url = {https://mediafutures.no/behavrec2023/},
year = {2023},
date = {2023-09-18},
urldate = {2023-09-18},
booktitle = {Association for Computing Machinery (ACM) RecSys ’23},
keywords = {},
pubstate = {published},
tppubtype = {conference}
}
Klimashevskaia, Anastasiia; Elahi, Mehdi; Jannach, Dietmar; Skjærven, Lars; Tessem, Astrid; Trattner, Christoph
Evaluating The Effects of Calibrated Popularity Bias Mitigation: A Field Study Conference
Association for Computing Machinery (ACM) RecSys ’23, 2023.
@conference{RecSys_2023_LBR,
title = {Evaluating The Effects of Calibrated Popularity Bias Mitigation: A Field Study},
author = {Anastasiia Klimashevskaia and Mehdi Elahi and Dietmar Jannach and Lars Skjærven and Astrid Tessem and Christoph Trattner},
url = {https://mediafutures.no/recsys_2023_lbr/},
year = {2023},
date = {2023-09-18},
booktitle = {Association for Computing Machinery (ACM) RecSys ’23},
keywords = {},
pubstate = {published},
tppubtype = {conference}
}
Knudsen, Erik; Starke, Alain D.; Trattner, Christoph
Association for Computing Machinery (ACM) RecSys ’23, 2023.
@conference{inra2023-1,
title = {Topical Preference Trumps Other Features in News Recommendation: A Conjoint Analysis on a Representative Sample from Norway},
author = {Erik Knudsen and Alain D. Starke and Christoph Trattner },
url = {https://mediafutures.no/inra2023-1/},
year = {2023},
date = {2023-09-18},
urldate = {2023-09-18},
booktitle = {Association for Computing Machinery (ACM) RecSys ’23},
keywords = {},
pubstate = {published},
tppubtype = {conference}
}
Majjodi, Ayoub El; Starke, Alain D.; Trattner, Christoph
Association for Computing Machinery (ACM) RecSys ’23, 2023.
@conference{inra2023,
title = {The Interplay between Food Knowledge, Nudges, and Preference Elicitation Methods Determines the Evaluation of a Recipe Recommender System},
author = {Ayoub El Majjodi and Alain D. Starke and Christoph Trattner },
url = {https://mediafutures.no/intrs2023-2/},
year = {2023},
date = {2023-09-18},
urldate = {2023-09-18},
booktitle = {Association for Computing Machinery (ACM) RecSys ’23},
keywords = {},
pubstate = {published},
tppubtype = {conference}
}
Opdahl, Andreas L.; Tessem, Bjørnar; Dang-Nguyen, Duc-Tien; Motta, Enrico; Setty, Vinay; Throndsen, Eivind; Tverberg, Are; Trattner, Christoph
Trustworthy Journalism Through AI Journal Article
In: Data & Knowledge Engineering (DKE), Elsevier, 2023.
@article{Opdahl2023,
title = {Trustworthy Journalism Through AI},
author = {Andreas L. Opdahl and Bjørnar Tessem and Duc-Tien Dang-Nguyen and Enrico Motta and Vinay Setty and Eivind Throndsen and Are Tverberg and Christoph Trattner},
url = {https://mediafutures.no/1-s2-0-s0169023x23000423-main/},
year = {2023},
date = {2023-04-29},
urldate = {2023-04-29},
journal = {Data & Knowledge Engineering (DKE), Elsevier},
abstract = {Quality journalism has become more important than ever due to the need for quality and trustworthy media outlets that can provide accurate information to the public and help to address and counterbalance the wide and rapid spread of disinformation. At the same time, quality journalism is under pressure due to loss of revenue and competition from alternative information providers. This vision paper discusses how recent advances in Artificial Intelligence (AI), and in Machine Learning (ML) in particular, can be harnessed to support efficient production of high-quality journalism. From a news consumer perspective, the key parameter here concerns the degree of trust that is engendered by quality news production. For this reason, the paper will discuss how AI techniques can be applied to all aspects of news, at all stages of its production cycle, to increase trust.},
keywords = {},
pubstate = {published},
tppubtype = {article}
}
Jeng, Jia Hua; Starke, Alain D.; Trattner, Christoph
Towards Attitudinal Change in News Recommender Systems: A Pilot Study on Climate Change Workshop
2023.
@workshop{Jeng2023,
title = {Towards Attitudinal Change in News Recommender Systems: A Pilot Study on Climate Change},
author = {Jia Hua Jeng and Alain D. Starke and Christoph Trattner},
url = {https://mediafutures.no/jeng2023-towards-attitudinal-change-in-news2908-2/},
year = {2023},
date = {2023-04-18},
urldate = {2023-04-18},
abstract = {Personalized recommender systems facilitate decision-making in various domains by presenting content closely aligned with users’ preferences.
However, personalization can lead to unintended consequences. In news, selective information exposure and consumption might amplify
polarization, as users are empowered to seek out information that is in line with their own attitudes and viewpoints. However, personalization in
terms of algorithmic content and persuasive technology could also help to narrow the gap between polarized user attitudes and news consumption
patterns. This paper presents a pilot study on climate change news. We examined the relation between users’ level of environmental concern, their preferences
for news articles, and news article content. We aimed to capture a news article’s viewpoint through sentiment analysis. Users (N = 180)
were asked to read and evaluate 10 news articles from the Washington Post. We found a positive correlation between users’ level of environmental
concern and whether they liked the article. In contrast, no significant correlation was found between sentiment and environmental concern.
We argue why a different type of news article analysis than sentiment is needed. Finally, we present our research agenda on how persuasive technology
might help to support more exploration of news article viewpoints in the future.},
keywords = {},
pubstate = {published},
tppubtype = {workshop}
}
However, personalization can lead to unintended consequences. In news, selective information exposure and consumption might amplify
polarization, as users are empowered to seek out information that is in line with their own attitudes and viewpoints. However, personalization in
terms of algorithmic content and persuasive technology could also help to narrow the gap between polarized user attitudes and news consumption
patterns. This paper presents a pilot study on climate change news. We examined the relation between users’ level of environmental concern, their preferences
for news articles, and news article content. We aimed to capture a news article’s viewpoint through sentiment analysis. Users (N = 180)
were asked to read and evaluate 10 news articles from the Washington Post. We found a positive correlation between users’ level of environmental
concern and whether they liked the article. In contrast, no significant correlation was found between sentiment and environmental concern.
We argue why a different type of news article analysis than sentiment is needed. Finally, we present our research agenda on how persuasive technology
might help to support more exploration of news article viewpoints in the future.
Angelsen, Aslaug; Starke, Alain D.; Trattner, Christoph
Healthiness and environmental impact of dinner recipes vary widely across developed countries Journal Article
In: Nature Food , 2023.
@article{Angelsen2023,
title = {Healthiness and environmental impact of dinner recipes vary widely across developed countries},
author = {Aslaug Angelsen and Alain D. Starke and Christoph Trattner},
url = {https://www.nature.com/articles/s43016-023-00746-5.epdf?sharing_token=40Ya430P4rvls-a-rD6AztRgN0jAjWel9jnR3ZoTv0MCv_q_H5Hiyr5PONI9wVjI-lufzQ9M-10R8so3hLNxXEl4h9v7mDvGnnsrg3iMb9lzo7cBlb7piPEpD26SYhEvanGp-MZrysYNovUsb5w_lSzfXiMvc6LraPBI2yf-nJQ%3D},
year = {2023},
date = {2023-04-06},
urldate = {2023-04-06},
journal = {Nature Food },
abstract = {Contrary to food ingredients, little is known about recipes’ healthiness or environmental impact. Here we examine 600 dinner recipes from Norway, the UK and the USA retrieved from cookbooks and the Internet. Recipe healthiness was assessed by adherence to dietary guidelines and aggregate health indicators based on front-of-pack nutrient labels, while environmental impact was assessed through greenhouse gas emissions and land use. Our results reveal that recipe healthiness strongly depends on the healthiness indicator used, with more than 70% of the recipes being classified as healthy for at least one front-of-pack label, but less than 1% comply with all dietary guidelines. All healthiness indicators correlated positively with each other and negatively with environmental impact. Recipes from the USA, found to use more red meat, have a higher environmental impact than those from Norway and the UK.},
keywords = {},
pubstate = {published},
tppubtype = {article}
}
Khan, Sohail Ahmed; Sheikhi, Ghazaal; Opdahl, Andreas L.; Rabbi, Fazle; Stoppel, Sergej; Trattner, Christoph; Dang-Nguyen, Duc-Tien
Visual User-Generated Content Verification in Journalism: An Overview Journal Article
In: IEEE Access, 2023.
@article{KHAN2023,
title = {Visual User-Generated Content Verification in Journalism: An Overview},
author = {Sohail Ahmed Khan and Ghazaal Sheikhi and Andreas L. Opdahl and Fazle Rabbi and Sergej Stoppel and Christoph Trattner and Duc-Tien Dang-Nguyen},
url = {https://mediafutures.no/e0ret1-visual_user-generated_content_verification_in_journalism_an_overview/},
year = {2023},
date = {2023-01-16},
urldate = {2023-01-16},
journal = {IEEE Access},
abstract = {Over the past few years, social media has become an indispensable part of the news generation and dissemination cycle on the global stage. These digital channels along with the easy-to-use editing tools have unfortunately created a medium for spreading mis-/disinformation containing visual content. Media practitioners and fact-checkers continue to struggle with scrutinising and debunking visual user-generated content (UGC) quickly and thoroughly as verification of visual content requires a high level of expertise and could be exceedingly complex amid the existing computational tools employed in newsrooms. The aim of this study is to present a forward-looking perspective on how visual UGC verification in journalism can be transformed by multimedia forensics research. We elaborate on a comprehensive overview of the five elements of the UGC verification and propose multimedia forensics as the sixth element. In addition, different types of visual content forgeries and detection approaches proposed by the computer science research community are explained. Finally, a mapping of the available verification tools media practitioners rely on is created along with their limitations and future research directions to gain the confidence of media professionals in using multimedia forensics tools in their day-to-day routine.},
keywords = {},
pubstate = {published},
tppubtype = {article}
}
2022
Hauptmann, Hanna; Said, Alan; Trattner, Christoph
Research directions in recommender systems for health and well-being Journal Article
In: User Modeling and User-Adapted Interaction Journal , 2022.
@article{Hauptmann2022,
title = {Research directions in recommender systems for health and well-being},
author = {Hanna Hauptmann and Alan Said and Christoph Trattner },
url = {https://mediafutures.no/s11257-022-09349-4/},
year = {2022},
date = {2022-11-14},
urldate = {2022-11-14},
journal = {User Modeling and User-Adapted Interaction Journal },
abstract = {Recommender systems have been put to use in the entertainment and e-commerce domains for decades, and in these decades, recommender systems have grown and matured into reliable and ubiquitous systems in today’s digital landscape. Relying on this maturity, the application of recommender systems for health and well-being has seen a rise in recent years, paving the way for tailored and personalized systems that support caretakers, caregivers, and other users in the health domain. In this introduction, we give a brief overview of the stakes, the requirements, and the possibilities that recommender systems for health and well-being bring.},
keywords = {},
pubstate = {published},
tppubtype = {article}
}
Majjodi, Ayoub El; Starke, Alain D.; Trattner, Christoph
Nudging Towards Health? Examining the Merits of Nutrition Labels and Personalization in a Recipe Recommender System, 2022.
@conference{Majjodi2022,
title = {Nudging Towards Health? Examining the Merits of Nutrition Labels and Personalization in a Recipe Recommender System},
author = { Ayoub El Majjodi and Alain D. Starke and Christoph Trattner
},
url = {https://dl.acm.org/doi/10.1145/3503252.3531312?fbclid=IwAR0eb6MPuISpVs9Vfkd-ww_KN7EjbMbiGdDQnPxjayogfKbHFgkSgeLdaxs},
year = {2022},
date = {2022-07-03},
urldate = {2022-07-03},
booktitle = {Nudging Towards Health? Examining the Merits of Nutrition Labels and Personalization in a Recipe Recommender System},
abstract = {Food recommender systems show personalized recipes to users based on content liked previously. Despite their potential, often recommended (popular) recipes in previous studies have turned out to be unhealthy, negatively contributing to prevalent obesity problems worldwide. Changing how foods are presented through digital nudges might help, but these are usually examined in non-personalized contexts, such as a brick-and-mortar supermarket. This study seeks to support healthy food choices in a personalized interface by adding front-of-package nutrition labels to recipes in a food recommender system. After performing an offline evaluation, we conducted an online study (N = 600) with six different recommender interfaces, based on a 2 (non-personalized vs. personalized recipe advice) x 3 (No Label, Multiple Traffic Light, Nutri-Score) between-subjects design. We found that recipe choices made in the non-personalized scenario were healthier, while the use of nutrition labels (our digital nudge) reduced choice difficulty when the content was personalized.},
keywords = {},
pubstate = {published},
tppubtype = {conference}
}
Bogina, Veronica; Kuflik, Tsvi; Jannach, Dietmar; Bielikova, Maria; Kompan, Michal; Trattner, Christoph
Considering Temporal Aspects in Recommender Systems: A Survey Journal Article
In: UMUAI journal, 2022.
@article{Bogina2022,
title = {Considering Temporal Aspects in Recommender Systems: A Survey },
author = {Veronica Bogina and Tsvi Kuflik and Dietmar Jannach and Maria Bielikova and Michal Kompan and Christoph Trattner
},
url = {https://mediafutures.no/revisedversion_considering_temporal_aspects_in_rs_a_survey-6/},
year = {2022},
date = {2022-05-31},
urldate = {2022-05-31},
journal = {UMUAI journal},
abstract = {The widespread use of temporal aspects in user modeling indicates their importance, and their consideration showed to be highly effective in var- ious domains related to user modeling, especially in recommender systems. Still, past and ongoing research, spread over several decades, provided multi- ple ad-hoc solutions, but no common understanding of the issue. There is no standardization and there is often little commonality in considering tempo- ral aspects in different applications. This may ultimately lead to the problem that application developers define ad-hoc solutions for their problems at hand, sometimes missing or neglecting aspects that proved to be effective in similar cases. Therefore, a comprehensive survey of the consideration of temporal as- pects in recommender systems is required. In this work, we provide an overview of various time-related aspects, categorize existing research, present a tempo- ral abstraction and point to gaps that require future research. We anticipate this survey will become a reference point for researchers and practitioners alike when considering the potential application of temporal aspects in their personalized applications.},
keywords = {},
pubstate = {published},
tppubtype = {article}
}
Elahi, Mehdi; Starke, Alain D.; Ioini, Nabil El; Lambrix, Anna Alexander; Trattner, Christoph
Developing and Evaluating a University Recommender System Journal Article
In: Frontiers in Artificial Intelligence , 2022.
@article{Elahi2022,
title = {Developing and Evaluating a University Recommender System},
author = {Mehdi Elahi and Alain D. Starke and Nabil El Ioini and Anna Alexander Lambrix and Christoph Trattner},
url = {https://www.frontiersin.org/articles/10.3389/frai.2021.796268/full},
doi = {https://doi.org/10.3389/frai.2021.796268},
year = {2022},
date = {2022-02-02},
journal = {Frontiers in Artificial Intelligence },
abstract = {A challenge for many young adults is to find the right institution to follow higher education. Global university rankings are a commonly used, but inefficient tool, for they do not consider a person's preferences and needs. For example, some persons pursue prestige in their higher education, while others prefer proximity. This paper develops and evaluates a university recommender system, eliciting user preferences as ratings to build predictive models and to generate personalized university ranking lists. In Study 1, we performed offline evaluation on a rating dataset to determine which recommender approaches had the highest predictive value. In Study 2, we selected three algorithms to produce different university recommendation lists in our online tool, asking our users to compare and evaluate them in terms of different metrics (Accuracy, Diversity, Perceived Personalization, Satisfaction, and Novelty). We show that a SVD algorithm scores high on accuracy and perceived personalization, while a KNN algorithm scores better on novelty. We also report findings on preferred university features.},
keywords = {},
pubstate = {published},
tppubtype = {article}
}
Kvifte, Tord; Elahi, Mehdi; Trattner, Christoph
Hybrid Recommendation of Movies based on Deep Content Features Proceedings Article
In: Springer Nature, 2022.
@inproceedings{cristin1957037,
title = {Hybrid Recommendation of Movies based on Deep Content Features},
author = {Tord Kvifte and Mehdi Elahi and Christoph Trattner},
url = {https://app.cristin.no/results/show.jsf?id=1957037, Cristin
https://aip-research-center.github.io/AIPA_workshop/2021/},
year = {2022},
date = {2022-01-01},
booktitle = {Springer Nature},
abstract = {When a movie is uploaded to a movie Recommender System (e.g., YouTube), the system can exploit various forms of descriptive features (e.g., tags and genre) in order to generate personalized recommendation for users. However, there are situations where the descriptive features are missing or very limited and the system may fail to include such a movie in the recommendation list. This paper investigates hybrid recommendation based on a novel form of content features, extracted from movies, in order to generate recommendation for users. Such features represent the visual aspects of movies, based on Deep Learning models, and hence, do not require any human annotation when extracted. We have evaluated our proposed technique using a large dataset of movies and shown that automatically extracted visual features can mitigate the cold-start problem by generating recommendation with a superior quality compared to different baselines, including recommendation based on human-annotated features.},
keywords = {},
pubstate = {published},
tppubtype = {inproceedings}
}
2021
Trattner, Christoph; Jannach, Dietmar; Motta, Enrico; Meijer, Irene Costera; Diakopoulos, Nicholas; Elahi, Mehdi; Opdahl, Andreas L.; Tessem, Bjørnar; Borch, Njål; Fjeld, Morten; Øvrelid, Lilja; Smedt, Koenraad De; Moe, Hallvard
Responsible media technology and AI: challenges and research directions Journal Article
In: AI and Ethics, 2021.
@article{cristin2000622,
title = {Responsible media technology and AI: challenges and research directions},
author = {Christoph Trattner and Dietmar Jannach and Enrico Motta and Irene Costera Meijer and Nicholas Diakopoulos and Mehdi Elahi and Andreas L. Opdahl and Bjørnar Tessem and Njål Borch and Morten Fjeld and Lilja Øvrelid and Koenraad De Smedt and Hallvard Moe},
url = {https://app.cristin.no/results/show.jsf?id=2000622, Cristin
https://link.springer.com/content/pdf/10.1007/s43681-021-00126-4.pdf},
doi = {https://doi.org/10.1007/s43681-021-00126-4},
year = {2021},
date = {2021-12-20},
urldate = {2021-12-20},
journal = {AI and Ethics},
keywords = {},
pubstate = {published},
tppubtype = {article}
}
Elahi, Mehdi; Jannach, Dietmar; Skjærven, Lars; Knudsen, Erik; Sjøvaag, Helle; Tolonen, Kristian; Holmstad, Øyvind; Pipkin, Igor; Throndsen, Eivind; Stenbom, Agnes; Fiskerud, Eivind; Oesch, Adrian; Vredenberg, Loek; Trattner, Christoph
Towards Responsible Media Recommendation Journal Article
In: AI and Ethics, 2021.
@article{cristin1953352,
title = {Towards Responsible Media Recommendation},
author = {Mehdi Elahi and Dietmar Jannach and Lars Skjærven and Erik Knudsen and Helle Sjøvaag and Kristian Tolonen and Øyvind Holmstad and Igor Pipkin and Eivind Throndsen and Agnes Stenbom and Eivind Fiskerud and Adrian Oesch and Loek Vredenberg and Christoph Trattner},
url = {https://app.cristin.no/results/show.jsf?id=1953352, Cristin
https://link.springer.com/article/10.1007%2Fs43681-021-00107-7},
doi = {https://doi.org/10.1007/s43681-021-00107-7},
year = {2021},
date = {2021-11-02},
journal = {AI and Ethics},
keywords = {},
pubstate = {published},
tppubtype = {article}
}
Starke, Alain D.; Willemsen, Martijn C.; Trattner, Christoph
Nudging Healthy Choices in Food Search Through Visual Attractiveness Journal Article
In: no. April 2021, pp. 1-18, 2021.
@article{Starke2021,
title = {Nudging Healthy Choices in Food Search Through Visual Attractiveness},
author = {Alain D. Starke and Martijn C. Willemsen and Christoph Trattner},
url = {https://www.frontiersin.org/articles/10.3389/frai.2021.621743/full},
doi = {10.3389/frai.2021.621743},
year = {2021},
date = {2021-04-22},
number = {April 2021},
pages = {1-18},
abstract = {Recipe websites are becoming increasingly popular to support people in their home cooking. However, most of these websites prioritize popular recipes, which tend to be unhealthy. Drawing upon research on visual biases and nudges, this paper investigates whether healthy food choices can be supported in food search by depicting attractive images alongside recipes, as well as by re-ranking search results on health. After modelling the visual attractiveness of recipe images, we asked 239 users to search for specific online recipes and to select those they liked the most. Our analyses revealed that users tended to choose a healthier recipe if a visually attractive image was depicted alongside it, as well as if it was listed at the top of a list of search results. Even though less popular recipes were promoted this way, it did not come at the cost of a user’s level of satisfaction},
keywords = {},
pubstate = {published},
tppubtype = {article}
}
Jannach, Dietmar; Jesse, Mathias; Jugovac, Michael; Trattner, Christoph
Exploring Multi-List User Interfaces for Similar-Item Recommendations Conference
29th ACM International Conference on User Modeling, Adaptation and Personalization (UMAP '21) 2021.
@conference{Jannach2021,
title = {Exploring Multi-List User Interfaces for Similar-Item Recommendations},
author = {Dietmar Jannach and Mathias Jesse and Michael Jugovac and Christoph Trattner},
url = {https://mediafutures.no/conference_umap_2021-2/},
year = {2021},
date = {2021-03-26},
organization = {29th ACM International Conference on User Modeling, Adaptation and Personalization (UMAP '21)},
abstract = {On many e-commerce and media streaming sites, the user inter-face (UI) consists of multiple lists of item suggestions. The itemsin each list are usually chosen based on pre-defined strategies and,e.g., show movies of the same genre or category. Such interfacesare common in practice, but there is almost no academic researchregarding the optimal design and arrangement of such multi-listUIs for recommenders. In this paper, we report the results of anexploratory user study that examined the effects of various designalternatives on the decision-making behavior of users in the con-text of similar-item recommendations. Our investigations showed,among other aspects, that decision-making is slower and more de-manding with multi-list interfaces, but that users also explore moreoptions before making a decision. Regarding the selection of thealgorithm to retrieve similar items, our study furthermore revealsthe importance of considering social-based similarity measures.},
keywords = {},
pubstate = {published},
tppubtype = {conference}
}
Elahi, Mehdi; Moghaddam, Farshad Bakhshandegan; Hosseini, Reza; Rimaz, Mohammad Hossein; Ioini, Nabil El; Tkalcic, Marko; Trattner, Christoph; Tillo, Tammam
Recommending Videos in Cold Start With Automatic Visual Tags Proceedings Article
In: Association for Computing Machinery (ACM), 2021.
@inproceedings{cristin1956967,
title = {Recommending Videos in Cold Start With Automatic Visual Tags},
author = {Mehdi Elahi and Farshad Bakhshandegan Moghaddam and Reza Hosseini and Mohammad Hossein Rimaz and Nabil El Ioini and Marko Tkalcic and Christoph Trattner and Tammam Tillo},
url = {https://app.cristin.no/results/show.jsf?id=1956967, Cristin
https://dl.acm.org/doi/10.1145/3450614.3461687},
year = {2021},
date = {2021-01-01},
booktitle = {Association for Computing Machinery (ACM)},
abstract = {This paper addresses the so-called New Item problem in video Recommender Systems, as part of Cold Start. New item problem occurs when a new item is added to the system catalog, and the recommender system has no or little data describing that item. This could cause the system to fail to meaningfully recommend the new item to the users. We propose a novel technique that can generate cold start recommendation by utilizing automatic visual tags, i.e., tags that are automatically annotated by deeply analyzing the content of the videos and detecting faces, objects, and even celebrities within the videos. The automatic visual tags do not need any human involvement and have been shown to be very effective in representing the video content. In order to evaluate our proposed technique, we have performed a set of experiments using a large dataset of videos. The results have shown that the automatically extracted visual tags can be incorporated into the cold start recommendation process and achieve superior results compared to the recommendation based on human-annotated tags.},
keywords = {},
pubstate = {published},
tppubtype = {inproceedings}
}
Elahi, Mehdi; Moghaddam, Farshad Bakhshandegan; Hosseini, Reza; Rimaz, Mohammad Hossein; Trattner, Christoph
Enhanced Movie Recommendation Incorporating Visual Features Working paper
2021.
@workingpaper{cristin1957034,
title = {Enhanced Movie Recommendation Incorporating Visual Features},
author = {Mehdi Elahi and Farshad Bakhshandegan Moghaddam and Reza Hosseini and Mohammad Hossein Rimaz and Christoph Trattner},
url = {https://app.cristin.no/results/show.jsf?id=1957034, Cristin},
year = {2021},
date = {2021-01-01},
keywords = {},
pubstate = {published},
tppubtype = {workingpaper}
}
Starke, Alain D.; Asotic, Edis; Trattner, Christoph
“Serving Each User”: Supporting Different Eating Goals Through a Multi-List Recommender Interface Proceedings Article
In: Association for Computing Machinery (ACM), 2021.
@inproceedings{cristin1956504,
title = {“Serving Each User”: Supporting Different Eating Goals Through a Multi-List Recommender Interface},
author = {Alain D. Starke and Edis Asotic and Christoph Trattner},
url = {https://app.cristin.no/results/show.jsf?id=1956504, Cristin},
doi = {https://doi.org/10.1145/3460231.3474232},
year = {2021},
date = {2021-01-01},
urldate = {2021-01-01},
booktitle = {Association for Computing Machinery (ACM)},
keywords = {},
pubstate = {published},
tppubtype = {inproceedings}
}
Starke, Alain D.; Trattner, Christoph
Promoting Healthy Food Choices Online: A Case for Multi-List Recommender Systems Proceedings Article
In: Association for Computing Machinery (ACM), 2021.
@inproceedings{cristin1956555,
title = {Promoting Healthy Food Choices Online: A Case for Multi-List Recommender Systems},
author = {Alain D. Starke and Christoph Trattner},
url = {https://app.cristin.no/results/show.jsf?id=1956555, Cristin},
year = {2021},
date = {2021-01-01},
urldate = {2021-01-01},
booktitle = {Association for Computing Machinery (ACM)},
keywords = {},
pubstate = {published},
tppubtype = {inproceedings}
}
Musto, Cataldo; Starke, Alain D.; Trattner, Christoph; Rapp, Amon; Semeraro, Giovanni
Exploring the effects of natural language justifications on food recommender systems Proceedings Article
In: Association for Computing Machinery (ACM), 2021.
@inproceedings{cristin1956541,
title = {Exploring the effects of natural language justifications on food recommender systems},
author = {Cataldo Musto and Alain D. Starke and Christoph Trattner and Amon Rapp and Giovanni Semeraro},
url = {https://app.cristin.no/results/show.jsf?id=1956541, Cristin},
year = {2021},
date = {2021-01-01},
urldate = {2021-01-01},
booktitle = {Association for Computing Machinery (ACM)},
keywords = {},
pubstate = {published},
tppubtype = {inproceedings}
}
Starke, Alain D.; Trattner, Christoph; Bakken, Hedda; Johannessen, Martin Skivenesvåg; Solberg, Vegard
The Cholesterol Factor: Balancing Accuracy and Health in Recipe Recommendation Through a Nutrient-Specific Metric Proceedings Article
In: Association for Computing Machinery (ACM), 2021.
@inproceedings{cristin1956600,
title = {The Cholesterol Factor: Balancing Accuracy and Health in Recipe Recommendation Through a Nutrient-Specific Metric},
author = {Alain D. Starke and Christoph Trattner and Hedda Bakken and Martin Skivenesvåg Johannessen and Vegard Solberg},
url = {https://app.cristin.no/results/show.jsf?id=1956600, Cristin},
year = {2021},
date = {2021-01-01},
urldate = {2021-01-01},
booktitle = {Association for Computing Machinery (ACM)},
keywords = {},
pubstate = {published},
tppubtype = {inproceedings}
}
Berge, Arngeir; Sjøen, Vegard Velle; Starke, Alain D.; Trattner, Christoph
Changing Salty Food Preferences with Visual and Textual Explanations in a Search Interface Proceedings Article
In: Association for Computing Machinery (ACM), 2021.
@inproceedings{cristin1956563,
title = {Changing Salty Food Preferences with Visual and Textual Explanations in a Search Interface},
author = {Arngeir Berge and Vegard Velle Sjøen and Alain D. Starke and Christoph Trattner},
url = {https://app.cristin.no/results/show.jsf?id=1956563, Cristin},
year = {2021},
date = {2021-01-01},
urldate = {2021-01-01},
booktitle = {Association for Computing Machinery (ACM)},
keywords = {},
pubstate = {published},
tppubtype = {inproceedings}
}
Starke, Alain D.; Larsen, Sebastian Øverhaug; Trattner, Christoph
Predicting Feature-based Similarity in the News Domain Using Human Judgments Proceedings Article
In: Association for Computing Machinery (ACM), 2021.
@inproceedings{cristin1956594,
title = {Predicting Feature-based Similarity in the News Domain Using Human Judgments},
author = {Alain D. Starke and Sebastian Øverhaug Larsen and Christoph Trattner},
url = {https://app.cristin.no/results/show.jsf?id=1956594, Cristin},
year = {2021},
date = {2021-01-01},
urldate = {2021-01-01},
booktitle = {Association for Computing Machinery (ACM)},
keywords = {},
pubstate = {published},
tppubtype = {inproceedings}
}
Berge, Arngeir; Sjøen, Vegard Velle; Starke, Alain D.; Trattner, Christoph
Changing Salty Food Preferences with Visual and TextualExplanations in a Search Interface Journal Article
In: CEUR Workshop Proceedings, 2021.
@article{cristin1933059,
title = {Changing Salty Food Preferences with Visual and TextualExplanations in a Search Interface},
author = {Arngeir Berge and Vegard Velle Sjøen and Alain D. Starke and Christoph Trattner},
url = {https://app.cristin.no/results/show.jsf?id=1933059, Cristin
http://ceur-ws.org/Vol-2903/IUI21WS-HEALTHI-2.pdf},
year = {2021},
date = {2021-01-01},
urldate = {2021-01-01},
journal = {CEUR Workshop Proceedings},
abstract = {Salt is consumed at too high levels in the general population, causing high blood pressure and related health problems. In this paper, we present results of ongoing research that tries to reduce salt intake via technology and in particular from an interface perspective. In detail, this paper features results of a study that examines the extent to which visual and textual explanations in a search interface can change salty food preferences. An online user study with 200 participants demonstrates that this is possible in food search results by accompanying recipes with a visual taste map that includes salt-replacer herbs and spices in the calculation of salty taste.},
keywords = {},
pubstate = {published},
tppubtype = {article}
}
2019
Trattner, Christoph; Jannach, Dietmar
Learning to Recommend Similar Items from Human Judgements Journal Article
In: User Modeling and User-Adapted Interaction Journal, pp. 1-50, 2019, (Pre SFI).
@article{Trattner2020,
title = {Learning to Recommend Similar Items from Human Judgements},
author = {Christoph Trattner and Dietmar Jannach},
url = {https://www.christophtrattner.info/pubs/UMUAI2019.pdf},
doi = {10.1007/s11257-019-09245-4},
year = {2019},
date = {2019-09-20},
journal = {User Modeling and User-Adapted Interaction Journal},
pages = {1-50},
abstract = {Similar item recommendations—a common feature of many Web sites—point users to other interesting objects given a currently inspected item. A common way of computing such recommendations is to use a similarity function, which expresses how much alike two given objects are. Such similarity functions are usually designed based on the specifics of the given application domain. In this work, we explore how such functions can be learned from human judgments of similarities between objects, using two domains of “quality and taste”—cooking recipe and movie recommendation—as guiding scenarios. In our approach, we first collect a few thousand pairwise similarity assessments with the help of crowdworkers. Using these data, we then train different machine learning models that can be used as similarity functions to compare objects. Offline analyses reveal for both application domains that models that combine different types of item characteristics are the best predictors for human-perceived similarity. To further validate the usefulness of the learned models, we conducted additional user studies. In these studies, we exposed participants to similar item recommendations using a set of models that were trained with different feature subsets. The results showed that the combined models that exhibited the best offline prediction performance led to the highest user-perceived similarity, but also to recommendations that were considered useful by the participants, thus confirming the feasibility of our approach.},
note = {Pre SFI},
keywords = {},
pubstate = {published},
tppubtype = {article}
}
2018
Ocaña, Marc Gallofré; Nyre, Lars; Opdahl, Andreas L.; Tessem, Bjørnar; Trattner, Christoph; Veres, Csaba
Towards a big data platform for news angles Workshop
Norwegian Big Data Symposium 2018, 2018, (Pre SFI).
@workshop{Ocaña2018,
title = {Towards a big data platform for news angles},
author = {Marc Gallofré Ocaña and Lars Nyre and Andreas L. Opdahl and Bjørnar Tessem and Christoph Trattner and Csaba Veres},
url = {https://www.researchgate.net/publication/332274562_Towards_a_Big_Data_Platform_for_News_Angles},
year = {2018},
date = {2018-11-01},
urldate = {2018-11-01},
booktitle = {Norwegian Big Data Symposium 2018},
abstract = {Finding good angles on news events is a central journalistic and editorial skill. As news work becomes increasingly computer-assisted and big-data based, journalistic tools therefore need to become better able to support news angles too. This paper outlines a big-data platform that is able to suggest appropriate angles on news events to journalists. We first clarify and discuss the central characteristics of news angles. We then proceed to outline a big-data architecture that can propose news angles. Important areas for further work include: representing news angles formally; identifying interesting and unexpected angles on unfolding events; and designing a big-data architecture that works on a global scale.
},
note = {Pre SFI},
keywords = {},
pubstate = {published},
tppubtype = {workshop}
}
Sciascio, Cecilia Di; Brusilovsky, Peter; Trattner, Christoph; Veas, Eduardo
The Roadmap to User-Controllable Social Exploratory Search Journal Article
In: ACM Transactions on Interactive Intelligent Systems, pp. 1-37, 2018, (Pre SFI).
@article{Sciascio2018,
title = {The Roadmap to User-Controllable Social Exploratory Search},
author = {Cecilia Di Sciascio and Peter Brusilovsky and Christoph Trattner and Eduardo Veas},
url = {https://www.christophtrattner.info/pubs/TIIS2019.pdf},
year = {2018},
date = {2018-01-01},
journal = {ACM Transactions on Interactive Intelligent Systems},
pages = {1-37},
abstract = {Information-seeking tasks with learning or investigative purposes are usually referred to as exploratory
search. Exploratory search unfolds as a dynamic process where the user, amidst navigation, trial-and-error
and on-the-!y selections, gathers and organizes information (resources). A range of innovative interfaces
with increased user control have been developed to support exploratory search process. In this work we
present our attempt to increase the power of exploratory search interfaces by using ideas of social search,
i.e., leveraging information left by past users of information systems. Social search technologies are highly
popular nowadays, especially for improving ranking. However, current approaches to social ranking do not
allow users to decide to what extent social information should be taken into account for result ranking. This
paper presents an interface that integrates social search functionality into an exploratory search system in
a user-controlled way that is consistent with the nature of exploratory search. The interface incorporates
control features that allow the user to (i) express information needs by selecting keywords and (ii) to express
preferences for incorporating social wisdom based on tag matching and user similarity. The interface promotes
search transparency through color-coded stacked bars and rich tooltips. This work presents the full series
of evaluations conducted to, "rst, assess the value of the social models in contexts independent to the user
interface, in terms of objective and perceived accuracy. Then, in a study with the full-!edged system, we
investigated system accuracy and subjective aspects with a structural model that revealed that, when users
actively interacted with all its control features, the hybrid system outperformed a baseline content-based-only
tool and users were more satis"ed.},
note = {Pre SFI},
keywords = {},
pubstate = {published},
tppubtype = {article}
}
search. Exploratory search unfolds as a dynamic process where the user, amidst navigation, trial-and-error
and on-the-!y selections, gathers and organizes information (resources). A range of innovative interfaces
with increased user control have been developed to support exploratory search process. In this work we
present our attempt to increase the power of exploratory search interfaces by using ideas of social search,
i.e., leveraging information left by past users of information systems. Social search technologies are highly
popular nowadays, especially for improving ranking. However, current approaches to social ranking do not
allow users to decide to what extent social information should be taken into account for result ranking. This
paper presents an interface that integrates social search functionality into an exploratory search system in
a user-controlled way that is consistent with the nature of exploratory search. The interface incorporates
control features that allow the user to (i) express information needs by selecting keywords and (ii) to express
preferences for incorporating social wisdom based on tag matching and user similarity. The interface promotes
search transparency through color-coded stacked bars and rich tooltips. This work presents the full series
of evaluations conducted to, "rst, assess the value of the social models in contexts independent to the user
interface, in terms of objective and perceived accuracy. Then, in a study with the full-!edged system, we
investigated system accuracy and subjective aspects with a structural model that revealed that, when users
actively interacted with all its control features, the hybrid system outperformed a baseline content-based-only
tool and users were more satis"ed.
2017
Elsweiler, David; Trattner, Christoph; Harvey, Morgan
Exploiting Food Choice Biases for Healthier Recipe Recommendation Conference
ACM SIGIR Conference 2017, (Pre SFI).
@conference{Elsweiler2017,
title = {Exploiting Food Choice Biases for Healthier Recipe Recommendation},
author = {David Elsweiler and Christoph Trattner and Morgan Harvey},
url = {https://www.christophtrattner.info/pubs/SIGIR2017.pdf},
year = {2017},
date = {2017-08-01},
organization = {ACM SIGIR Conference},
abstract = {By incorporating healthiness into the food recommendation / ranking
process we have the potential to improve the eating habits of a
growing number of people who use the Internet as a source of food
inspiration. In this paper, using insights gained from various data
sources, we explore the feasibility of substituting meals that would
typically be recommended to users with similar, healthier dishes.
First, by analysing a recipe collection sourced from Allrecipes.com,
we quantify the potential for nding replacement recipes, which are
comparable but have dierent nutritional characteristics and are
nevertheless highly rated by users. Building on this, we present two
controlled user studies (n=107, n=111) investigating how people
perceive and select recipes. We show participants are unable to
reliably identify which recipe contains most fat due to their answers
being biased by lack of information, misleading cues and limited
nutritional knowledge on their part. By applying machine learning
techniques to predict the preferred recipes, good performance can
be achieved using low-level image features and recipe meta-data as
predictors. Despite not being able to consciously determine which
of two recipes contains most fat, on average, participants select
the recipe with the most fat as their preference. The importance of
image features reveals that recipe choices are often visually driven.
A nal user study (n=138) investigates to what extent the predictive
models can be used to select recipe replacements such that users
can be “nudged” towards choosing healthier recipes. Our ndings
have important implications for online food systems.},
note = {Pre SFI},
keywords = {},
pubstate = {published},
tppubtype = {conference}
}
process we have the potential to improve the eating habits of a
growing number of people who use the Internet as a source of food
inspiration. In this paper, using insights gained from various data
sources, we explore the feasibility of substituting meals that would
typically be recommended to users with similar, healthier dishes.
First, by analysing a recipe collection sourced from Allrecipes.com,
we quantify the potential for nding replacement recipes, which are
comparable but have dierent nutritional characteristics and are
nevertheless highly rated by users. Building on this, we present two
controlled user studies (n=107, n=111) investigating how people
perceive and select recipes. We show participants are unable to
reliably identify which recipe contains most fat due to their answers
being biased by lack of information, misleading cues and limited
nutritional knowledge on their part. By applying machine learning
techniques to predict the preferred recipes, good performance can
be achieved using low-level image features and recipe meta-data as
predictors. Despite not being able to consciously determine which
of two recipes contains most fat, on average, participants select
the recipe with the most fat as their preference. The importance of
image features reveals that recipe choices are often visually driven.
A nal user study (n=138) investigates to what extent the predictive
models can be used to select recipe replacements such that users
can be “nudged” towards choosing healthier recipes. Our ndings
have important implications for online food systems.
Elahi, Mehdi; Hosseini, Reza; Rimaz, Mohammad Hossein; Moghaddam, Farshad B.; Trattner, Christoph
Visually-Aware Video Recommendation in the Cold Start Conference
Proccedings of theACM Hypertext 2020 2017, (Pre SFI).
@conference{Elahi2017b,
title = {Visually-Aware Video Recommendation in the Cold Start},
author = {Mehdi Elahi and Reza Hosseini and Mohammad Hossein Rimaz and Farshad B. Moghaddam and Christoph Trattner},
url = {https://christophtrattner.com/pubs/ht2020.pdf},
year = {2017},
date = {2017-07-01},
urldate = {2017-07-01},
pages = {1-5},
organization = {Proccedings of theACM Hypertext 2020},
abstract = {Recommender Systems (RSs) have become essential tools in any
video-sharing platforms (such as YouTube) by generating video
suggestions for users. Although, RSs have been e!ective, however,
they su!er from the so-called New Item problem. New item problem,
as part of Cold Start problem, happens when a new item is added to
the system catalogue and the RS has no or little data available for
that new item. In such a case, the system may fail to meaningfully
recommend the new item to any user.
In this paper, we propose a novel recommendation technique
based on visual tags, i.e., tags that are automatically annotated
to videos based on visual description of videos. Such visual tags
can be used in an extreme cold start situation, where neither any
rating, nor any tag is available for the new video item. The visual
tags could also be used in the moderate cold start situation when
the new video item has been annotated with few tags. This type
of content features can be extracted automatically without any
human involvement and have been shown to be very e!ective in
representing the video content.
We have used a large dataset of videos and shown that automatically
extracted visual tags can be incorporated into the cold start
recommendation process and achieve superior results compared to
the recommendation based on human-annotated tags.},
note = {Pre SFI},
keywords = {},
pubstate = {published},
tppubtype = {conference}
}
video-sharing platforms (such as YouTube) by generating video
suggestions for users. Although, RSs have been e!ective, however,
they su!er from the so-called New Item problem. New item problem,
as part of Cold Start problem, happens when a new item is added to
the system catalogue and the RS has no or little data available for
that new item. In such a case, the system may fail to meaningfully
recommend the new item to any user.
In this paper, we propose a novel recommendation technique
based on visual tags, i.e., tags that are automatically annotated
to videos based on visual description of videos. Such visual tags
can be used in an extreme cold start situation, where neither any
rating, nor any tag is available for the new video item. The visual
tags could also be used in the moderate cold start situation when
the new video item has been annotated with few tags. This type
of content features can be extracted automatically without any
human involvement and have been shown to be very e!ective in
representing the video content.
We have used a large dataset of videos and shown that automatically
extracted visual tags can be incorporated into the cold start
recommendation process and achieve superior results compared to
the recommendation based on human-annotated tags.
2016
Mutlu, Belgin; Veas, Eduardo; Trattner, Christoph
VizRec: Recommending Personalized Visualizations Journal Article
In: ACM Transactions on Interactive Intelligent Systems (TiiS), vol. 6, no. 4, pp. 1-40, 2016, (Pre SFI).
@article{Mutlu2016,
title = {VizRec: Recommending Personalized Visualizations},
author = {Belgin Mutlu and Eduardo Veas and Christoph Trattner},
url = {https://www.christophtrattner.info/pubs/ACM-TIIS.pdf},
year = {2016},
date = {2016-01-01},
journal = {ACM Transactions on Interactive Intelligent Systems (TiiS)},
volume = {6},
number = {4},
pages = {1-40},
abstract = {Visualizations have a distinctive advantage when dealing with the information overload problem: since they
are grounded in basic visual cognition, many people understand them. However, creating the appropriate
representation requires specific expertise of the domain and underlying data. Our quest in this paper is to
study methods to suggest appropriate visualizations autonomously. To be appropriate, a visualization has
to follow studied guidelines to find and distinguish patterns visually, and encode data therein. Thus, a
visualization tells a story of the underlying data; yet, to be appropriate, it has to clearly represent those aspects
of the data the viewer is interested in. Which aspects of a visualization are important to the viewer? Can
we capture and use those aspects to recommend visualizations? This paper investigates strategies to
recommend visualizations considering different aspects of user preferences. A multi-dimensional scale is used to
estimate aspects of quality for charts for collaborative filtering. Alternatively, tag vectors describing charts
are used to recommend potentially interesting charts based on content. Finally, a hybrid approach combines
information on what a chart is about (tags) and how good it is (ratings). We present the design principles
behind VizRec, our visual recommender. We describe its architecture, the data acquisition approach with a
crowd sourced study, and the analysis of strategies for visualization recommendation.},
note = {Pre SFI},
keywords = {},
pubstate = {published},
tppubtype = {article}
}
are grounded in basic visual cognition, many people understand them. However, creating the appropriate
representation requires specific expertise of the domain and underlying data. Our quest in this paper is to
study methods to suggest appropriate visualizations autonomously. To be appropriate, a visualization has
to follow studied guidelines to find and distinguish patterns visually, and encode data therein. Thus, a
visualization tells a story of the underlying data; yet, to be appropriate, it has to clearly represent those aspects
of the data the viewer is interested in. Which aspects of a visualization are important to the viewer? Can
we capture and use those aspects to recommend visualizations? This paper investigates strategies to
recommend visualizations considering different aspects of user preferences. A multi-dimensional scale is used to
estimate aspects of quality for charts for collaborative filtering. Alternatively, tag vectors describing charts
are used to recommend potentially interesting charts based on content. Finally, a hybrid approach combines
information on what a chart is about (tags) and how good it is (ratings). We present the design principles
behind VizRec, our visual recommender. We describe its architecture, the data acquisition approach with a
crowd sourced study, and the analysis of strategies for visualization recommendation.