Four research papers from MediaFutures are set to be presented at the 32nd ACM International Conference on User Modeling, Adaptation, and Personalization (ACM UMAP) 2024, a premier event for groundbreaking advancements in user-adaptive systems. This conference covers a broad spectrum of topics, including adaptive websites, personalized learning, and recommender systems.
Amongst the researchers are MediaFutures professors, research assistants and PhDs, almost all of them part of the research group DARS which stands for Behavioral Data Analytics & Recommender Systems Research Group.
Center Director Christoph Trattner expresses his satisfaction in witnessing MediaFutures PhDs being at the forefront of the research:
It’s rewarding to see that our work is making a difference not just in the academic world, but also for our industry partners.
Perception versus Reality: Evaluating User Awareness of Political Selective Exposure in News Recommender Systems
– Alain D. Starke, Anders Sandvik Bremnes, Erik Knudsen, Damian Trilling, Christoph Trattner
This study looked into how aware people are of getting news that matches their political views from recommendation systems. These systems are commonly used to customize news for users based on what they like, but they often end up showing similar perspectives, which can limit diversity. MediaFutures Associate Professor Alain D. Starke, Associate Professor Erik Knudsen, Professor Damian Trilling, Professor Christoph Trattner and research assistant Anders Bremnes designed an online experiment in which users were asked to select any number of news articles that matched their political orientation (i.e., Democrat or Republican) from a list of 50 news articles (5 Democrat, 5 Republican, 40 filler articles). The researchers wanted to see how well people could tell if an article matched their political bias. Some articles were clearly labeled as leaning towards a political side, while others were filler articles. Surprisingly, they found no big difference in how people picked articles between those shown the articles in a random order, and those shown clearly on top of the page. However, they noticed that Republicans had more trouble than Democrats in finding articles that matched their views.
Emotional Reframing of Economic News using a Large Language
– Jeng Jia-Hua, Gloria Anne Babile Kasangu, Alain D. Starke, Christoph Trattner
MediaFutures Ph.D. candidate Jeng Jia-Hua collaborated with Associate Professor Alain Starke, Professor and center director Christoph Trattner and research assistant Gloria Anne Babile Kasangu on a study about emotional framing techniques and trust in the media. Together, they investigated how ChatGPT’s emotional framing of news impacts readers’ emotions and trust compared to framing by human journalists. Their focus included emotions such as anger, fear, hope, and neutrality (typical of journalists). The results revealed that news framed with anger and fear by ChatGPT triggered stronger negative emotions in readers compared to human-framed news. However, hopeful framing showed minimal overall changes. Surprisingly, trust in the news remained consistently stable across all framing conditions. The paper delves into the implications of these findings, particularly regarding how emotional framing might contribute to societal polarization issues.
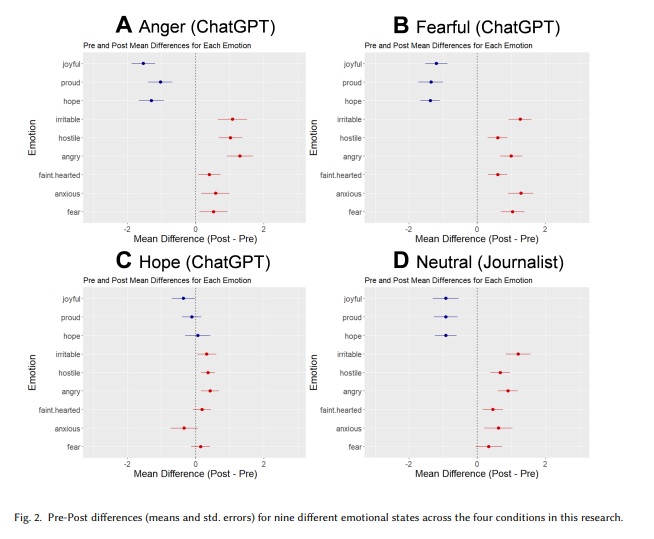
Incorporating Editorial Feedback in the Evaluation of News Recommender Systems
– Bilal Mahmood, Mehdi Elahi, Samia Touileb, Lubos Steskal, Christoph Trattner
The third accepted paper tackled the complex interplay between editorial control and personalization within recommender systems. MediaFutures Ph.D. candidate Bilal Mahmood conducted together with team of Associate Professors Mehdi Elahi and Samia Touileb, as well as Data Scientist at TV2, Lubos Steskal and Professor Christoph Trattner experiments to evaluate the relevance of recommendations from an editorial standpoint. Using data from TV 2 Norway, a major editor-managed media house, they compared algorithm-generated recommendations, utilizing the K-Nearest Neighbor (KNN) model and various text embedding techniques to analyze different parts of news articles (e.g., title, lead title, body text, and full text), against editorial feedback. The results highlight the recommendation system’s effectiveness in meeting editorial expectations. Mahmood discovered that TV2 could achieve approximately 30% alignment with editorial recommendations using their approach, a promising outcome with significant implications for the recommender system community.
Shaping the Future of Content-based News Recommenders: Insights from Evaluating Feature-Specific Similarity Metrics
– Daniel Rosnes, Alain D. Starke, Christoph Trattner
This paper is about improving how news articles are recommended to readers. The researchers faced challenges because news content and readers are always changing. They tested different ways to measure how similar news articles are to each other, using a group of people to judge this.
The study found three main things:
- A new way of measuring similarity (using the large language model based metrics SBERT) matched closely with what people thought.
- The differences between national and local news were small and mostly not important.
- While it’s possible to suggest similar news articles automatically, the quality and suitability of these suggestions can vary.
The paper provides insights that could help design better systems for suggesting news articles based on their content and other factors. This could make it easier for readers to find news that is relevant and interesting to them.
All papers can be found under “publications” in the menu.