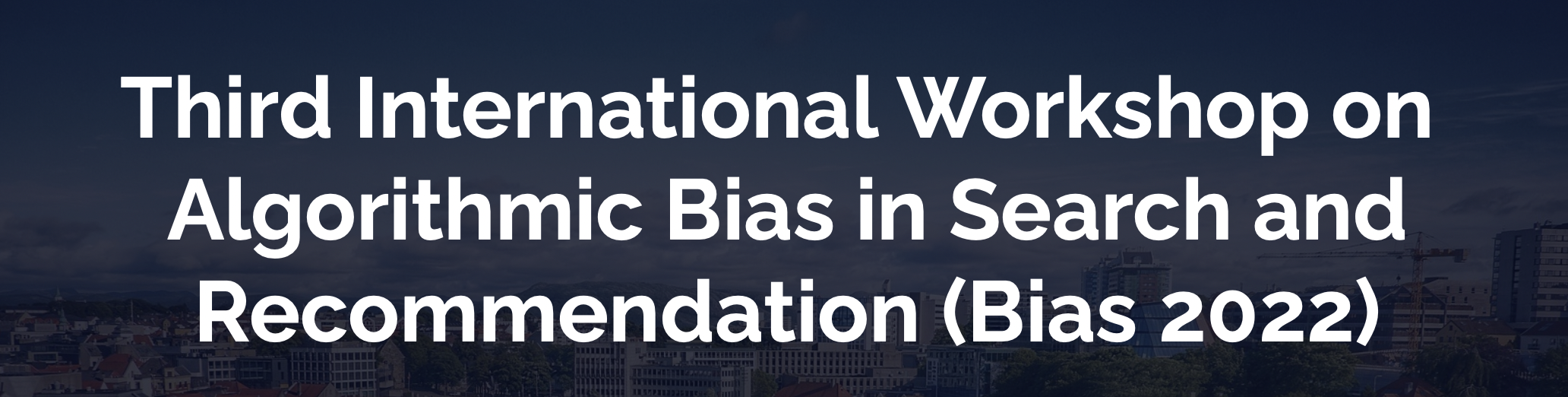
We are excited to inform that a research paper Mitigating Popularity Bias in Recommendation: Potential and Limits of Calibration Approaches by Anastasiia Klimashevskaia1, Mehdi Elahi, Dietmar Jannach, Christoph Trattner and Lars Skjæerven, has been accepted for oral presentation and inclusion in the proceedings of the Third International Workshop on Algorithmic Bias in Search and Recommendation (Bias 2022).
Held as part of the 44th European Conference on Information Retrieval, the workshop aims to collect novel contribution in the field of search and recommendation algorithms, and offers a common ground for interested researchers and partitioners. The workshop will take place in April in Stavanger, Norway.
In Mitigating Popularity Bias in Recommendation: Potential and Limits of Calibration Approaches, MediaFutures WP2 researchers used a dataset provided by their industry partner, Norwegian Broadcaster TV2. The paper constitutes an offline study that assesses different re-anking approaches to recommendation systems.
Abstract:
While recommender systems are highly successful at helping users find relevant information online, they may also exhibit a certain undesired bias of mostly promoting only already popular items. Various approaches of quantifying and mitigating such biases were put forward in the literature. Most recently, calibration methods were proposed that aim to match the popularity of the recommended items with popularity preferences of individual users. In this paper, we show that while such methods are efficient in avoiding the recommendation of too popular items for some users, other techniques may be more effective in reducing the popularity bias on the platform level. Overall, our work highlights that in practice choices regarding metrics and algorithms have to be made with caution to ensure the desired effects.