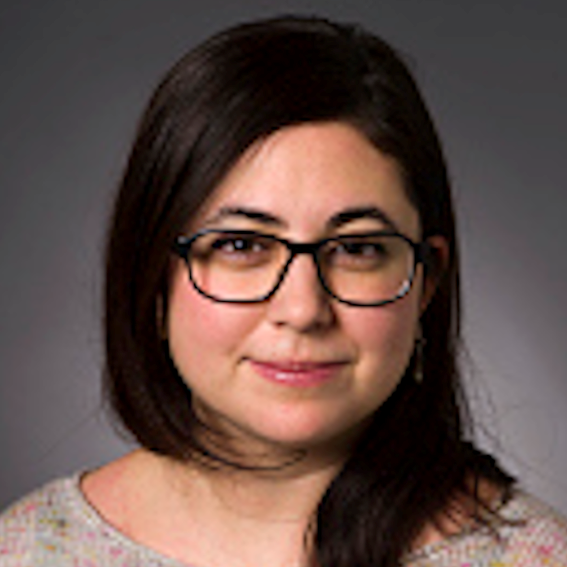
Samia Touileb
Researcher
2024
Mahmood, Bilal; Elahi, Mehdi; Touileb, Samia; Steskal, Lubos; Trattner, Christoph
Incorporating Editorial Feedback in the Evaluation of News Recommender Systems Conference
ACM UMAP 2024, 2024.
@conference{incoed24,
title = {Incorporating Editorial Feedback in the Evaluation of News Recommender Systems},
author = {Bilal Mahmood and Mehdi Elahi and Samia Touileb and Lubos Steskal and Christoph Trattner},
url = {https://mediafutures.no/lbr_umap_editorial_component_in_nrs/},
year = {2024},
date = {2024-07-01},
urldate = {2024-07-01},
booktitle = {ACM UMAP 2024},
abstract = {Research in the recommender systems field typically applies a rather traditional evaluation methodology when assessing the quality of recommendations. This methodology heavily relies on incorporating different forms of user feedback (e.g., clicks) representing the specific needs and interests of the users. While this methodology may offer various benefits, it may fail to comprehensively project the complexities of certain application domains, such as the news domain. This domain is distinct from other domains primarily due to the strong influence of editorial control in the news delivery process. Incorporation of this role can profoundly impact how the relevance of news articles is measured when recommended to the users. Despite its critical importance, there appears to be a research gap in investigating the dynamics between the roles of editorial control and personalization in the community of recommender systems. In this paper, we address this gap by conducting experiments where the relevance of recommendations is assessed from an editorial perspective. We received a real-world dataset from TV 2, one of the largest editor-managed commercial media houses in Norway, which includes editors’ feedback on how news articles are being related. In our experiment, we considered a scenario where algorithm-generated recommendations, using the K-Nearest Neighbor (KNN) model, employing various text embedding models to encode different sections of the news articles (e.g., title, lead title, body text, and full text), are compared against the editorial feedback. The results are promising, demonstrating the effectiveness of the recommendation in fulfilling the editorial prospects.},
keywords = {},
pubstate = {published},
tppubtype = {conference}
}
Touileb, Samia; Murstad, Jeanett; Mæhlum, Petter; Steskal, Lubos; Storset, Lilja Charlotte; You, Huiling; Øvrelid, Lilja
EDEN: A Dataset for Event Detection in Norwegian News Conference
LREC-COLING 2024, 2024.
@conference{EDEN,
title = {EDEN: A Dataset for Event Detection in Norwegian News},
author = {Samia Touileb and Jeanett Murstad and Petter Mæhlum and Lubos Steskal and Lilja Charlotte Storset and Huiling You and Lilja Øvrelid},
url = {https://mediafutures.no/2024-lrec-main-488/},
year = {2024},
date = {2024-05-23},
booktitle = {LREC-COLING 2024},
abstract = {We present EDEN, the first Norwegian dataset annotated with event information at the sentence level, adapting the
widely used ACE event schema to Norwegian. The paper describes the manual annotation of Norwegian text as well
as transcribed speech in the news domain, together with inter-annotator agreement and discussions of relevant
dataset statistics. We also present preliminary modeling results using a graph-based event parser. The resulting
dataset will be made freely available for download and use.
},
keywords = {},
pubstate = {published},
tppubtype = {conference}
}
widely used ACE event schema to Norwegian. The paper describes the manual annotation of Norwegian text as well
as transcribed speech in the news domain, together with inter-annotator agreement and discussions of relevant
dataset statistics. We also present preliminary modeling results using a graph-based event parser. The resulting
dataset will be made freely available for download and use.
2023
Samuel, David; Kutuzov, Andrey; Touileb, Samia; Velldal, Erik; Øvrelid, Lilja; Rønningstad, Egil; Sigdel, Elina; Palatkina, Anna
NorBench – A Benchmark for Norwegian Language Models Conference
2023.
@conference{Samuel2023,
title = {NorBench – A Benchmark for Norwegian Language Models},
author = {David Samuel and Andrey Kutuzov and Samia Touileb and Erik Velldal and Lilja Øvrelid and Egil Rønningstad and Elina Sigdel and Anna Palatkina},
url = {https://mediafutures.no/2023_nodalida-1_61/},
year = {2023},
date = {2023-05-24},
urldate = {2023-05-24},
abstract = {We present NorBench: a streamlined suite of NLP tasks and probes for evaluating Norwegian language models (LMs) on standardized data splits and evaluation metrics. We also introduce a range of new Norwegian language models (both encoder and encoder-decoder based). Finally, we compare and analyze their performance, along with other existing LMs, across the different benchmark tests of NorBench.},
keywords = {},
pubstate = {published},
tppubtype = {conference}
}
Sheikhi, Ghazaal; Touileb, Samia; Khan, Sohail Ahmed
2023.
@conference{Sheikhi2023,
title = {Automated Claim Detection for Fact-checking: A Case Study using Norwegian Pre-trained Language Models},
author = {Ghazaal Sheikhi and Samia Touileb and Sohail Ahmed Khan },
url = {https://mediafutures.no/2023_nodalida-1_1/},
year = {2023},
date = {2023-05-24},
urldate = {2023-05-24},
abstract = {We investigate to what extent pre-trained language models can be used for automated claim detection for fact-checking in a low resource setting. We explore this idea by fine-tuning four Norwegian pre-trained language models to perform the binary classification task of determining if a claim should be discarded or upheld to be further processed by human fact-checkers. We conduct a set of experiments to compare the performance of the language models, and provide a simple baseline model using SVM with tf-idf features. Since we are focusing on claim detection, the recall score for the upheld class is to be emphasized over other performance measures. Our experiments indicate that the language models are superior to the baseline system in terms of F1, while the baseline model results in the highest precision. However, the two Norwegian models, NorBERT2 and NB-BERT_large, give respectively superior F1 and recall values. We argue that large language models could be successfully employed to solve the automated claim detection problem. The choice of the model depends on the desired end-goal. Moreover, our error analysis shows that language models are generally less sensitive to the changes in claim length and source than the SVM model.},
keywords = {},
pubstate = {published},
tppubtype = {conference}
}
Touileb, Samia; Øvrelid, Lilja; Velldal, Erik
Measuring Normative and Descriptive Biases in Language Models Using Census Data Conference
2023.
@conference{Touileb2023,
title = {Measuring Normative and Descriptive Biases in Language Models Using Census Data},
author = {Samia Touileb and Lilja Øvrelid and Erik Velldal},
url = {https://mediafutures.no/2023_eacl-main_164/},
year = {2023},
date = {2023-05-02},
abstract = {We investigate in this paper how distributions of occupations with respect to gender is reflected
in pre-trained language models. Such distributions are not always aligned to normative ideals, nor do they necessarily reflect a descriptive assessment of reality. In this paper, we introduce an approach for measuring to what degree pre-trained language models are aligned to normative and descriptive occupational distributions. To this end, we use official demographic information about gender–occupation distributions provided by the national statistics agencies of France, Norway, United Kingdom, and the United States. We manually generate template-based sentences combining gendered pronouns and nouns with occupations,
and subsequently probe a selection of ten language models covering the English, French, and Norwegian languages. The scoring system we introduce in this work is language independent, and can be used on any combination of
template-based sentences, occupations, and languages. The approach could also be extended to other dimensions of national census data and other demographic variables.},
keywords = {},
pubstate = {published},
tppubtype = {conference}
}
in pre-trained language models. Such distributions are not always aligned to normative ideals, nor do they necessarily reflect a descriptive assessment of reality. In this paper, we introduce an approach for measuring to what degree pre-trained language models are aligned to normative and descriptive occupational distributions. To this end, we use official demographic information about gender–occupation distributions provided by the national statistics agencies of France, Norway, United Kingdom, and the United States. We manually generate template-based sentences combining gendered pronouns and nouns with occupations,
and subsequently probe a selection of ten language models covering the English, French, and Norwegian languages. The scoring system we introduce in this work is language independent, and can be used on any combination of
template-based sentences, occupations, and languages. The approach could also be extended to other dimensions of national census data and other demographic variables.
2022
Touileb, Samia; Nozza, Debora
Measuring Harmful Representations in Scandinavian Language Models Conference
2022.
@conference{Touileb2022b,
title = {Measuring Harmful Representations in Scandinavian Language Models},
author = {Samia Touileb and Debora Nozza},
url = {https://mediafutures.no/2211-11678/},
year = {2022},
date = {2022-11-21},
urldate = {2022-11-21},
abstract = {Scandinavian countries are perceived as rolemodels when it comes to gender equality. With the advent of pre-trained language models and their widespread usage, we investigate to what extent gender-based harmful and toxic content exist in selected Scandinavian language models. We examine nine models, covering Danish, Swedish, and Norwegian, by manually creating template-based sentences and probing
the models for completion. We evaluate the completions using two methods for measuring harmful and toxic completions and provide a thorough analysis of the results. We show that Scandinavian pre-trained language models contain harmful and gender-based stereotypes with similar values across all languages.
This finding goes against the general expectations related to gender equality in Scandinavian countries and shows the possible problematic outcomes of using such models in real world settings.},
keywords = {},
pubstate = {published},
tppubtype = {conference}
}
the models for completion. We evaluate the completions using two methods for measuring harmful and toxic completions and provide a thorough analysis of the results. We show that Scandinavian pre-trained language models contain harmful and gender-based stereotypes with similar values across all languages.
This finding goes against the general expectations related to gender equality in Scandinavian countries and shows the possible problematic outcomes of using such models in real world settings.
Mæhlum, Petter; Kåsen, Andre; Touileb, Samia; Barnes, Jeremy
Annotating Norwegian language varieties on Twitter for Part-of-speech Workshop
2022.
@workshop{Mæhlum2022,
title = {Annotating Norwegian language varieties on Twitter for Part-of-speech},
author = {Petter Mæhlum and Andre Kåsen and Samia Touileb and Jeremy Barnes},
url = {https://mediafutures.no/2022-vardial-1-7/},
year = {2022},
date = {2022-10-24},
urldate = {2022-10-24},
abstract = {Norwegian Twitter data poses an interesting challenge for Natural Language Processing (NLP) tasks. These texts are difficult for models trained on standardized text in one of the two Norwegian written forms (Bokmål and Nynorsk), as they contain both the typical variation of social media text, as well as a large amount of dialectal variety. In this paper we present a novel Norwegian Twitter dataset annotated with POS-tags. We show that models trained on Universal Dependency (UD) data perform worse when evaluated against this dataset, and that models trained on Bokmål generally perform better than those trained on Nynorsk. We also see that performance on dialectal tweets is comparable to the written standards for some models. Finally we perform a detailed analysis of the errors that models commonly make on this data.},
keywords = {},
pubstate = {published},
tppubtype = {workshop}
}
Touileb, Samia; Øvrelid, Lilja; Velldal, Erik
Occupational Biases in Norwegian and Multilingual Language Models Workshop
2022.
@workshop{Touileb2022,
title = {Occupational Biases in Norwegian and Multilingual Language Models},
author = {Samia Touileb and Lilja Øvrelid and Erik Velldal },
url = {https://mediafutures.no/2022-gebnlp-1-21/},
year = {2022},
date = {2022-07-01},
abstract = {In this paper we explore how a demographic distribution of occupations, along gender dimensions, is reflected in pre-trained language models. We give a descriptive assessment of the distribution of occupations, and investigate to what extent these are reflected in four Norwegian and two multilingual models. To this end, we introduce a set of simple bias probes, and perform five different tasks combining gendered pronouns, first names, and a set of occupations from the Norwegian statistics bureau. We show that language specific models obtain more accurate results, and are much closer to the real-world distribution of clearly gendered occupations. However, we see that none of the models have correct representations of the occupations that are demographically balanced between genders. We also discuss the importance of the training data on which the models were trained on, and argue that template-based bias probes can sometimes be fragile, and a simple alteration in a template can change a model’s behavior.},
keywords = {},
pubstate = {published},
tppubtype = {workshop}
}
Kutuzov, Andrei; Touileb, Samia; Mæhlum, Petter; Enstad, Tita; Witteman, Alexandra
NorDiaChange: Diachronic Semantic Change Dataset for Norwegian Book Chapter
In: pp. 2563-2572, European Language Resources Association NVI-nivå 1, 2022, ISBN: 979-10-95546-72-6.
@inbook{nokeyi,
title = {NorDiaChange: Diachronic Semantic Change Dataset for Norwegian},
author = {Andrei Kutuzov and Samia Touileb and Petter Mæhlum and Tita Enstad and Alexandra Witteman},
url = {https://www.duo.uio.no/handle/10852/100876},
isbn = {979-10-95546-72-6},
year = {2022},
date = {2022-06-25},
pages = {2563-2572},
publisher = {European Language Resources Association NVI-nivå 1},
abstract = {We describe NorDiaChange: the first diachronic semantic change dataset for Norwegian. NorDiaChange comprises two novel subsets, covering about 80 Norwegian nouns manually annotated with graded semantic change over time. Both datasets follow the same annotation procedure and can be used interchangeably as train and test splits for each other. NorDiaChange covers the time periods related to pre- and post-war events, oil and gas discovery in Norway, and technological developments. The annotation was done using the DURel framework and two large historical Norwegian corpora. NorDiaChange is published in full under a permissive licence, complete with raw annotation data and inferred diachronic word usage graphs (DWUGs).},
keywords = {},
pubstate = {published},
tppubtype = {inbook}
}
2021
Touileb, Samia; Øvrelid, Lilja; Velldal, Erik
Using Gender- and Polarity-informed Models to Investigate Bias Working paper
2021.
@workingpaper{cristin1958571,
title = {Using Gender- and Polarity-informed Models to Investigate Bias},
author = {Samia Touileb and Lilja Øvrelid and Erik Velldal},
url = {https://app.cristin.no/results/show.jsf?id=1958571, Cristin},
year = {2021},
date = {2021-01-01},
keywords = {},
pubstate = {published},
tppubtype = {workingpaper}
}
2020
Touileb, Samia; Øvrelid, Lilja; Velldal, Erik
Gender and sentiment, critics and authors: a dataset of Norwegian book reviews Journal Article
In: Gender Bias in Natural Language Processing. Association for Computational Linguistics, 2020, (Pre SFI).
@article{Touileb2020,
title = {Gender and sentiment, critics and authors: a dataset of Norwegian book reviews},
author = {Samia Touileb and Lilja Øvrelid and Erik Velldal},
url = {https://www.aclweb.org/anthology/2020.gebnlp-1.11.pdf},
year = {2020},
date = {2020-12-01},
journal = {Gender Bias in Natural Language Processing. Association for Computational Linguistics},
abstract = {Gender bias in models and datasets is widely studied in NLP. The focus has usually been on analysing how females and males express themselves, or how females and males are described. However, a less studied aspect is the combination of these two perspectives, how female and male describe the same or opposite gender. In this paper, we present a new gender annotated sentiment dataset of critics reviewing the works of female and male authors. We investigate if this newly annotated dataset contains differences in how the works of male and female authors are critiqued, in particular in terms of positive and negative sentiment. We also explore the differences in how this is done by male and female critics. We show that there are differences in how critics assess the works of authors of the same or opposite gender. For example, male critics rate crime novels written by females, and romantic and sentimental works written by males, more negatively.},
note = {Pre SFI},
keywords = {},
pubstate = {published},
tppubtype = {article}
}
Adouane, Wafia; Touileb, Samia; Bernardy, Jean-Philippe
Identifying Sentiments in Algerian Code-switched User-generated Comments Conference
2020, (Pre SFI).
@conference{Adouane2020,
title = {Identifying Sentiments in Algerian Code-switched User-generated Comments},
author = {Wafia Adouane and Samia Touileb and Jean-Philippe Bernardy},
url = {https://www.aclweb.org/anthology/2020.lrec-1.328.pdf},
year = {2020},
date = {2020-05-06},
journal = {Proceedings of the 12th Conference on Language Resources and Evaluation (LREC 2020)},
pages = {2698–2705},
abstract = {We present in this paper our work on Algerian language, an under-resourced North African colloquial Arabic variety, for which we
built a comparably large corpus of more than 36,000 code-switched user-generated comments annotated for sentiments. We opted
for this data domain because Algerian is a colloquial language with no existing freely available corpora. Moreover, we compiled
sentiment lexicons of positive and negative unigrams and bigrams reflecting the code-switches present in the language. We compare
the performance of four models on the task of identifying sentiments, and the results indicate that a CNN model trained end-to-end fits
better our unedited code-switched and unbalanced data across the predefined sentiment classes. Additionally, injecting the lexicons as
background knowledge to the model boosts its performance on the minority class with a gain of 10.54 points on the F-score. The results
of our experiments can be used as a baseline for future research for Algerian sentiment analysis.
},
note = {Pre SFI},
keywords = {},
pubstate = {published},
tppubtype = {conference}
}
built a comparably large corpus of more than 36,000 code-switched user-generated comments annotated for sentiments. We opted
for this data domain because Algerian is a colloquial language with no existing freely available corpora. Moreover, we compiled
sentiment lexicons of positive and negative unigrams and bigrams reflecting the code-switches present in the language. We compare
the performance of four models on the task of identifying sentiments, and the results indicate that a CNN model trained end-to-end fits
better our unedited code-switched and unbalanced data across the predefined sentiment classes. Additionally, injecting the lexicons as
background knowledge to the model boosts its performance on the minority class with a gain of 10.54 points on the F-score. The results
of our experiments can be used as a baseline for future research for Algerian sentiment analysis.
Lison, Pierre; Hubin, Aliaksandr; Barnes, Jeremy; Touileb, Samia
Named Entity Recognition without Labelled Data: A Weak Supervision Approach Journal Article
In: Proceedings of the 58th Annual Meeting of the Association for Computational Linguistics, pp. 1518–1533, 2020, (Pre SFI).
@article{Lison2020,
title = {Named Entity Recognition without Labelled Data: A Weak Supervision Approach},
author = {Pierre Lison and Aliaksandr Hubin and Jeremy Barnes and Samia Touileb},
url = {https://arxiv.org/pdf/2004.14723.pdf},
year = {2020},
date = {2020-04-30},
journal = {Proceedings of the 58th Annual Meeting of the Association for Computational Linguistics},
pages = {1518–1533},
abstract = {Named Entity Recognition (NER) performance often degrades rapidly when applied to target domains that differ from the texts observed during training. When in-domain labelled data is available, transfer learning techniques can be used to adapt existing NER models to the target domain. But what should one do when there is no hand-labelled data for the target domain? This paper presents a simple but powerful approach to learn NER models in the absence of labelled data through weak supervision. The approach relies on a broad spectrum of labelling functions to automatically annotate texts from the target domain. These annotations are then merged together using a hidden Markov model which captures the varying accuracies and confusions of the labelling functions. A sequence labelling model can finally be trained on the basis of this unified annotation. We evaluate the approach on two English datasets (CoNLL 2003 and news articles from Reuters and Bloomberg) and demonstrate an improvement of about 7 percentage points in entity-level F1 scores compared to an out-of-domain neural NER model.},
note = {Pre SFI},
keywords = {},
pubstate = {published},
tppubtype = {article}
}
2019
Barnes, Jeremy; Touileb, Samia; Øvrelid, Lilja; Velldal, Erik
Lexicon information in neural sentiment analysis: a multi-task learning approach Conference
Linköping University Electronic Press, 2019, (Pre SFI).
@conference{Barnes2019,
title = {Lexicon information in neural sentiment analysis: a multi-task learning approach},
author = {Jeremy Barnes and Samia Touileb and Lilja Øvrelid and Erik Velldal},
url = {https://www.aclweb.org/anthology/W19-6119.pdf},
year = {2019},
date = {2019-10-01},
journal = {Proceedings of the 22nd Nordic Conference on Computational Linguistics (NoDaLiDa)},
pages = {175–186},
publisher = {Linköping University Electronic Press},
abstract = {This paper explores the use of multi-task learning (MTL) for incorporating external knowledge in neural models. Specifically, we show how MTL can enable a BiLSTM sentiment classifier to incorporate information from sentiment lexicons. Our MTL set-up is shown to improve model performance (compared to a single-task set-up) on both English and Norwegian sentence-level sentiment datasets. The paper also introduces a new sentiment lexicon for Norwegian.},
note = {Pre SFI},
keywords = {},
pubstate = {published},
tppubtype = {conference}
}
2018
Velldal, Erik; Øvrelid, Lilja; Bergem, Eivind Alexander; Stadsnes, Cathrine; Touileb, Samia; Jørgensen, Fredrik
NoReC: The Norwegian Review Corpus Proceedings
2018, (Pre SFI).
@proceedings{Velldal2018,
title = {NoReC: The Norwegian Review Corpus},
author = {Erik Velldal and Lilja Øvrelid and Eivind Alexander Bergem and Cathrine Stadsnes and Samia Touileb and Fredrik Jørgensen},
year = {2018},
date = {2018-05-12},
abstract = {https://repo.clarino.uib.no/xmlui/handle/11509/124},
note = {Pre SFI},
keywords = {},
pubstate = {published},
tppubtype = {proceedings}
}
2017
Touileb, Samia; Pedersen, Truls; Sjøvaag, Helle
Automatic identification of unknown names with specific roles Journal Article
In: Proceedings of the Second Joint SIGHUM Workshop on Computational Linguistics for Cultural Heritage, Social Sciences, Humanities and Literature, pp. 150-158, 2017, (Pre SFI).
@article{Touileb2017,
title = {Automatic identification of unknown names with specific roles},
author = {Samia Touileb and Truls Pedersen and Helle Sjøvaag},
url = {https://www.aclweb.org/anthology/W18-4517.pdf},
year = {2017},
date = {2017-08-01},
journal = {Proceedings of the Second Joint SIGHUM Workshop on Computational Linguistics for Cultural Heritage, Social Sciences, Humanities and Literature},
pages = {150-158},
abstract = {Automatically identifying persons in a particular role within a large corpus can be a difficult task, especially if you don’t know who you are actually looking for. Resources compiling names of persons can be available, but no exhaustive lists exist. However, such lists usually contain known names that are “visible” in the national public sphere, and tend to ignore the marginal and international ones. In this article we propose a method for automatically generating suggestions of names found in a corpus of Norwegian news articles, and which “naturally” belong to a given initial list of members, and that were not known (compiled in a list) beforehand. The approach is based, in part, on the assumption that surface level syntactic features reveal parts of the underlying semantic content and can help uncover the structure of the language.},
note = {Pre SFI},
keywords = {},
pubstate = {published},
tppubtype = {article}
}