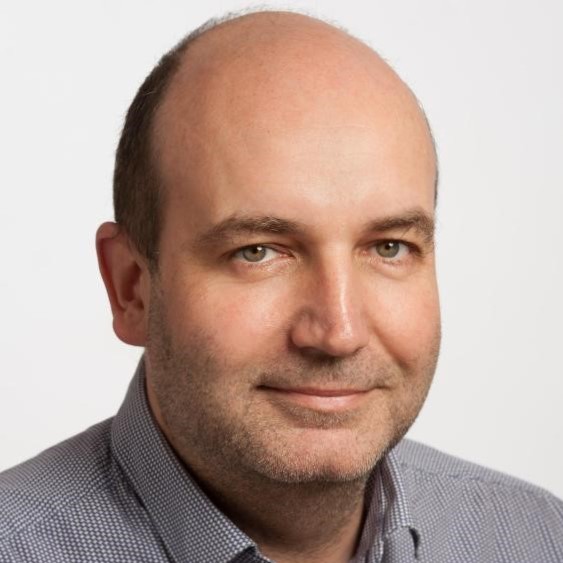
Prof. Dietmar Jannach
Work Package Advisor and Key Researcher
2024
Klimashevskaia, Anastasiia; Elahi, Mehdi; Jannach, Dietmar; Trattner, Christoph; Buodd, Simen
Empowering Editors: How Automated Recommendations Support Editorial Article Curation Workshop
RecSys 2024, INRA workshop, 2024.
@workshop{empoweringANA24,
title = {Empowering Editors: How Automated Recommendations Support Editorial Article Curation},
author = {Anastasiia Klimashevskaia and Mehdi Elahi and Dietmar Jannach and Christoph Trattner and Simen Buodd},
url = {https://mediafutures.no/recsys2024-workshops_paper_119-3/},
year = {2024},
date = {2024-10-01},
urldate = {2024-10-01},
booktitle = {RecSys 2024, INRA workshop},
issue = {RecSys 2024, INRA workshop},
abstract = {The application of recommender systems in the news domain has experienced rapid growth in recent years. Various news outlets are proposing a full automation of a newspaper front page through automated recommendation. In this paper, however, we explore the synergy of editorial and algorithmic news curation by analyzing the front page of a real-world news platform, where news articles are either selected automatically by a recommendation algorithm or are selected manually by editors. An investigation of the interaction log data from an online newspaper revealed that while the editorial staff is focusing on content that is generally popular across large parts of the audience, the algorithmic curation can, in addition, provide small yet noteworthy personalization touches for individual readers. The results of the analysis demonstrate an example of a successful coexistence of editorial and algorithmic news curation.},
howpublished = {RecSys 2024, INRA workshop},
keywords = {},
pubstate = {published},
tppubtype = {workshop}
}
Klimashevskaia, Anastasiia; Jannach, Dietmar; Elahi, Mehdi; Trattner, Christoph
A Survey on Popularity Bias in Recommender Systems Journal Article Forthcoming
In: User Modeling and User-Adapted Interaction (UMUAI), Forthcoming.
@article{biasanas24,
title = {A Survey on Popularity Bias in Recommender Systems},
author = {Anastasiia Klimashevskaia and Dietmar Jannach and Mehdi Elahi and Christoph Trattner},
url = {https://mediafutures.no/popularitybias_literature_review-5/},
year = {2024},
date = {2024-06-13},
journal = {User Modeling and User-Adapted Interaction (UMUAI)},
abstract = {Recommender systems help people find relevant content in a personalized way. One main promise of such systems is that they are able to increase the visibility of items in the long tail, i.e., the lesser-
known items in a catalogue. Existing research, however, suggests that in many situations today’s recommendation algorithms instead exhibit a popularity bias, meaning that they often focus on rather popular items in their recommendations. Such a bias may not only lead to the limited value of the recommendations for consumers and providers in the short run, but it may also cause undesired reinforcement effects over time. In this paper, we discuss the potential reasons for popularity bias and review existing approaches to detect, quantify and mitigate popularity bias in
recommender systems. Our survey, therefore, includes both an overview
of the computational metrics used in the literature as well as a review of the main technical approaches to reduce the bias. Furthermore, we critically discuss today’s literature, where we observe that the research is almost entirely based on computational experiments and on certain
assumptions regarding the practical effects of including long-tail items in the recommendations.},
keywords = {},
pubstate = {forthcoming},
tppubtype = {article}
}
known items in a catalogue. Existing research, however, suggests that in many situations today’s recommendation algorithms instead exhibit a popularity bias, meaning that they often focus on rather popular items in their recommendations. Such a bias may not only lead to the limited value of the recommendations for consumers and providers in the short run, but it may also cause undesired reinforcement effects over time. In this paper, we discuss the potential reasons for popularity bias and review existing approaches to detect, quantify and mitigate popularity bias in
recommender systems. Our survey, therefore, includes both an overview
of the computational metrics used in the literature as well as a review of the main technical approaches to reduce the bias. Furthermore, we critically discuss today’s literature, where we observe that the research is almost entirely based on computational experiments and on certain
assumptions regarding the practical effects of including long-tail items in the recommendations.
Diakopoulos, Nicholas; Trattner, Christoph; Jannach, Dietmar; Meijer, Irene Costera; Motta, Enrico
Leveraging Professional Ethics for Responsible AI: Applying AI techniques to journalism. Journal Article
In: Communications of the ACM, 2024.
@article{leverag24,
title = {Leveraging Professional Ethics for Responsible AI: Applying AI techniques to journalism.},
author = {Nicholas Diakopoulos and Christoph Trattner and Dietmar Jannach and Irene Costera Meijer and Enrico Motta},
url = {https://mediafutures.no/3625252/https://mediafutures.no/3625252-1/},
year = {2024},
date = {2024-01-23},
urldate = {2024-01-23},
journal = {Communications of the ACM},
keywords = {},
pubstate = {published},
tppubtype = {article}
}
2023
Klimashevskaia, Anastasiia; Elahi, Mehdi; Jannach, Dietmar; Skjærven, Lars; Tessem, Astrid; Trattner, Christoph
Evaluating The Effects of Calibrated Popularity Bias Mitigation: A Field Study Conference
Association for Computing Machinery (ACM) RecSys ’23, 2023.
@conference{RecSys_2023_LBR,
title = {Evaluating The Effects of Calibrated Popularity Bias Mitigation: A Field Study},
author = {Anastasiia Klimashevskaia and Mehdi Elahi and Dietmar Jannach and Lars Skjærven and Astrid Tessem and Christoph Trattner},
url = {https://mediafutures.no/recsys_2023_lbr/},
year = {2023},
date = {2023-09-18},
booktitle = {Association for Computing Machinery (ACM) RecSys ’23},
keywords = {},
pubstate = {published},
tppubtype = {conference}
}
2022
Bogina, Veronica; Kuflik, Tsvi; Jannach, Dietmar; Bielikova, Maria; Kompan, Michal; Trattner, Christoph
Considering Temporal Aspects in Recommender Systems: A Survey Journal Article
In: UMUAI journal, 2022.
@article{Bogina2022,
title = {Considering Temporal Aspects in Recommender Systems: A Survey },
author = {Veronica Bogina and Tsvi Kuflik and Dietmar Jannach and Maria Bielikova and Michal Kompan and Christoph Trattner
},
url = {https://mediafutures.no/revisedversion_considering_temporal_aspects_in_rs_a_survey-6/},
year = {2022},
date = {2022-05-31},
urldate = {2022-05-31},
journal = {UMUAI journal},
abstract = {The widespread use of temporal aspects in user modeling indicates their importance, and their consideration showed to be highly effective in var- ious domains related to user modeling, especially in recommender systems. Still, past and ongoing research, spread over several decades, provided multi- ple ad-hoc solutions, but no common understanding of the issue. There is no standardization and there is often little commonality in considering tempo- ral aspects in different applications. This may ultimately lead to the problem that application developers define ad-hoc solutions for their problems at hand, sometimes missing or neglecting aspects that proved to be effective in similar cases. Therefore, a comprehensive survey of the consideration of temporal as- pects in recommender systems is required. In this work, we provide an overview of various time-related aspects, categorize existing research, present a tempo- ral abstraction and point to gaps that require future research. We anticipate this survey will become a reference point for researchers and practitioners alike when considering the potential application of temporal aspects in their personalized applications.},
keywords = {},
pubstate = {published},
tppubtype = {article}
}
2021
Trattner, Christoph; Jannach, Dietmar; Motta, Enrico; Meijer, Irene Costera; Diakopoulos, Nicholas; Elahi, Mehdi; Opdahl, Andreas L.; Tessem, Bjørnar; Borch, Njål; Fjeld, Morten; Øvrelid, Lilja; Smedt, Koenraad De; Moe, Hallvard
Responsible media technology and AI: challenges and research directions Journal Article
In: AI and Ethics, 2021.
@article{cristin2000622,
title = {Responsible media technology and AI: challenges and research directions},
author = {Christoph Trattner and Dietmar Jannach and Enrico Motta and Irene Costera Meijer and Nicholas Diakopoulos and Mehdi Elahi and Andreas L. Opdahl and Bjørnar Tessem and Njål Borch and Morten Fjeld and Lilja Øvrelid and Koenraad De Smedt and Hallvard Moe},
url = {https://app.cristin.no/results/show.jsf?id=2000622, Cristin
https://link.springer.com/content/pdf/10.1007/s43681-021-00126-4.pdf},
doi = {https://doi.org/10.1007/s43681-021-00126-4},
year = {2021},
date = {2021-12-20},
urldate = {2021-12-20},
journal = {AI and Ethics},
keywords = {},
pubstate = {published},
tppubtype = {article}
}
Elahi, Mehdi; Jannach, Dietmar; Skjærven, Lars; Knudsen, Erik; Sjøvaag, Helle; Tolonen, Kristian; Holmstad, Øyvind; Pipkin, Igor; Throndsen, Eivind; Stenbom, Agnes; Fiskerud, Eivind; Oesch, Adrian; Vredenberg, Loek; Trattner, Christoph
Towards Responsible Media Recommendation Journal Article
In: AI and Ethics, 2021.
@article{cristin1953352,
title = {Towards Responsible Media Recommendation},
author = {Mehdi Elahi and Dietmar Jannach and Lars Skjærven and Erik Knudsen and Helle Sjøvaag and Kristian Tolonen and Øyvind Holmstad and Igor Pipkin and Eivind Throndsen and Agnes Stenbom and Eivind Fiskerud and Adrian Oesch and Loek Vredenberg and Christoph Trattner},
url = {https://app.cristin.no/results/show.jsf?id=1953352, Cristin
https://link.springer.com/article/10.1007%2Fs43681-021-00107-7},
doi = {https://doi.org/10.1007/s43681-021-00107-7},
year = {2021},
date = {2021-11-02},
journal = {AI and Ethics},
keywords = {},
pubstate = {published},
tppubtype = {article}
}
Jannach, Dietmar; Jesse, Mathias; Jugovac, Michael; Trattner, Christoph
Exploring Multi-List User Interfaces for Similar-Item Recommendations Conference
29th ACM International Conference on User Modeling, Adaptation and Personalization (UMAP '21) 2021.
@conference{Jannach2021,
title = {Exploring Multi-List User Interfaces for Similar-Item Recommendations},
author = {Dietmar Jannach and Mathias Jesse and Michael Jugovac and Christoph Trattner},
url = {https://mediafutures.no/conference_umap_2021-2/},
year = {2021},
date = {2021-03-26},
organization = {29th ACM International Conference on User Modeling, Adaptation and Personalization (UMAP '21)},
abstract = {On many e-commerce and media streaming sites, the user inter-face (UI) consists of multiple lists of item suggestions. The itemsin each list are usually chosen based on pre-defined strategies and,e.g., show movies of the same genre or category. Such interfacesare common in practice, but there is almost no academic researchregarding the optimal design and arrangement of such multi-listUIs for recommenders. In this paper, we report the results of anexploratory user study that examined the effects of various designalternatives on the decision-making behavior of users in the con-text of similar-item recommendations. Our investigations showed,among other aspects, that decision-making is slower and more de-manding with multi-list interfaces, but that users also explore moreoptions before making a decision. Regarding the selection of thealgorithm to retrieve similar items, our study furthermore revealsthe importance of considering social-based similarity measures.},
keywords = {},
pubstate = {published},
tppubtype = {conference}
}
2020
Nunes, Ingrid; Jannach, Dietmar
A Systematic Review and Taxonomy of Explanations in Decision Support and Recommender Systems Journal Article
In: User-Modeling and User-Adapted Interaction, vol. 27, no. 3-5, pp. 393-444, 2020, (Pre SFI).
@article{Nunes2020,
title = {A Systematic Review and Taxonomy of Explanations in Decision Support and Recommender Systems},
author = {Ingrid Nunes and Dietmar Jannach},
url = {https://arxiv.org/pdf/2006.08672.pdf},
doi = {10.1007/s11257-017-9195-0},
year = {2020},
date = {2020-06-15},
journal = {User-Modeling and User-Adapted Interaction},
volume = {27},
number = {3-5},
pages = {393-444},
abstract = {With the recent advances in the field of artificial intelligence, an increasing number of decision-making tasks are delegated to software systems. A key requirement for the success and adoption of such systems is that users must trust system choices or even fully automated decisions. To achieve this, explanation facilities have been widely investigated as a means of establishing trust in these systems since the early years of expert systems. With today's increasingly sophisticated machine learning algorithms, new challenges in the context of explanations, accountability, and trust towards such systems constantly arise. In this work, we systematically review the literature on explanations in advice-giving systems. This is a family of systems that includes recommender systems, which is one of the most successful classes of advice-giving software in practice. We investigate the purposes of explanations as well as how they are generated, presented to users, and evaluated. As a result, we derive a novel comprehensive taxonomy of aspects to be considered when designing explanation facilities for current and future decision support systems. The taxonomy includes a variety of different facets, such as explanation objective, responsiveness, content and presentation. Moreover, we identified several challenges that remain unaddressed so far, for example related to fine-grained issues associated with the presentation of explanations and how explanation facilities are evaluated.},
note = {Pre SFI},
keywords = {},
pubstate = {published},
tppubtype = {article}
}
2019
Trattner, Christoph; Jannach, Dietmar
Learning to Recommend Similar Items from Human Judgements Journal Article
In: User Modeling and User-Adapted Interaction Journal, pp. 1-50, 2019, (Pre SFI).
@article{Trattner2020,
title = {Learning to Recommend Similar Items from Human Judgements},
author = {Christoph Trattner and Dietmar Jannach},
url = {https://www.christophtrattner.info/pubs/UMUAI2019.pdf},
doi = {10.1007/s11257-019-09245-4},
year = {2019},
date = {2019-09-20},
journal = {User Modeling and User-Adapted Interaction Journal},
pages = {1-50},
abstract = {Similar item recommendations—a common feature of many Web sites—point users to other interesting objects given a currently inspected item. A common way of computing such recommendations is to use a similarity function, which expresses how much alike two given objects are. Such similarity functions are usually designed based on the specifics of the given application domain. In this work, we explore how such functions can be learned from human judgments of similarities between objects, using two domains of “quality and taste”—cooking recipe and movie recommendation—as guiding scenarios. In our approach, we first collect a few thousand pairwise similarity assessments with the help of crowdworkers. Using these data, we then train different machine learning models that can be used as similarity functions to compare objects. Offline analyses reveal for both application domains that models that combine different types of item characteristics are the best predictors for human-perceived similarity. To further validate the usefulness of the learned models, we conducted additional user studies. In these studies, we exposed participants to similar item recommendations using a set of models that were trained with different feature subsets. The results showed that the combined models that exhibited the best offline prediction performance led to the highest user-perceived similarity, but also to recommendations that were considered useful by the participants, thus confirming the feasibility of our approach.},
note = {Pre SFI},
keywords = {},
pubstate = {published},
tppubtype = {article}
}
Dacrema, Maurizio Ferrari; Cremonesi, Paolo; Jannach, Dietmar
Are We Really Making Much Progress? A Worrying Analysis of Recent Neural Recommendation Approaches Conference
Proceedings of the 2019 ACM Conference on Recommender Systems (RecSys 2019), Copenhagen, 2019, (Pre SFI).
@conference{Dacrema2019,
title = {Are We Really Making Much Progress? A Worrying Analysis of Recent Neural Recommendation Approaches},
author = {Maurizio Ferrari Dacrema and Paolo Cremonesi and Dietmar Jannach},
url = {https://arxiv.org/pdf/1907.06902.pdf},
year = {2019},
date = {2019-08-16},
booktitle = {Proceedings of the 2019 ACM Conference on Recommender Systems (RecSys 2019)},
address = {Copenhagen},
abstract = {Deep learning techniques have become the method of choice for
researchers working on algorithmic aspects of recommender systems. With the strongly increased interest in machine learning in
general, it has, as a result, become difficult to keep track of what
represents the state-of-the-art at the moment, e.g., for top-n recommendation tasks. At the same time, several recent publications
point out problems in today’s research practice in applied machine
learning, e.g., in terms of the reproducibility of the results or the
choice of the baselines when proposing new models.
In this work, we report the results of a systematic analysis of algorithmic proposals for top-n recommendation tasks. Specifically,
we considered 18 algorithms that were presented at top-level research conferences in the last years. Only 7 of them could be reproduced with reasonable effort. For these methods, it however
turned out that 6 of them can often be outperformed with comparably simple heuristic methods, e.g., based on nearest-neighbor or
graph-based techniques. The remaining one clearly outperformed
the baselines but did not consistently outperform a well-tuned nonneural linear ranking method. Overall, our work sheds light on a
number of potential problems in today’s machine learning scholarship and calls for improved scientific practices in this area.},
note = {Pre SFI},
keywords = {},
pubstate = {published},
tppubtype = {conference}
}
researchers working on algorithmic aspects of recommender systems. With the strongly increased interest in machine learning in
general, it has, as a result, become difficult to keep track of what
represents the state-of-the-art at the moment, e.g., for top-n recommendation tasks. At the same time, several recent publications
point out problems in today’s research practice in applied machine
learning, e.g., in terms of the reproducibility of the results or the
choice of the baselines when proposing new models.
In this work, we report the results of a systematic analysis of algorithmic proposals for top-n recommendation tasks. Specifically,
we considered 18 algorithms that were presented at top-level research conferences in the last years. Only 7 of them could be reproduced with reasonable effort. For these methods, it however
turned out that 6 of them can often be outperformed with comparably simple heuristic methods, e.g., based on nearest-neighbor or
graph-based techniques. The remaining one clearly outperformed
the baselines but did not consistently outperform a well-tuned nonneural linear ranking method. Overall, our work sheds light on a
number of potential problems in today’s machine learning scholarship and calls for improved scientific practices in this area.
2018
Ludewig, Malte; Jannach, Dietmar
Evaluation of Session-based Recommendation Algorithms Journal Article
In: User-Modeling and User-Adapted Interaction, vol. 28, no. 4-5, pp. 331-390, 2018, (Pre SFI).
@article{Ludewig2018,
title = {Evaluation of Session-based Recommendation Algorithms},
author = {Malte Ludewig and Dietmar Jannach},
url = {https://arxiv.org/pdf/1803.09587.pdf},
doi = {10.1007/s11257-018-9209-6},
year = {2018},
date = {2018-03-26},
journal = {User-Modeling and User-Adapted Interaction},
volume = {28},
number = {4-5},
pages = {331-390},
abstract = {Recommender systems help users find relevant items of interest, for example on e-commerce or media streaming sites. Most academic research is concerned with approaches that personalize the recommendations according to long-term user profiles. In many real-world applications, however, such long-term profiles often do not exist and recommendations therefore have to be made solely based on the observed behavior of a user during an ongoing session. Given the high practical relevance of the problem, an increased interest in this problem can be observed in recent years, leading to a number of proposals for session-based recommendation algorithms that typically aim to predict the user's immediate next actions. In this work, we present the results of an in-depth performance comparison of a number of such algorithms, using a variety of datasets and evaluation measures. Our comparison includes the most recent approaches based on recurrent neural networks like GRU4REC, factorized Markov model approaches such as FISM or FOSSIL, as well as simpler methods based, e.g., on nearest neighbor schemes. Our experiments reveal that algorithms of this latter class, despite their sometimes almost trivial nature, often perform equally well or significantly better than today's more complex approaches based on deep neural networks. Our results therefore suggest that there is substantial room for improvement regarding the development of more sophisticated session-based recommendation algorithms.},
note = {Pre SFI},
keywords = {},
pubstate = {published},
tppubtype = {article}
}
Quadrana, Massimo; Cremonesi, Paolo; Jannach, Dietmar
Sequence-Aware Recommender Systems Journal Article
In: ACM Computing Surveys, vol. 51, no. 4, pp. 1-35, 2018, (Pre SFI).
@article{Quadrana2018,
title = {Sequence-Aware Recommender Systems},
author = {Massimo Quadrana and Paolo Cremonesi and Dietmar Jannach},
url = {https://arxiv.org/pdf/1802.08452.pdf},
year = {2018},
date = {2018-02-23},
journal = {ACM Computing Surveys},
volume = {51},
number = {4},
pages = {1-35},
abstract = {Recommender systems are one of the most successful applications of data mining and machine learning technology in practice. Academic research in the field is historically often based on the matrix completion problem formulation, where for each user-item-pair only one interaction (e.g., a rating) is considered. In many application domains, however, multiple user-item interactions of different types can be recorded over time. And, a number of recent works have shown that this information can be used to build richer individual user models and to discover additional behavioral patterns that can be leveraged in the recommendation process. In this work we review existing works that consider information from such sequentially-ordered user- item interaction logs in the recommendation process. Based on this review, we propose a categorization of the corresponding recommendation tasks and goals, summarize existing algorithmic solutions, discuss methodological approaches when benchmarking what we call sequence-aware recommender systems, and outline open challenges in the area.},
note = {Pre SFI},
keywords = {},
pubstate = {published},
tppubtype = {article}
}
2016
Jannach, Dietmar; Tuzhilin, Alexander; Zanker, Markus
Recommender Systems - Beyond Matrix Completion Journal Article
In: Communications of the ACM, vol. 59, no. 11, pp. 94-102, 2016, (Pre SFI).
@article{Jannach2016,
title = {Recommender Systems - Beyond Matrix Completion},
author = {Dietmar Jannach and Alexander Tuzhilin and Markus Zanker },
url = {https://www.researchgate.net/publication/309600906_Recommender_systems---_beyond_matrix_completion},
doi = {10.1145/2891406},
year = {2016},
date = {2016-10-01},
journal = {Communications of the ACM},
volume = {59},
number = {11},
pages = {94-102},
abstract = {Recommender systems have become a natural part of the user experience in today's online world. These systems are able to deliver value both for users and providers and are one prominent example where the output of academic research has a direct impact on the advancements in industry. In this article, we have briefy reviewed the history of this multidis-ciplinary field and looked at recent efforts in the research community to consider the variety of factors that may influence the long-term success of a recommender system. The list of open issues and success factors is still far from complete and new challenges arise constantly that require further research. For example, the huge amounts of user data and preference signals that become available through the Social Web and the Internet of Things not only leads to technical challenges such as scalability, but also to societal questions concerning user privacy. Based on our reflections on the developments in the field, we finally emphasize the need for a more holistic research approach that combines the insights of different disciplines. We urge that research focuses even more on practical problems that matter and are truly suited to increase the utility of recommendations from the viewpoint of the users.
},
note = {Pre SFI},
keywords = {},
pubstate = {published},
tppubtype = {article}
}