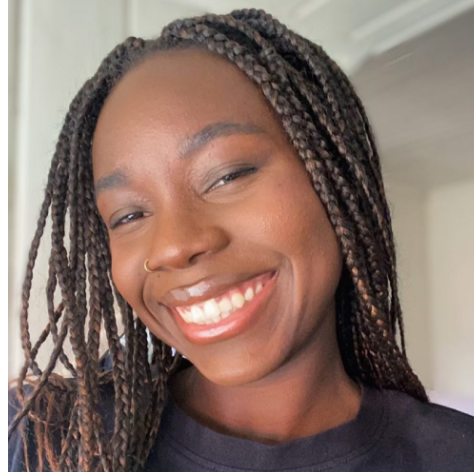
Gloria Anne Babile Kasangu
Research Assistant
University of Bergen
Gloria Anne Babile Kasangu is a Research Assistant at MediaFutures. She’s currently undertaking a Bachelor’s degree in Information Science at the University of Bergen, and holds a Bachelor’s degree in General Psychology. When she’s not studying or working, she enjoys cooking, writing, and working out.
2024
Jeng, Jia Hua; Kasangu, Gloria Anne Babile; Starke, Alain D.; Knudsen, Erik; Trattner, Christoph
Negativity Sells? Using an LLM to Affectively Reframe News Articles in a Recommender System Workshop
2024.
@workshop{negativ24,
title = {Negativity Sells? Using an LLM to Affectively Reframe News Articles in a Recommender System},
author = {Jia Hua Jeng and Gloria Anne Babile Kasangu and Alain D. Starke and Erik Knudsen and Christoph Trattner},
url = {https://mediafutures.no/inra_jeng/},
year = {2024},
date = {2024-10-30},
issue = {RecSys2024 - INRA workshop},
abstract = {Recent developments in artificial intelligence allow newsrooms to automate journalistic choices and processes. In doing so, news framing can impact people's engagement with news media, as well as their willingness to pay for news articles. Large Language Models (LLMs) can be used as a framing tool, aligning headlines with a news website user's preferences or state. It is, however, unknown how users perceive and experience the use of a platform with such LLM-reframed news headlines. We present the results of a user study (N = 300) with a news recommender system (NRS). Users had to read three news articles from The Washington Post from a preferred category (abortion, economics, gun control). Headlines were rewritten by an LLM (ChatGPT-4) and images were replaced in specific affective styles, across 2 (positive or negative headlines) x 3 (positive or negative image, or no image) between-subject framing conditions. We found that negatively framed images and text elicited negative emotions, while positive framing had little effect. Users were also more willing to pay for a news service when facing negatively framed headlines and images. Surprisingly, the congruency between text and image (i.e., both being framed negatively or positively) did not significantly impact engagement. We discuss how this study can shape further research on affective framing in news recommender systems and how such applications could impact journalism practices.},
keywords = {},
pubstate = {published},
tppubtype = {workshop}
}
Recent developments in artificial intelligence allow newsrooms to automate journalistic choices and processes. In doing so, news framing can impact people's engagement with news media, as well as their willingness to pay for news articles. Large Language Models (LLMs) can be used as a framing tool, aligning headlines with a news website user's preferences or state. It is, however, unknown how users perceive and experience the use of a platform with such LLM-reframed news headlines. We present the results of a user study (N = 300) with a news recommender system (NRS). Users had to read three news articles from The Washington Post from a preferred category (abortion, economics, gun control). Headlines were rewritten by an LLM (ChatGPT-4) and images were replaced in specific affective styles, across 2 (positive or negative headlines) x 3 (positive or negative image, or no image) between-subject framing conditions. We found that negatively framed images and text elicited negative emotions, while positive framing had little effect. Users were also more willing to pay for a news service when facing negatively framed headlines and images. Surprisingly, the congruency between text and image (i.e., both being framed negatively or positively) did not significantly impact engagement. We discuss how this study can shape further research on affective framing in news recommender systems and how such applications could impact journalism practices.
Jeng, Jia Hua; Kasangu, Gloria Anne Babile; Starke, Alain D.; Trattner, Christoph
Emotional Reframing of Economic News using a Large Language Model Conference
ACM UMAP 2024, 2024.
@conference{emorefram24,
title = {Emotional Reframing of Economic News using a Large Language Model},
author = {Jia Hua Jeng and Gloria Anne Babile Kasangu and Alain D. Starke and Christoph Trattner},
url = {https://mediafutures.no/umap2024___jeng_alain_gloria_christoph__workshop_-3/},
year = {2024},
date = {2024-07-01},
urldate = {2024-07-01},
booktitle = {ACM UMAP 2024},
abstract = {News media framing can shape public perception and potentially polarize views. Emotional language can exacerbate these framing effects, as a user’s emotional state can be an important contextual factor to use in news recommendation. Our research explores the relation between emotional framing techniques and the emotional states of readers, as well as readers’ perceived trust in specific news articles. Users (N = 200) had to read three economic news articles from the Washington Post. We used ChatGPT-4 to reframe news articles with specific emotional languages (Anger, Fear, Hope), compared to a neutral baseline reframed by a human journalist. Our results revealed that negative framing (Anger, Fear) elicited stronger negative emotional states among users than the neutral baseline, while Hope led to little changes overall. In contrast, perceived trust levels varied little across the different conditions. We discuss the implications of our findings and how emotional framing could affect societal polarization issues},
keywords = {},
pubstate = {published},
tppubtype = {conference}
}
News media framing can shape public perception and potentially polarize views. Emotional language can exacerbate these framing effects, as a user’s emotional state can be an important contextual factor to use in news recommendation. Our research explores the relation between emotional framing techniques and the emotional states of readers, as well as readers’ perceived trust in specific news articles. Users (N = 200) had to read three economic news articles from the Washington Post. We used ChatGPT-4 to reframe news articles with specific emotional languages (Anger, Fear, Hope), compared to a neutral baseline reframed by a human journalist. Our results revealed that negative framing (Anger, Fear) elicited stronger negative emotional states among users than the neutral baseline, while Hope led to little changes overall. In contrast, perceived trust levels varied little across the different conditions. We discuss the implications of our findings and how emotional framing could affect societal polarization issues