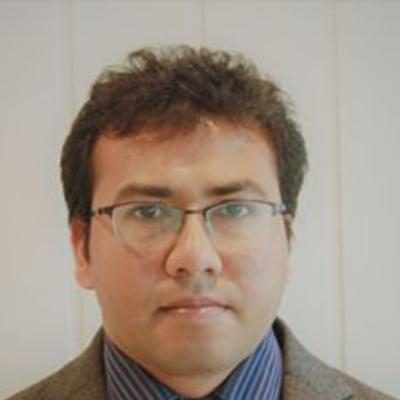
Assoc Prof. Fazle Rabbi
Work Package Co-Leader and Task Leader
2024
Tarekegn, Adane Nega; Rabbi, Fazle; Tessem, Bjørnar
Large Language Model Enhanced Clustering for News Event Detection Conference Forthcoming
AIMEDIA : AI-based Media Disruption and Transformation, Forthcoming.
@conference{newseventdec24,
title = {Large Language Model Enhanced Clustering for News Event Detection},
author = {Adane Nega Tarekegn and Fazle Rabbi and Bjørnar Tessem},
url = {https://mediafutures.no/1_aimedia_paper_cr_version-1/},
year = {2024},
date = {2024-09-02},
booktitle = {AIMEDIA : AI-based Media Disruption and Transformation},
abstract = {The news landscape is continuously evolving, with an ever-increasing volume of information from around the world. Automated event detection within this vast data repository is crucial for monitoring, identifying, and categorizing significant news occurrences across diverse platforms. This paper presents an event detection framework that leverages Large Language Models (LLMs) combined with clustering analysis to detect news events from the Global Database of Events, Language, and Tone (GDELT). The framework enhances event clustering through both pre-event detection tasks (keyword extraction and text embedding) and post-event detection tasks (event summarization and topic labeling). We also evaluate the impact of various textual embeddings on the quality of clustering outcomes, ensuring robust news categorization. Additionally, we introduce a novel Cluster Stability Assessment Index (CSAI) to assess the validity and robustness of clustering results. CSAI utilizes latent feature vectors to provide a new way of measuring clustering quality. Our experiments indicate that combining LLM embeddings with clustering algorithms yields the best results, demonstrating greater robustness in terms of CSAI scores. Moreover, post-event detection tasks generate meaningful insights, facilitating effective interpretation of event clustering results. Overall, our findings indicate that the proposed framework offers valuable insights and could enhance the accuracy and depth of news reporting.},
keywords = {},
pubstate = {forthcoming},
tppubtype = {conference}
}
2023
Fatemi, Bahareh; Rabbi, Fazle; Opdahl, Andreas L.
Evaluating the Effectiveness of GPT Large Language Model for News Classification in the IPTC News Ontology Journal Article
In: IEEE Access, 2023.
@article{GPTLangMo,
title = {Evaluating the Effectiveness of GPT Large Language Model for News Classification in the IPTC News Ontology},
author = {Bahareh Fatemi and Fazle Rabbi and Andreas L. Opdahl },
url = {https://mediafutures.no/evaluating_the_effectiveness_of_gpt_large_language_model_for_news_classification_in_the_iptc_news_ontology/},
year = {2023},
date = {2023-12-21},
journal = {IEEE Access},
abstract = {News classification plays a vital role in newsrooms, as it involves the time-consuming task
of categorizing news articles and requires domain knowledge. Effective news classification is essential
for categorizing and organizing a constant flow of information, serving as the foundation for subsequent
tasks, such as news aggregation, monitoring, filtering, and organization. The automation of this process can
significantly benefit newsrooms by saving time and resources. In this study, we explore the potential of the
GPT large language model in a zero-shot setting for multi-class classification of news articles within the
widely accepted International Press Telecommunications Council (IPTC) news ontology. The IPTC news
ontology provides a structured framework for categorizing news, facilitating the efficient organization and
retrieval of news content. By investigating the effectiveness of the GPT language model in this classification
task, we aimed to understand its capabilities and potential applications in the news domain. This study was
conducted as part of our ongoing research in the field of automated journalism.},
keywords = {},
pubstate = {published},
tppubtype = {article}
}
of categorizing news articles and requires domain knowledge. Effective news classification is essential
for categorizing and organizing a constant flow of information, serving as the foundation for subsequent
tasks, such as news aggregation, monitoring, filtering, and organization. The automation of this process can
significantly benefit newsrooms by saving time and resources. In this study, we explore the potential of the
GPT large language model in a zero-shot setting for multi-class classification of news articles within the
widely accepted International Press Telecommunications Council (IPTC) news ontology. The IPTC news
ontology provides a structured framework for categorizing news, facilitating the efficient organization and
retrieval of news content. By investigating the effectiveness of the GPT language model in this classification
task, we aimed to understand its capabilities and potential applications in the news domain. This study was
conducted as part of our ongoing research in the field of automated journalism.
Rabbi, Fazle; Fatemi, Bahareh; Lamo, Yngve; Opdahl, Andreas L.
A model-based framework for NEWS content analysis Journal Article
In: 12th International Conference on Model-Based Software and Systems Engineering, 2023.
@article{modelBased23,
title = {A model-based framework for NEWS content analysis},
author = {Fazle Rabbi and Bahareh Fatemi and Yngve Lamo and Andreas L. Opdahl},
url = {https://mediafutures.no/news-content-analysis/},
year = {2023},
date = {2023-12-12},
urldate = {2023-12-12},
journal = {12th International Conference on Model-Based Software and Systems Engineering},
abstract = {News articles are published all over the world to cover important events. Journalists need to keep track of
ongoing events in a fair and accountable manner and analyze them for newsworthiness. It requires enormous
amount of time for journalists to process information coming from main stream news media, social media
from all over the world as well as policy and law circulated by governments and international organizations.
News articles published by different news providers may consist of subjectivity of the reporters due to the
influence of reporters’ backgrounds, world views and opinions. In today’s practice of journalism there is a
lack of computational methods to support journalists to investigate fairness and monitor and analyze large
massive information streams. In this paper we present a model based approach to analyze the perspectives of
news publishers and monitor the progression of news events from various perspective. The domain concepts
in the news domain such as the news events and their contextual information is represented across various
dimensions in a knowledge graph. We presented a multi dimensional comparative analysis method of news
events for analyzing news article variants and for uncovering underlying storylines. To show the applicability
of the proposed method in real life, we demonstrated a running example in this paper. The utilization of
a model-based approach ensures the adaptability of our proposed method for representing a wide array of
domain concepts within the news domain.},
keywords = {},
pubstate = {published},
tppubtype = {article}
}
ongoing events in a fair and accountable manner and analyze them for newsworthiness. It requires enormous
amount of time for journalists to process information coming from main stream news media, social media
from all over the world as well as policy and law circulated by governments and international organizations.
News articles published by different news providers may consist of subjectivity of the reporters due to the
influence of reporters’ backgrounds, world views and opinions. In today’s practice of journalism there is a
lack of computational methods to support journalists to investigate fairness and monitor and analyze large
massive information streams. In this paper we present a model based approach to analyze the perspectives of
news publishers and monitor the progression of news events from various perspective. The domain concepts
in the news domain such as the news events and their contextual information is represented across various
dimensions in a knowledge graph. We presented a multi dimensional comparative analysis method of news
events for analyzing news article variants and for uncovering underlying storylines. To show the applicability
of the proposed method in real life, we demonstrated a running example in this paper. The utilization of
a model-based approach ensures the adaptability of our proposed method for representing a wide array of
domain concepts within the news domain.
Fatemi, Bahareh; Rabbi, Fazle; Tessem, Bjørnar
Fairness in automated data journalism systems Journal Article
In: NIKT: Norsk IKT-konferanse for forskning og utdanning, 2023.
@article{nokeyg,
title = {Fairness in automated data journalism systems},
author = {Bahareh Fatemi and Fazle Rabbi and Bjørnar Tessem},
url = {https://www.researchgate.net/publication/365127564_Fairness_in_automated_data_journalism_systems},
doi = {10.13140/RG.2.2.30374.19522},
year = {2023},
date = {2023-03-09},
urldate = {2023-03-09},
journal = {NIKT: Norsk IKT-konferanse for forskning og utdanning},
abstract = {Automated data journalism is an application of computing and artificial intelligence (AI) that aims to create stories from raw data, possibly in a variety of formats (such as visuals or text). Conventionally, a variety of methodologies and tools, including statistical software packages and data visualization tools have been used to generate stories from raw data. Artificial intelligence, and particularly machine learning techniques have recently been introduced because they can handle more complex data and scale more easily to larger datasets. However, AI techniques may raise a number of ethical concerns such as an unfair presentation which typically occurs due to bias. Stories that contains unfair presentation could be destructive at individual and societal levels; they could also damage the reputation of news providers. In this paper we study an existing framework of automated journalism and enhance the framework to make it aware of fairness concern. We present various steps of the framework where bias enters into the production of a story and address the causes and effects of different types of biases.},
keywords = {},
pubstate = {published},
tppubtype = {article}
}
Khan, Sohail Ahmed; Sheikhi, Ghazaal; Opdahl, Andreas L.; Rabbi, Fazle; Stoppel, Sergej; Trattner, Christoph; Dang-Nguyen, Duc-Tien
Visual User-Generated Content Verification in Journalism: An Overview Journal Article
In: IEEE Access, 2023.
@article{KHAN2023,
title = {Visual User-Generated Content Verification in Journalism: An Overview},
author = {Sohail Ahmed Khan and Ghazaal Sheikhi and Andreas L. Opdahl and Fazle Rabbi and Sergej Stoppel and Christoph Trattner and Duc-Tien Dang-Nguyen},
url = {https://mediafutures.no/e0ret1-visual_user-generated_content_verification_in_journalism_an_overview/},
year = {2023},
date = {2023-01-16},
urldate = {2023-01-16},
journal = {IEEE Access},
abstract = {Over the past few years, social media has become an indispensable part of the news generation and dissemination cycle on the global stage. These digital channels along with the easy-to-use editing tools have unfortunately created a medium for spreading mis-/disinformation containing visual content. Media practitioners and fact-checkers continue to struggle with scrutinising and debunking visual user-generated content (UGC) quickly and thoroughly as verification of visual content requires a high level of expertise and could be exceedingly complex amid the existing computational tools employed in newsrooms. The aim of this study is to present a forward-looking perspective on how visual UGC verification in journalism can be transformed by multimedia forensics research. We elaborate on a comprehensive overview of the five elements of the UGC verification and propose multimedia forensics as the sixth element. In addition, different types of visual content forgeries and detection approaches proposed by the computer science research community are explained. Finally, a mapping of the available verification tools media practitioners rely on is created along with their limitations and future research directions to gain the confidence of media professionals in using multimedia forensics tools in their day-to-day routine.},
keywords = {},
pubstate = {published},
tppubtype = {article}
}