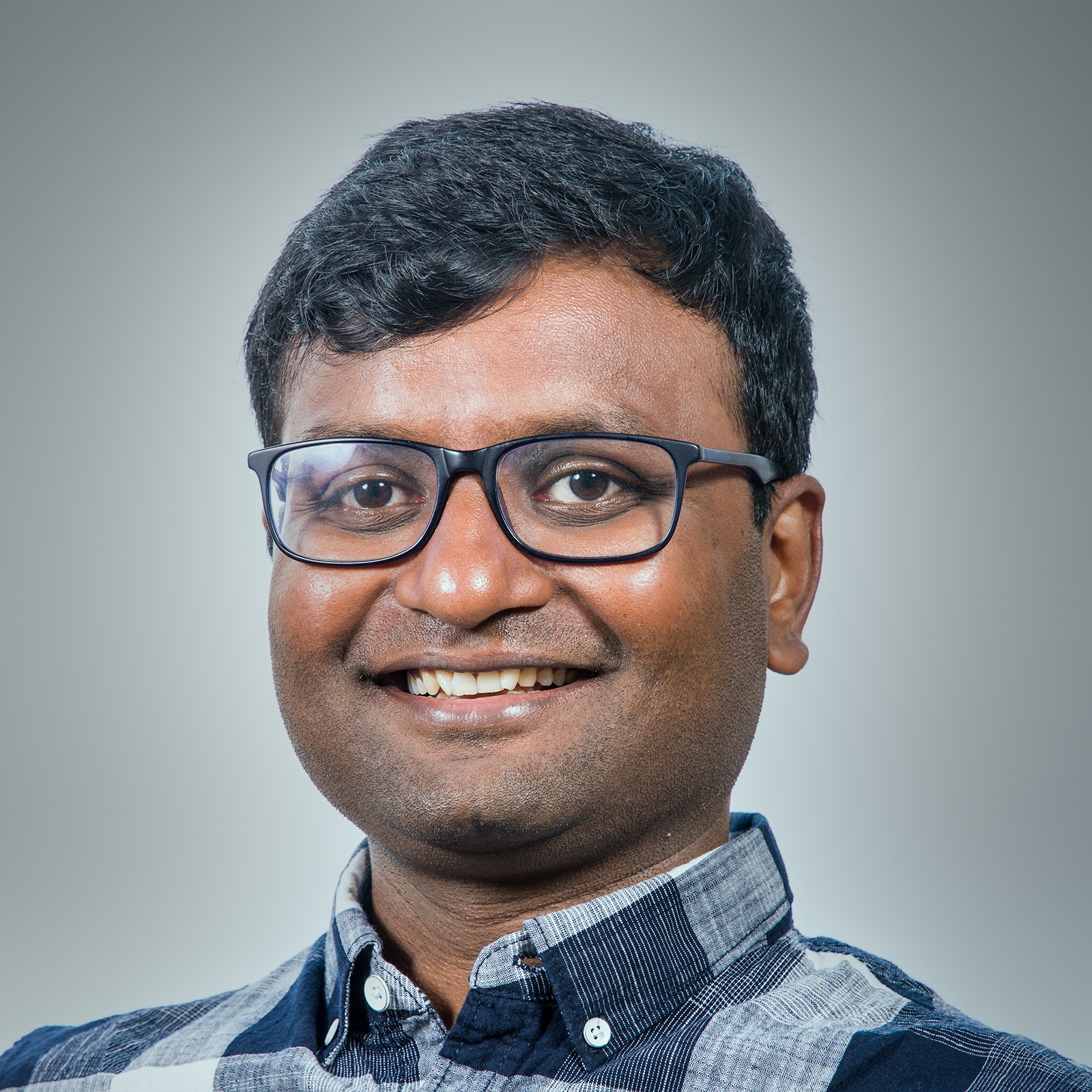
Assoc. Prof. Vinay Setty
Task Leader
2024
Aarnes, Peter Røysland; Setty, Vinay; Galuščáková, Petra
Conference and Labs of the Evaluation Forum, 2024.
@conference{checkthat24,
title = {IAI Group at CheckThat! 2024: Transformer Models and Data Augmentation for Checkworthy Claim Detection},
author = {Peter Røysland Aarnes and Vinay Setty and Petra Galuščáková},
url = {https://mediafutures.no/checkthat-lab-task-1-notebook/},
year = {2024},
date = {2024-09-13},
urldate = {2024-09-13},
booktitle = {Conference and Labs of the Evaluation Forum},
abstract = {This paper describes IAI group’s participation for automated check-worthiness estimation for claims, within
the framework of the 2024 CheckThat! Lab “Task 1: Check-Worthiness Estimation”. The task involves the
automated detection of check-worthy claims in English, Dutch, and Arabic political debates and Twitter data. We
utilized various pre-trained generative decoder and encoder transformer models, employing methods such as
few-shot chain-of-thought reasoning, fine-tuning, data augmentation, and transfer learning from one language
to another. Despite variable success in terms of performance, our models achieved notable placements on the
organizer’s leaderboard: ninth-best in English, third-best in Dutch, and the top placement in Arabic, utilizing
multilingual datasets for enhancing the generalizability of check-worthiness detection. Despite a significant drop
in performance on the unlabeled test dataset compared to the development test dataset, our findings contribute
to the ongoing efforts in claim detection research, highlighting the challenges and potential of language-specific
adaptations in claim verification systems.},
keywords = {},
pubstate = {published},
tppubtype = {conference}
}
the framework of the 2024 CheckThat! Lab “Task 1: Check-Worthiness Estimation”. The task involves the
automated detection of check-worthy claims in English, Dutch, and Arabic political debates and Twitter data. We
utilized various pre-trained generative decoder and encoder transformer models, employing methods such as
few-shot chain-of-thought reasoning, fine-tuning, data augmentation, and transfer learning from one language
to another. Despite variable success in terms of performance, our models achieved notable placements on the
organizer’s leaderboard: ninth-best in English, third-best in Dutch, and the top placement in Arabic, utilizing
multilingual datasets for enhancing the generalizability of check-worthiness detection. Despite a significant drop
in performance on the unlabeled test dataset compared to the development test dataset, our findings contribute
to the ongoing efforts in claim detection research, highlighting the challenges and potential of language-specific
adaptations in claim verification systems.
2023
Opdahl, Andreas L.; Tessem, Bjørnar; Dang-Nguyen, Duc-Tien; Motta, Enrico; Setty, Vinay; Throndsen, Eivind; Tverberg, Are; Trattner, Christoph
Trustworthy Journalism Through AI Journal Article
In: Data & Knowledge Engineering (DKE), Elsevier, 2023.
@article{Opdahl2023,
title = {Trustworthy Journalism Through AI},
author = {Andreas L. Opdahl and Bjørnar Tessem and Duc-Tien Dang-Nguyen and Enrico Motta and Vinay Setty and Eivind Throndsen and Are Tverberg and Christoph Trattner},
url = {https://mediafutures.no/1-s2-0-s0169023x23000423-main/},
year = {2023},
date = {2023-04-29},
urldate = {2023-04-29},
journal = {Data & Knowledge Engineering (DKE), Elsevier},
abstract = {Quality journalism has become more important than ever due to the need for quality and trustworthy media outlets that can provide accurate information to the public and help to address and counterbalance the wide and rapid spread of disinformation. At the same time, quality journalism is under pressure due to loss of revenue and competition from alternative information providers. This vision paper discusses how recent advances in Artificial Intelligence (AI), and in Machine Learning (ML) in particular, can be harnessed to support efficient production of high-quality journalism. From a news consumer perspective, the key parameter here concerns the degree of trust that is engendered by quality news production. For this reason, the paper will discuss how AI techniques can be applied to all aspects of news, at all stages of its production cycle, to increase trust.},
keywords = {},
pubstate = {published},
tppubtype = {article}
}
2020
Setty, Vinay; Rekve, Erlend
Truth be told: Fake news detection using user reactions on reddit Journal Article
In: Proceedings of the 29th acm international conference on information knowledge management, pp. 3325–3328, 2020, (Pre SFI).
@article{Setty2020,
title = {Truth be told: Fake news detection using user reactions on reddit},
author = {Vinay Setty and Erlend Rekve},
url = {https://dl.acm.org/doi/pdf/10.1145/3340531.3417463},
doi = {https://doi.org/10.1145/3340531.3417463},
year = {2020},
date = {2020-10-01},
journal = {Proceedings of the 29th acm international conference on information knowledge management},
pages = {3325–3328},
abstract = {In this paper, we provide a large dataset for fake news detection using social media comments. The dataset consists of 12,597 claims (of which 63% are labelled as fake) from four different sources (Snopes, Poltifact, Emergent and Twitter). The novel part of the dataset is that it also includes over 662K social media discussion comments related to these claims from Reddit. We make this dataset public for the research community. In addition, for the task of fake news detection using social media comments, we provide a simple but strong baseline solution deep neural network model which beats several solutions in the literature.},
note = {Pre SFI},
keywords = {},
pubstate = {published},
tppubtype = {article}
}
Botnevik, Bjarte; Sakariassen, Eirik; Setty, Vinay
Brenda: Browser extension for fake news detection Journal Article
In: Proceedings of the 43rd international acm sigir conference on research and development in information retrieval, pp. 2117–2120, 2020, (Pre SFI).
@article{Botnevik2020,
title = {Brenda: Browser extension for fake news detection},
author = {Bjarte Botnevik and Eirik Sakariassen and Vinay Setty},
url = {https://arxiv.org/pdf/2005.13270.pdf},
doi = {10.1145/3397271.3401396},
year = {2020},
date = {2020-05-27},
journal = {Proceedings of the 43rd international acm sigir conference on research and development in information retrieval},
pages = { 2117–2120},
publisher = {Association for Computing Machinery},
abstract = {Misinformation such as fake news has drawn a lot of attention in recent years. It has serious consequences on society, politics and economy. This has lead to a rise of manually fact-checking websites such as Snopes and Politifact. However, the scale of misinformation limits their ability for verification. In this demonstration, we propose BRENDA a browser extension which can be used to automate the entire process of credibility assessments of false claims. Behind the scenes BRENDA uses a tested deep neural network architecture to automatically identify fact check worthy claims and classifies as well as presents the result along with evidence to the user. Since BRENDA is a browser extension, it facilities fast automated fact checking for the end user without having to leave the Webpage.},
note = {Pre SFI},
keywords = {},
pubstate = {published},
tppubtype = {article}
}
2019
Mishra, Rahul; Setty, Vinay
Hierarchical attention networks to learn latent aspect embeddings for fake news detection Conference
Proceedings of the 2019 acm sigir international conference on theory of information retrieval, Association for Computing Machinery, New York, 2019, (Pre SFI).
@conference{Mishra2019,
title = {Hierarchical attention networks to learn latent aspect embeddings for fake news detection},
author = {Rahul Mishra and Vinay Setty},
url = {https://dl.acm.org/doi/pdf/10.1145/3341981.3344229},
doi = {10.1145/3341981.3344229},
year = {2019},
date = {2019-09-01},
booktitle = {Proceedings of the 2019 acm sigir international conference on theory of information retrieval},
pages = {197–204},
publisher = {Association for Computing Machinery},
address = {New York},
abstract = {Recently false claims and misinformation have become rampant in the web, affecting election outcomes, societies and economies. Consequently, fact checking websites such as snopes.com and politifact.com are becoming popular. However, these websites require expert analysis which is slow and not scalable. Many recent works try to solve these challenges using machine learning models trained on a variety of features and a rich lexicon or more recently, deep neural networks to avoid feature engineering. In this paper, we propose hierarchical deep attention networks to learn embeddings for various latent aspects of news. Contrary to existing solutions which only apply word-level self-attention, our model jointly learns the latent aspect embeddings for classifying false claims by applying hierarchical attention. Using several manually annotated high quality datasets such as Politifact, Snopes and Fever we show that these learned aspect embeddings are strong predictors of false claims. We show that latent aspect embeddings learned from attention mechanisms improve the accuracy of false claim detection by up to 13.5% in terms of Macro F1 compared to a state-of-the-art attention mechanism guided by claim-text DeClarE. We also extract and visualize the evidence from the external articles which supports or disproves the claims},
note = {Pre SFI},
keywords = {},
pubstate = {published},
tppubtype = {conference}
}
2018
Setty, Vinay; Hose, Katja
Neural embeddings for news events Conference
The 41st international acm sigir conference on research development in information retrieval, Association for Computing Machinery Association for Computing Machinery, New York, 2018, (Pre SFI).
@conference{Setty2018,
title = {Neural embeddings for news events},
author = {Vinay Setty and Katja Hose},
url = {https://dl.acm.org/doi/pdf/10.1145/3209978.3210136},
doi = {10.1145/3209978.3210136},
year = {2018},
date = {2018-06-01},
booktitle = {The 41st international acm sigir conference on research development in information retrieval},
pages = {1013–1016},
publisher = {Association for Computing Machinery},
address = {New York},
organization = {Association for Computing Machinery},
abstract = {Representation of news events as latent feature vectors is essential for several tasks, such as news recommendation, news event linking, etc. However, representations proposed in the past fail to capture the complex network structure of news events. In this paper we propose Event2Vec, a novel way to learn latent feature vectors for news events using a network. We use recently proposed network embedding techniques, which are proven to be very effective for various prediction tasks in networks. As events involve different classes of nodes, such as named entities, temporal information, etc, general purpose network embeddings are agnostic to event semantics. To address this problem, we propose biased random walks that are tailored to capture the neighborhoods of news events in event networks. We then show that these learned embeddings are effective for news event recommendation and news event linking tasks using strong baselines, such as vanilla Node2Vec, and other state-of-the-art graph-based event ranking techniques.},
note = {Pre SFI},
keywords = {},
pubstate = {published},
tppubtype = {conference}
}
2017
Setty, Vinay; Anand, Abhijit; Mishra, Arunav; Anand, Avishek
Modeling event importance for ranking daily news events Conference
Proceedings of the tenth acm international conference on web search and data mining, Association for Computing Machinery New York, 2017, (Pre SFI).
@conference{Setty2017,
title = {Modeling event importance for ranking daily news events},
author = {Vinay Setty and Abhijit Anand and Arunav Mishra and Avishek Anand},
url = {https://dl.acm.org/doi/pdf/10.1145/3018661.3018728},
doi = {10.1145/3018661.3018728},
year = {2017},
date = {2017-02-01},
booktitle = {Proceedings of the tenth acm international conference on web search and data mining},
pages = {231–240},
address = {New York},
organization = {Association for Computing Machinery},
abstract = {We deal with the problem of ranking news events on a daily basis for large news corpora, an essential building block for news aggregation. News ranking has been addressed in the literature before but with individual news articles as the unit of ranking. However, estimating event importance accurately requires models to quantify current day event importance as well as its significance in the historical context. Consequently, in this paper we show that a cluster of news articles representing an event is a better unit of ranking as it provides an improved estimation of popularity, source diversity and authority cues. In addition, events facilitate quantifying their historical significance by linking them with long-running topics and recent chain of events. Our main contribution in this paper is to provide effective models for improved news event ranking.
To this end, we propose novel event mining and feature generation approaches for improving estimates of event importance. Finally, we conduct extensive evaluation of our approaches on two large real-world news corpora each of which span for more than a year with a large volume of up to tens of thousands of daily news articles. Our evaluations are large-scale and based on a clean human curated ground-truth from Wikipedia Current Events Portal. Experimental comparison with a state-of-the-art news ranking technique based on language models demonstrates the effectiveness of our approach.},
note = {Pre SFI},
keywords = {},
pubstate = {published},
tppubtype = {conference}
}
To this end, we propose novel event mining and feature generation approaches for improving estimates of event importance. Finally, we conduct extensive evaluation of our approaches on two large real-world news corpora each of which span for more than a year with a large volume of up to tens of thousands of daily news articles. Our evaluations are large-scale and based on a clean human curated ground-truth from Wikipedia Current Events Portal. Experimental comparison with a state-of-the-art news ranking technique based on language models demonstrates the effectiveness of our approach.